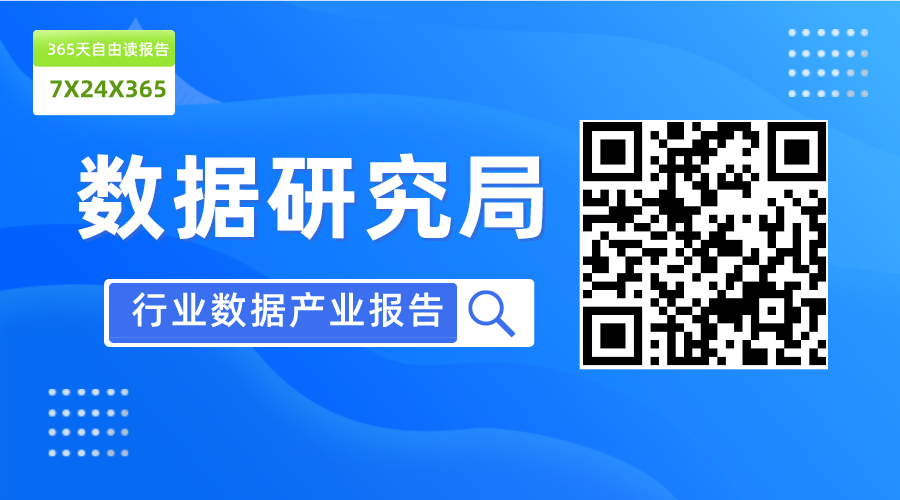
The Unfolding of Women’s Economic Empowerment Outcomes: Time Path of Impacts in an Indonesia TrialMayra Buvinic, James C. Knowles and Firman WitoelarAbstractThis paper makes the case that some interventions designed to improve women’s economic lives need to be tracked long enough for women to manifest new and beneficial behaviors. The study analyzes the time paths of the estimated impacts in a randomized trial providing financial incentives to bank agents (on the supply-side) and basic business training to women business owners (on the demand-side) for them to access and use formal financial services. The trial took place over a twoyear period in 401 villages in five regencies of East Java province, Indonesia. Although estimated impacts of the treatments are positive and significant for seven of eight economic empowerment outcomes, the impacts of most outcomes do not adhere strictly to a linear time trend. For example, strong positive impacts on women’s profits and household welfare do not become significant until the second year after their first exposure to the treatments, whereas the impact on women’s agency in household decision-making is significant only during the first six months since first exposure. In contrast, the timing of some impacts (business practices and savings) does not vary significantly over time and can therefore be measured correctly with the typical constant impact model. The paper’s findings are consistent with other incipient evidence on the delayed effects of some interventions targeting women’s economic empowerment. They underline the need to measure impacts over a sufficiently long period, which in this case is at least two years, and suggest that this may be especially important to do when interventions seek to fundamentally alter women’s traditional household roles and their agency.Keywords: Women’s economic empowerment, financial inclusion, entrepreneurship, and agencyJEL: J16, B54, and L26, and D91www.cgdev.orgWorking Paper 586 June 2021The Unfolding of Women’s Economic Empowerment Outcomes: Time Path of Impacts in an Indonesia TrialMayra Buvinic Center for Global DevelopmentJames C. KnowlesFirman Witoelar Crawford School of Public Policy, Australian National UniversityThis research was supported by generous grants from the ExxonMobil Foundation to the Center for Global Development and from the Umbrella Facility for Gender Equality (UFGE) in partnership with the Australian Department of Foreign Affairs and Trade to the World Bank Group’s East Asia and Pacific Gender Innovation Lab. Special thanks go to James M. Jones for his steadfast support of this work, to Mead Over for his many insightful comments, and to two anonymous readers for their careful reviews. We are also grateful to Erika Deserrano, Hillary C. Johnson, Gianmarco León-Ciliotta, Elizaveta Perova and our partners J-PAL SEA, Mercy Corps Indonesia, and Survey Meter for their substantive contributions to the research trial on which this paper is based. We are especially grateful to Glory Sunarto, Andrea Adhi, Marifatual Amalia, Jenna Juwono, Lolita Moorena, Michael Tjahjadi, and Irwan Setyawan for their excellent field work.The Center for Global Development is grateful for contributions from the ExxonMobil Foundation in support of this work.Mayra Buvinic, James C. Knowles, and Firman Witoelar, 2021. “The Unfolding of Women’s Economic Empowerment Outcomes: Time Path of Impacts in an Indonesia Trial.” CGD Working Paper 586. Washington, DC: Center for Global Development. https://www.cgdev.org/publication/unfolding-womens-economic-empowermentoutcomes-time-path-impacts-indonesia-trial..Center for Global Development 2055 L Street NWWashington, DC 20036202.416.4000 (f) 202.416.4050The Center for Global Development works to reduce global poverty and improve lives through innovative economic research that drives better policy and practice by the world’s top decision makers. Use and dissemination of this Working Paper is encouraged; however, reproduced copies may not be used for commercial purposes. Further usage is permitted under the terms of the Creative Commons License.www.cgdev.orgThe views expressed in CGD Working Papers are those of the authors and should not be attributed to the board of directors, funders of the Center for Global Development, or the authors’ respective organizations.Contents1. Introduction.................................................................................................................................. 1 2. Background, description of the trial, and review of the literature........................................ 32.1 Background ............................................................................................................................ 3 2.2 Description of the trial ......................................................................................................... 3 3. Data................................................................................................................................................ 5 3.1 Description of the data......................................................................................................... 5 3.2 Sample attrition ..................................................................................................................... 6 4. Model specification...................................................................................................................... 7 4.1 Time elapsed after treatment exposure.............................................................................. 7 4.2 Impact measurement models .............................................................................................. 8 5. Estimation ................................................................................................................................... 10 5.1 Transformations of outcomes prior to estimation ........................................................ 10 5.2 Model estimation.................................................................................................................11 6. Results..........................................................................................................................................12 7. Conclusions.................................................................................................................................16 References ....................................................................................................................................... 18 Figures and tables...........................................................................................................................20 Annex 1. Description of the outcomes analyzed ...................................................................... 33 Annex 2. Re-transformation of estimates to their original metrics ........................................ 351. IntroductionThis paper is based on a randomized controlled trial in rural Indonesia designed to evaluate alternative approaches to increasing women business owners’ (WBO) access to and utilization of formal financial services. The trial measured the impact of both supply-side (agent incentives) and demand-side (basic business training and mentoring) treatments on WBO profits, capital and labor inputs, business practices, savings, agency and household welfare. In this paper we are particularly interested in the time paths of the estimated impacts, e.g., when they materialized and whether they increased or decreased or remained unchanged over time. This is important for several reasons. First, it provides information about whether the estimated impacts can be expected to continue, diminish or increase in the future, which is relevant to the economic evaluation of the trial and may be required for impacts that take time to materialize. Second, knowledge of the time paths of impacts should be helpful in deciding when to measure impacts in future trials (King and Behrman 2009). Third, the sequence of impacts (i.e., which ones occurred at the same time as, prior to or after other impacts) should help to understand possible causal linkages between outcomes. For example, did improvements in business practices precede increases in profits? Did increases in savings precede increases in capital inputs?The analysis in this paper exploits the unique features of the panel data collected during the trial while addressing some of its limitations. The household survey data include two phases of baseline data on 2,853 WBOs in 401 villages spanning a 14-month period (November 2016–December 2017) and two follow-up surveys (the first in February 2018 and the second in November/December 2018). Actual implementation of the treatments occurred over a 15-month period (December 2016–June 2018). As a result, there is considerable variation in the data in the time since WBOs were first exposed to the treatments. Table 1 shows that with the combined data from both follow-up surveys, there are multiple observations in four six-month post-exposure intervals, although there are relatively few observations for the last six-month interval of 19–24 months.The analysis addresses endogeneity in the time since first exposure to the treatments in both follow-up survey rounds by obtaining village-level fixed-effects (FE) estimates that are limited to variation within village-level panels, effectively removing the effects of any unobserved village-level fixed effects that may have been present during the period of the data collection. Possible sample selection bias in the follow-up surveys is addressed by assessing the robustness of the results with respect to alternative adjustments for sample selection bias.The results show that the time path of estimated impacts from exposure to the training and mentoring of WBOs alone or combined with high agent incentives varies for different economic empowerment outcomes. Women’s increased agency is short-lived, while increases in profits, business capital and household welfare emerge with a significant delay (after 12 months). Improved business practices and increased savings materialize in the short-term.The training and mentoring have strong significant positive effects on WBO’s agency with respect to household decision-making in the first six months post-treatment. While shortlived, increased agency may have contributed, alongside the treatments, to increasing WBO’s profits and business capital. Predicted effects on profits of the time elapsed after exposure to1treatments are positive starting in the third six-month interval (13–18 months posttreatment) and increase non-linearly over time. Similarly, the predicted effect on capital inputs, after adjusting for sample attrition, also increases after a significant delay (after 12 months) but much less so than profits.WBO’s labor inputs (hours worked in a typical month in the primary business) are not significantly affected by the treatments over time, whereas the proportion of recommended business practices followed are significantly higher in the second and third six-month intervals (no data are available for the first six-month interval) and compatible with the notion that effects do not vary with the time elapsed since exposure.Predicted effects on WBO’s savings (non-zero savings and amount saved) are positive and significant in the second and third six-month intervals and seem to increase as the time after their first exposure to the treatments lengthens, albeit at a much lesser degree than for profits. Savings may have contributed to increased business capital and/or increased household welfare. The predicted effects of exposure to the treatments on household welfare (as measured by a household-level asset index) are uniformly positive and highly significant in the third and fourth six-month intervals and they increase non-linearly over time, consistent with the notion that the increase in household assets was financed by the increase in WBO’s profits.These results complement an incipient literature on the path of treatment effects on women’s economic empowerment outcomes showing both short-lived and delayed effects. Banerjee, Karlan and Zinman (2015) found -- like our findings here -- that an increase in women’s empowerment (measured through reported influence on household decision making) as a result of the Graduation programs for the very poor in six countries, picked up in most programs, dissipated within the year. These Graduation programs included a capital transfer and complementary training and, in four of the six countries, targeted women within poor households. Buvinic and O’Donnell (2019) reviewing the literature suggest that these short-lived empowerment effects could be because women’s increased income and control over it is not sizeable enough to overcome traditional social norms, which take effort and time to change, or because empowerment effects are domain or context-specific, or because empowerment effects are hard to measure reliably through self-reporting.Regarding delayed effects, recent business training trials targeting micro-entrepreneurs have shown positive effects of business training on profits and/or sales and have included two or more follow-up surveys so that they are able to observe how impacts vary over time posttreatment: Studies in Peru and in South Africa showed increases in WBO’s business profits with a significant delay: in Peru, women business owners who only received training caught up in terms of increased sales with those who also received technical assistance but only in the second year (Valdivia 2015). In South Africa, an intensive training course to business owners (45% of them female), increased profits significantly, but only after a year – there were no significant increases in profits in the first six months (Anderson-MacDonald and others 2018).Similarly, in both Kenya and Vietnam, an ILO training and mentoring program for WBOs showed positive results with a delay – in Kenya increased profits and sales were significant only three years after training (McKenzie and Puerto 2017). In Vietnam, women’s profits and personal empowerment (internal control and intra-household decision making) materialized a year after the training (Huis, Lensink, Vu and Hansen 2019).2Deviating from these delayed results on profits, in rural Mexico intensive training provided to WBOs showed short-term positive effects that were maintained 2.5 years after implementation (Calderon, Cunha and De Giorgi 2013). Similarly, in Sri Lanka, a combination of business training and cash grants led to large and significant increases in WBO’s profits during the first eight months post-treatment; these effects, however, largely disappeared in the second year (De Mel, McKenzie and Woodruff 2014).It makes intuitive sense that increases in the profits of WBO’s firms, which on average are considerably smaller and less capital intensive and profitable than male-owned firms, will take time to materialize, especially after relatively ‘light’ interventions, such as most of the ones in the studies cited above as well as the ones in this trial. More intensive interventions (Sri Lanka above) and/or targeting WBOs with larger firms (Mexico above) may produce results more quickly.2. Background, description of the trial, and review of the literature2.1 BackgroundTo promote full financial inclusion, the Government of Indonesia adopted a law in 2014 that authorized the establishment of banking services in the absence of branch offices, called “branchless banking.”1 The Indonesian model of branchless banking uses village-based agents and mobile telephone communications (including both SMS and internet access) to offer basic banking services, including interest-bearing savings accounts with no opening or maintenance fees. The agents are mostly existing shop-owners who are authorized to assist customers to open accounts, accept cash deposits and enable cash withdrawals from customers’ savings accounts. Only the account holders and their banks have access to account balances, which makes them less vulnerable to demands from spouses, relatives and other social claimants.Although targeted more generally to its large, mainly rural unbanked population, Indonesia’s branchless banking services provide a unique opportunity to improve rural women’s access to formal savings accounts and other formal financial services. Among underbanked rural women, WBOs are a potentially important target group for branchless banking services both because they are substantially under-banked relative to men business owners, and possibly partly as a consequence, have lower savings, fewer business assets and lower business incomes (World Bank 2016, Buvinic, Knowles and Witolear 2018).22.2 Description of the trialThe trial supported both supply-side and demand-side interventions designed to increase the utilization of saving and other branchless banking services by WBOs in 401 predominantly1 OJK Regulation No. 19/POJK.03/2014 dated 18 November 2014 (“Laku Pandai Regulation”). 2 Sample WBOs earn about half as much as men business owners from their primary and secondary businesses and have only about a third of the business assets. WBOs are about half as likely as men business owners to have borrowed money during the past 12 months and their total savings during the last 12 months are about half as large in value, although 84% of WBOs report having saved during the last 12 months, compared to only 69% of men business owners.3rural villages of five regencies (kabupaten) of East Java province, Indonesia in which the study’s partner bank planned to establish branchless banking services.3 The interventions were randomized within an experimental design that makes it possible to assess the effectiveness and cost effectiveness of the supply-side and demand-side interventions, both separately and in combination. In each sample village, the partner bank (with assistance from the trial) recruited a branchless banking agent using the bank’s standard selection criteria (the selected agents were in many cases clients with a good credit history).4Sample villages were randomly assigned to high or low incentives, including one agent in each village (48.3% of whom were women). The random assignment of villages was stratified by village randomization group. One hundred and ninety-six villages/agents (48.9%) were randomly assigned to the high-incentive treatment, while 205 villages/agents (51.1%) were randomly assigned to the low-incentive treatment.The standard agent fee for identifying a new savings account client that deposits at least Rp. 20,000 (about US$1.50) and who maintains an average savings balance of Rp. 20,000 over two weeks is Rp. 2,000 (about US$0.14). This is the “low-incentive treatment” in the trial. The randomly assigned “high-incentive treatment” is Rp. 10,000 (about US$0.71) for each new client enrolled.5The random assignment of WBOs to the training and mentoring treatment was also stratified by village, resulting in 1,604 WBOs (56.2%) randomly assigned to the training and mentoring treatment and the remaining 1,248 WBOs (43.8%) assigned to the control group. 6 Random assignment of the WBO training and mentoring treatment within villages raises the possibility of spillover effects of the training and mentoring from WBOs randomly assigned to receive the treatment to WBOs (primarily in the same village) assigned to the control group. However, the data do not point to the presence of significant spillover effects. 7All agents and randomly selected WBOs were trained and mentored by Mercy Corps Indonesia (MCI), an organization with extensive experience providing financial and business literacy training to Indonesian business owners and farmers. Both the agent and WBO training and mentoring were conducted in two batches, the first batch during the3 The five sample regencies are: Tuban, Bojonegoro, Ngawi, Lamongan and Gresik, with a combined 2010 population of 5.56 million. 354 of the sample villages (88.3%) are rural, while 47 (11.7%) are semi-urban. 4 The standard criteria are: (1) the owner is a previous borrower from the bank, (2) the business is in a central location in the village, (3) the owner is mostly present at the business premises, (4) the owner has a good reputation among villagers (as reported by the village chief), (5) the owner is able to demonstrate sufficient financial liquidity, (6) the owner is not an agent for another bank, and (6) the owner is willing to participate as an agent. 5 The trial also included a village-level informational treatment (orthogonal to the main agent incentive treatment) that varied information about the level of incentives agents received in randomly selected villages. See Deserrano, E., G. Leon-Ciliotta and F. Witoelar (2021). 6 The sample WBOs were randomly selected from village lists of WBOs who were between 18 and 55 years of age, had a currently operating business in a sector other than agriculture, resided in the sample village, and had an operational mobile phone. 7For example, 39.3% and 43.2% of the WBOs randomly assigned to receive the training and mentoring treatment reported that they “knew about” the partner bank’s two e-savings products, compared to only 4.9% and 3.9% respectively of WBOs who were not assigned to receive the training and mentoring. By comparison, only 6.3% and 5.8% of the two groups of WBOs respectively “knew about” the most frequently known e-savings product of another bank. Only 9 and 6 respectively of the 58 and 46 WBOs not randomly assigned to receive the training and mentoring who knew about the partner bank’s two e-savings products, reported that they learned about the products from “friends or relatives.”4period December 2016 to July 2017 and the second batch during the period August 2017 to June 2018.The randomly assigned WBO training and mentoring focused on financial and business literacy (tracking income and expenses, setting priorities, the importance of saving, financial planning, basic bookkeeping, cash flow planning, record-keeping) and information on the partner bank’s branchless banking products. Apart from marketing (not included) and the focus on saving and use of the branchless banking products (included), the topics covered are typical of those covered in business training trials (McKenzie and Woodruff 2014). The initial training was conducted in village groups and lasted about 3 hours (but ranging from 1 to 4 hours) and was followed up by three group mentoring sessions of similar length that focused on addressing any questions from the trainees and on actual practices using their own individual businesses as cases. Program data indicate that the percentages of WBOs randomly assigned to the training and mentoring treatment (N=1,604) who participated in the initial training and each of the three successive mentoring sessions are 94.3%, 84.4%, 83.5% and 82.7% respectively, indicating a relatively high level of compliance. The agent training was provided to all agents in one personal (one-on-one) session that averaged about 3 hours in length and three subsequent one-on-one mentoring visits. The agent training included a module on marketing that emphasized the potential value of marketing to underbanked groups, particularly women.An endline impact evaluation of the trial found that the interventions were successful in increasing WBO’s profits, capital inputs, adherence to recommended business practices, agency in household decision-making, savings and household ownership of durable assets, and reduced use of money lenders (Buvinic and others 2020). However, it found very little effect of the treatments on the take-up and utilization of the partner bank’s branchless banking services.3. Data3.1 Description of the dataA Baseline Survey (BS) was conducted in 401 villages in two phases (November 2016– February 2017 in 107 villages; July-November 2017 in the remaining 294 villages). The BS collected extensive data on both agents and business owners, including 2,852 WBOs. The business owner data were collected in a single household questionnaire that required about 1.5 hours to administer.The first follow-up survey (FS1) of 1,399 WBOs interviewed in the BS was conducted in February 2018 in a non-random sample of 200 villages in which the training and mentoring of agents and WBOs had been completed prior to the survey. The second follow-up survey (FS2) of 2,841 WBOs interviewed in the BS was conducted in all 401 sample villages in November-December 2018.The analysis in this paper focuses on the following WBO outcomes: profits, capital and labor inputs in WBOs’ businesses, self-reported adherence to recommended business practices, individual savings (any savings and the level of non-zero savings), WBOs’ participation in multiple household decisions (agency) and household welfare (based on an asset index). Annex 1 provides detailed descriptions of these eight outcomes, while Table 45reports selected statistics by survey round on the eight outcomes. Three of the outcomes (profits, capital inputs and non-zero savings) might not be expected to respond as quickly to the treatments as the other outcomes due to the way they are measured. For example, WBOs’ profits are measured as “average monthly profits during the past 12 months,” WBOs savings are measured as “savings during the past 12 months” (or during the past 3 months, in FS1). Capital inputs are measured as the current value of the business capital stock, which one might expect to be less sensitive to the treatments than to annual investment flows (data on which were collected only in FS2). In addition, all three of these outcomes are highly skewed (positively).83.2 Sample attritionBoth follow-up surveys experienced relatively low rates of overall sample attrition (i.e., 3.9% in FS1 and 4.1% in FS2) when defined as the inability to collect follow-up data from WBOs who were interviewed in the baseline survey. In most cases, sample attrition was due to refusal to respond rather than to inability to be contacted. However, attrition (non-reporting) rates are higher for some of the outcomes studied (e.g., 7.0% in profits, and 12.7% in capital inputs).9 Possible attrition bias in WBO outcomes was assessed by estimating the following linear regression model with data from the two follow-up survey rounds (r=FS1, FS2):Ai,j,r = β1,0 + β1,1Highj + β1,2Traini,j + xi,jδ1 + ε1,i,j,r (r=FS1, FS2)(1)where Ai,j,r indicates that an outcome for WBO i in village j is not reported in follow-up survey round r, Highj is a dummy variable indicating that the agent in village j was randomly assigned to receive high incentives, Traini,j is a dummy variable indicating that WBO i in village j was randomly assigned to receive training and mentoring, xi,j is a row vector of baseline covariates,10 β1,0, β1,1, β1,2 and the vector δ1 are fixed coefficients, and ε1i,j,r is a random disturbance clustered at the village level. Equation (1) was estimated both by OLS and by fixed-effects estimation at the village level.11 The results in Table 5 (columns 1 and 4) indicate that overall sample attrition is not significantly related to either one of the randomized treatments (or to both jointly). To check whether the observed and unobserved characteristics of attriters differ across the treatment arms (Dumville and others 2006, Bell and others 2013, Winston and others 2016, Millan and Macours 2017) the following equation was estimated for each treatment arm separately for the three attrition measures:Ai,j,r = β2,0 + xi,jδ2 + ε2,i,j,r(r=FS1, FS2)(2)where the variables are defined as in equation (1). The results in Tables A-1 to A-3 in the online appendix indicate that the joint hypothesis that the coefficients of the covariates (i.e., the predictors of attrition) are the same across the four treatment arms (i.e., control, high agent incentives only, WBO training and mentoring only, and both treatments combined) is not rejected at conventional significance levels with either estimation method. However, several of the estimated coefficients of individual covariates vary significantly across8 The skewness statistics of the profits, capital inputs and non-zero savings outcomes are 60.36, 12.74 and 8.97 respectively in the pooled data for these three outcomes, compared to zero in a symmetric distribution, 0.64 for the household welfare measure and 1.32 for labor inputs. 9 These overall non-reporting rates are based on both follow-up survey rounds, as reported in Table 5. 10 The covariates (xi,j) include all of the variables analyzed in Table B-1 in the online appendix. 11 Village-level fixed-effects estimates are obtained because the FS1 sample is a non-random sample of the villages, as discussed in section 5.2.6treatment arms. Accordingly, for the two outcomes of profits and capital inputs, with significantly higher attrition, the models are also estimated using either inverse probability weights or Heckman’s sample selection model (Wooldridge 2010).12 The estimates are presented in section D of the online appendix and their implications are discussed in the results section.An additional sample selection problem arises from the non-random sampling of the villages included in the first follow-up survey (FS1). However, because the FS1 sample is selective only at the village level, use of village-level fixed effects estimation should remove any sample selection bias (as the results in columns 1 and 4 of Table 5 suggest). As an additional robustness check, however, the models are re-estimated with the FS1 data excluded. The results are presented in section E of the online appendix and are quite similar to the results reported in Tables 6–8 with the FS1 data included.4. Model specification4.1 Time elapsed after treatment exposureIn this paper, we are interested in how the effects of both the supply-side and demand-side treatments may vary with the time elapsed since WBOs were first exposed to the randomly assigned treatments. In the case of the supply-side treatment, the time elapsed since the WBOs’ first exposure to the randomly assigned treatment of high or low agent incentives (DA) is assumed to have begun in the month of the agents’ initial deployment (MA) and to have continued up to the month in which the WBO was re-interviewed in a follow-up survey (M):DAi,r = Mi,r – MAj(r=FS1, FS2)(3)where i refers to the WBO, j refers to village, and r refers to the survey round. DA is assumed to be zero for all WBOs in both phases of the baseline survey (r=BSa, BSb), and DA is also assumed to be zero for WBOs in untreated (low incentive) villages in both follow-up survey rounds (r=FS1, FS2).13In the case of the demand-side treatment, the time elapsed since the WBOs’ first exposure to the randomly assigned treatment of training and mentoring (DW) is assumed to have begun in the month in which the WBO’s training and mentoring began (MWi) and to have continued up to the month in which the WBO was re-interviewed in a follow-up survey (M):DWi,r = Mi,r – MWi(r=FS1, FS2)(4)where i, j and r are defined as above. DW is assumed to be zero for all WBOs in both phases of the baseline survey (r=BSa, BSb), and DW is also assumed to be zero for WBOs not12 The selection equation in Heckman’s model is identified by the randomized treatments, which are significantly related to attrition in this case, as indicated by the results in Table 5. 13 DAi,r is also assumed to be zero in all survey rounds in villages in which the agent resigned or was discharged prior to receiving initial training and was not replaced (10 villages). However, if the resigned agent received initial training (11 villages), DAi,r is assumed to equal the difference between the month of resignation and the month of initial training. In cases where an initial agent resigned and was subsequently replaced (18 villages), MAj is assumed to be the month in which the replacement agents received their initial training.7randomly assigned to receive the WBO training and mentoring treatment in both follow-up survey rounds (r=FS1, FS2).WBOs’ training month (MWi) varied within 24 of the 401 sample villages. Because this variation may not be random (King and Behrman 2009), the training month of all WBOs in a given village is assumed to be the earliest initial training month reported for a WBO in the same village (MWi=min(MWi)j, j= 1, …, 401).Some WBOs were exposed to both the supply and demand-side treatments. The time elapsed since first exposure to both treatments combined (DAWi,r) is assumed to have begun in the month in which the WBO was first exposed to both treatments (MAWi) and to have continued up to the month in which the WBO was re-interviewed in a follow-up survey (M):DAWi,r = Mi,r – MAWi(r=FS1, FS2)(5)where MAWi is equal to max(MAj, MWi).4.2 Impact measurement modelsImpact estimates are obtained using three alternative models. Model 1 estimates the impacts in successive six-month intervals since the time of first exposure to the treatments (i.e., at 1– 6 months, 7–12 months, 13–18 months, and 19–24 months). The estimated impacts in Model 1 are unconstrained (free to vary) from one six-month interval to another. However, the precision of the estimates is sensitive to the number of observations in each six-month interval. Model 2 estimates the impacts as a linear function of the time since first exposure. Although the estimates may be positive, negative or zero, they are constrained to lie along a single line. The estimates in Model 2 are more precise (and therefore more likely to be significant) than those obtained with Model 1 because only one parameter is estimated for each treatment. Model 3 estimates the impacts as a quadratic function of the time since first exposure to the treatments. Although the estimates are also constrained by the quadratic functional form, the implied time path of impacts is considerably more flexible than in Model 2. However, the increased flexibility of Model 3 over Model 2 comes at the cost of decreased precision because two parameters must be estimated for each treatment.Model 1 (unconstrained time patterns of impacts) can be written as:Yi,j,r = α6 + iajβ6 + iwiγ6 + iawi,j,δ6 + sρ6 + zλ + ε6,i,j,r(6)where Y refers to an outcome, r refers to the survey round, j refers to the village, i refers to an individual WBO, s is a row vector of dummy variables indicating different survey rounds (r=1b, 2, 3),14 z is a row vector of covariates including the baseline value of the outcome but whose other components vary across outcomes, where α6, ρ6 and λ are fixed parameters, ε6,i,j,r is a random disturbance term equal to c6,j + c6,i + u6,i,j,r (where c6,j is an unobserved village-level fixed effect that is assumed to be constant over time, c6,i is an unobserved WBO-level effect that may or may not be constant over time, and u6,i,j,r is an idiosyncratic error) and where14 Because the survey round dummies are included in the models to adjust for time (as well as variations in the definition of outcomes across survey rounds in some cases, as discussed in Annex 1). A dummy is also specified for the second phase of the baseline survey, which was separated from the first phase by several months (the first phase of the baseline survey is the omitted category).8iajβ6 = β6,1A1j + β6,2A2j + β6,3A3j + β6,4A4j(6a)iwiγ6 = γ6,1W1i + γ6,2W2i + γ6,3W2i + γ6,4W2i(6b)iawi,j,δ6 = δ6,1AW1i,j + δ6,2AW2i,j + δ6,3AW3i,j + δ6,4AW4i,j(6c)The A1–A4, W1–W4 and AW1–AW4 are dummy variables indicating different six-month post-exposure intervals to the high agent incentives, WBO training and mentoring and combined treatments respectively, and β6,1-β6,4, γ6,1-γ6,4 and δ6,1-δ6,4 are fixed parameters.The covariates in z are WBO-level baseline characteristics, including the baseline value of Y. In addition to restricting the covariates to baseline values, additional criteria for including a given covariate is that it be reported in the entire baseline sample and be statistically significant at the 0.05 level when included as an explanatory variable in a linear regression model estimated with baseline data and with a given outcome Y as the dependent variable. Consequently, the covariates included in z vary across outcomes.15 The covariates are included to increase the precision of the estimates and to control at least partially for possible attrition bias.The dummy variables in the row vector s are included to reflect possible intercept shifts in equation (6) over time (i.e., between survey rounds) as well as changes in the definitions of some outcome measures between survey rounds, as discussed in Annex 1.ε6,i,j,r is assumed to be correlated with the dummy variables indicating alternative six-month post-treatment exposures to the treatment variables (i.e., A1–A4, W1–W4 and AW1–AW4), as discussed below. In addition, due to the presence of the fixed effect c6,j , ε6i,j,r is assumed to be serially correlated in the same village j, but is assumed to be independent across villages with a variance that may be non-constant (heteroscedastic).The following joint hypotheses are tested with Model 1: (1) agent incentives have no effect (β6,1=β6,2=β6,3=β6,4=0); (2) the WBO training and mentoring has no effect (γ6,1=γ6,2=γ6,3=γ6,4=0); (3) there is no interaction effect between the two treatments (δ6,4=δ6,4=δ6,4=δ6,4=0); and (4) there is no combined effect of exposure to both treatments (β6,1+β6,2+β6,3+β6,4+γ6,1+γ6,2+γ6,3+γ6,4+δ6,1+δ6,2+δ6,3+δ6,4=0).Model 2 (linear trends in the time since first exposure to the treatments) is written as:Yi,j,r = α7 + β7DAj + γ7DWi + δ7DAWi,j + sρ7 + zλ + ε7,i,j,r(7)where DA, DW DAW are the time (in months) since first exposure to the alternative treatments, α7, β7, γ7, and δ7 are fixed parameters, and where the other variables and parameters are defined as in equation (6).The following joint hypotheses are tested with Model 2: (1) agent incentives have no effect (β7=0); (2) the WBO training and mentoring has no effect (γ7=0); (3) there is no interaction effect between the two treatments (δ7=0); and (4) there is no combined effect of the treatment arm for WBOs exposed to both treatments (β7+γ7+δ7=0).15 Table B-1 in the online appendix reports the regressions used to select the covariates to include in the models for each outcome.9Model 3 (quadratic trends in the time since first exposure to the treatments) is written as:Yi,j,r = α8 + β8,1DAj + β8,2DA2j + γ8,1DWi + γ8,2DW2i,j + δ8,1DAWi,j + δ8,2DAW2i,j+ sρ8 + zλ + ε8,i,j,r(8)The following joint hypotheses are tested with Model 3: (1) agent incentives have no effect (β8,1= β8,2=0); (2) the WBO training and mentoring has no effect (γ8,1= γ8,2=0); (3) there is no interaction effect between the two treatments (δ8,1= δ8,2=0); and (4) there is no combined effect of the treatment arm for WBOs exposed to both treatments (β8,1+ β8,2+γ8,1+ γ8,2+δ8,1+δ8,2=0).Joint tests can also be used to identify which model is most appropriate for estimating impact for a given outcome. For example, in Model 1, joint tests for equality in the impacts of each treatment across six-month intervals (β6,1=β6,2=β6,3=β6,4), (γ6,1=γ6,2=γ6,3=γ6,4), and (δ6,1=δ6,2=δ6,3=δ6,4) provide a basis for comparing Model 1 to the typical impact estimation model in which impact is assumed not to vary with the time elapsed since treatment exposure. In Model 2, which assumes a linear trend in the time pattern of effects, a joint test of the hypothesis that impacts do not vary with the time elapsed since exposure can provide a basis for choosing between Model 1 and Model 2. Lastly, a joint test of the significance of the quadratic terms in Model 3 provides a basis for choosing between Model 2 and Model 3.5. Estimation5.1 Transformations of outcomes prior to estimationAverage monthly profits in the WBOs’ primary business and the total value of their business capital inputs are both highly skewed (skewness = 17.48 and 8.97 respectively) with distributions that are approximately lognormal with only 3 and 13 zero values respectively. Both outcomes are transformed to inverse hyperbolic sine (IHS) values after being re-scaled (multiplied by 1,000) so that their distributions more closely approximate lognormal distributions, making the estimates easier to interpret (Bellemarre and Wichman 2019).16 In this case, the estimated average treatment effect on both dependent variables can be converted to a percentage change relative to the baseline sample mean in Model 1 by using Kennedy’s approximation (equation A2–1 in Annex 2) or to the projected percentage changes in Models 2 and 3 using the formula in equation (A2–2) in Annex 2.Labor inputs (hours worked in a typical month by WBOs in their primary business) is a discrete count variable bounded between zero and 720 with an approximately normal distribution, apart from the truncated left tail (skewness=1.32). Business practices (the sample proportion of 13 recommended businesses practices followed) is a scaled discrete count variable between 0 and 1, while household decision-making (the sample proportion of five household decisions in which the WBO is reported to participate) is also a scaled discrete count variable between 0 and 1.17 Although it might be preferable to model all three of these outcomes as nonlinear Poisson regressions, particularly if they were to be used in16 The estimates obtained using these two IHS-transformed variables are not significantly affected by the rescaling (Knowles 2019). 17 The fact that these two outcomes are scaled count variables (i.e., the counts are divided by 13 and 5 respectively) affects only the scaling of the estimated coefficients, not their significance levels.10forecasting, analyzing them as linear regression models, as done in this paper, makes little difference to the estimated average treatment effects.18The two savings measures are based on two separate questions: (1) did you save at all during the last 12 months (or last 3 months, in the first follow-up survey)? and, if yes, (2) how much did you save in various savings instruments? The responses are analyzed as two separate outcomes, the first as a zero-one (binary) dependent variable in a linear probability model (“any savings”)19 and the second as the non-zero amount saved (“non-zero savings”), which is highly skewed (skewness=44.71) and approximately lognormally distributed and is modeled as a natural log transformed dependent variable in a linear regression model.20 The estimated average treatment effect on the logged non-zero value of the amount saved can be converted to a percentage change relative to the baseline sample mean in Model 1 by using Kennedy’s approximation (equation A2–1 in Annex 2) or to the projected percentage changes in Models 2 and 3 using the formula in equation (A2–2) in Annex 2.Household welfare is a composite variable (index) equal to the first principal component of a set of standardized indicators referring to durable asset ownership that ranges from -5.323 to 10.571. Since the component indicators are standardized, the distribution of the index is centered on zero and its distribution is similar to a normal distribution (skewness=0.64), albeit with an extended positive tail. Accordingly, the household welfare variable is not transformed.5.2 Model estimationThe time elapsed since WBOs first exposure to the treatments (DA, DW and DAW), unlike the treatments themselves, were not randomly assigned. In particular, the time since first exposure is shorter in villages in which the agent or WBO training and mentoring was conducted later (as proxied for by the baseline survey month) due to delays in recruiting agents (Table 2).In addition, the first follow-up survey (FS1) sample is limited to 200 of the 401 sample villages in which the training and mentoring of agents and WBOs had been completed. This is clearly a non-random sample of the 401 sample villages, as evidenced by differences in the mean time elapsed since exposure, which is substantially higher in the second follow-up survey in villages that were included in FS1 (Table 3).Fortunately, the time elapsed since exposure for the WBOs is endogenous and/or selective only at the village level because the random assignment of WBOs to the training intervention was done within villages, with the time elapsed since exposure based on the date on which the first WBO in each village began her training, and was preserved in all survey rounds (i.e., if a village was included in FS1, all 7 sample WBOs in the village were included in the survey sample).The random assignment of the WBO training and mentoring treatment within each village panel removes any correlation between the unobserved WBO-level fixed effect (ci) and the18 This is shown in Tables C-1 to C-3 in the online appendix. 19 Estimating “any savings” as a probit (or logit model) does not significantly affect the estimated average treatment effects, as demonstrated in Table C-4 in the online appendix. 20 Responses of “yes” to the first question with responses of zero to the second question (15 responses in all survey rounds) are assumed to indicate that there was zero saving during the reference period.11random disturbance term (ε). Accordingly, fixed-effects (FE) estimation based solely on the variation in the time elapsed since treatment exposure within village panels yields consistent estimates of the duration of post-treatment exposure effects and was used to estimate the models in equations (6) – (8).21 Consistent estimates of the standard errors are obtained by adjusting them for possible heteroscedasticity, serial correlation and clustering within each village panel using the Huber-White sandwich estimator (Wooldridge 2010).Estimates of the post-exposure treatment effects of high versus low agent incentives are in this case based on the observed variation within each village panel in the outcomes between the baseline values (when there were no high agent incentives) and the values of the same outcomes in the follow-up surveys in which some villages had high agent incentives while others had low agent incentives. Differences in outcomes between villages are ignored. Similarly, estimates of the post-exposure effects of the WBO training and mentoring are based on the observed variation within each village panel in the outcomes between the baseline values (when the training had not yet occurred) and the values of the same outcomes in the follow-up surveys in which some WBOs in each village had received the training and mentoring treatment while other WBOs in the same village had not. Variation in outcomes between WBOs in different village panels are ignored. Since there is no variation in the village-level unobserved fixed effect (cj) within each village panel, the treatment measures are uncorrelated with the random disturbance term (ε) in all three models, and the estimates are consistent.6. ResultsVillage-level fixed-effects estimates of Models 1–3 (equations 6–8) are reported in Tables 6– 8. The tables report the estimates of the average treatment effects (using variable names to identify coefficients instead of the Greek letters used in equations 6–8) as well as the coefficients of the dummy variables referring to different survey rounds (the ρs in equations 6–8),22 the p-values for the joint hypotheses tested for each outcome, the baseline sample means, standard deviations and root mean square errors (RMSE) of the outcome in their post-transformation metrics (as described in section 5.1), the R-squared and the size of the estimation sample (N).23Figures 1–8 show graphically the statistically significant predicted effects measured in the common metric of standard deviations from the baseline means together with 95% confidence intervals. Estimates for which the 95% confidence intervals do not bracket a value of zero (that is, are above or below zero) are considered “significant” (at the 0.05 level), while estimates that are significant at only the 0.10 level are referred to as “marginally significant.” The predicted effects are obtained from the estimated regressions reported in21 Fixed-effects estimates based on WBO panels rather than village panels would also be consistent, but they would be inefficient because they would unnecessarily neglect WBO-level variation within each village panel (and would also prevent inclusion of WBO-level covariates from the estimation equation). 22 The estimated coefficients of the ρs in Tables 6–8 are often highly significant. This is not surprising because some of the questions used to obtain the outcome data varied across survey rounds, as discussed in Annex 1. The reasons for significant differences are less clear in other cases, possibly reflecting changes in the outcome over time, differential respondent bias across survey rounds, or (less plausibly) differences in the way the interviews were conducted. 23 The estimated coefficients of the included covariates for each outcome are not reported but are available upon request.12Tables 7–9 using Stata’s “adjust” command.24 The predicted effects for DAW in Figures 1–8 refer to the predicted post-treatment effects of exposure to both treatments combined (e.g., β7+γ7+δ7 in Model 2), not only to their interaction effects (e.g., δ7 in Model 2).Profits. The results in Figure 1 show the predicted effects on profits over successive 6– month post-treatment exposure intervals for the WBO training and mentoring alone (DW) and to both treatments combined (DAW) in all three models (the predicted effects of high agent incentives alone are not shown since they are statistically insignificant in all three models). Beginning in the third six-month interval (13–18 months post-treatment), all six predicted effects are positive and either significant or marginally significant (with the exception of both treatments combined (DAW) in Model 1 in the fourth interval). Figure 1 also shows that the unconstrained predicted effects of both DW and DAW in Model 1 show a tendency to increase in magnitude as the time since first exposure to these two treatments lengthens, although not uniformly. The same tendency is evident as well in the predicted quadratic trends in Model 3.25The joint tests indicate that Models 1–3 provide more accurate estimates of the impact of the treatments on profits than the conventional impact estimation model, which assumes constant effects. For example, the joint hypothesis in Model 1 (Table 6) that the effects of the WBO training and mentoring are equal across intervals is rejected at the 0.05 level. The joint tests also provide a case for preferring the more flexible Models 1 or 3 over the highly constrained Model 2. For example, the joint test of the hypothesis that the linear trend in Model 2 (Table 7) for both treatments combined is constant across the four six-month intervals is rejected at the 0.05 level, while in Model 3 (Table 8) the hypothesis that all three quadratic terms are equal to zero (i.e., that Model 3 is no better than the simpler Model 2) is rejected at the 0.10 level. This suggests that as the time since first exposure to training or training combined with high agent incentives lengthens (beyond the first 12 months), WBOs’ profits increase non-linearly.An important question is whether the delayed impact of the WBO training and mentoring on profits is real or merely reflects a time lag in the measurement of profits (“average monthly profits during the past 12 months”). However, the results clearly support the former interpretation. If the timing of the impacts were immediate, one would expect to see a pattern in year one in Model 1 that is similar to what is observed in year two, i.e., half of the full effect observed in the first six months with the full impact observed in the second six months. What one observes instead is a decreasing estimated effect of training between the first and second six-month intervals in year one, in contrast to increasing predicted effects between the third and fourth six-month intervals in year two.24 The “adjust” command is the predecessor to Stata’s “margins” command, which can do everything that “adjust” can do, with the exception of its “generate()”and “stdf” options (the first of which is used here). Treatment variables and covariates included in the estimation command but not included in the “adjust” variable list (which identifies the treatment variables for which predicted values are calculated) are left at their current values, observation by observation. 25 Additional analysis was done to see whether adjusting for the relatively high rate of attrition in the responses to the question on profits (7.0% in Table 5) affects the estimates reported in Tables 6–8 and Figure 1. The results from using either inverse probability weights or a Heckman sample selection model (using the random assignment to treatment variables to identify the selection equation) are reported in Table D-1 and Figure D-1 in the online appendix and do not show any major differences with the estimates reported in Tables 6–8 and Figure 1.13Capital inputs. The results in Tables 6 and 7 indicate that the total value of business capital inputs is not significantly related (even marginally) to the time since first exposure to any of the treatments in Models 1 or 2, although the results in Table 8 indicate that the value of capital inputs is weakly related to both the linear and quadratic terms in the two treatments combined (DAW) in Model 3. In addition, none of the joint tests for capital inputs in Tables 6–8 is even marginally significant. However, because sample attrition is highest for capital inputs (i.e., 12.7% in both follow-up surveys combined in Table 5), additional analysis was done to see whether adjustments for sample attrition would have any effect. It turns out that they do. Figure 2 compares the predicted effects of both treatments combined (DAW) in Tables 6–8 to those obtained with a Heckman sample selection model.26 The predicted effects based on the Heckman model are positive in all three models, are marginally significant in Model 2 (linear trend) and are almost marginally significant in Model 3 (quadratic trend), suggesting that the combined treatments (DAW) have a positive effect on capita inputs after a significant delay (after 12 months).Labor inputs. Figure 3 indicates that the WBO’s labor inputs (hours worked in a typical month in the primary business) are not significantly related (even marginally) to the predicted effects of the time elapsed since exposure to both treatments combined (DAW), while the results in Tables 6–8 indicate that the number of hours worked is also not significantly related to either treatment alone.27 Unlike capital inputs, however, sample attrition is not relatively high for labor inputs (Table 4), so no adjusted estimates are reported for labor inputs. One possible explanation for the results in Figure 3 is that WBOs choose to work fewer hours in response to higher profits.28 Although one way of doing this might be to close their second businesses, the proportion of WBO’s operating second businesses actually increased over time in all treatment groups (e.g., from 15.5% at baseline to 21.0% among WBOs receiving both treatments combined). In addition, a few of the joint hypotheses pertaining to labor inputs are rejected (as reported in Tables 6–8), including the hypothesis that all agent incentive effects are equal across the four duration of exposure intervals in Model 1 (at the 0.10 level)29 and that there is no interaction effect in both Models 2 and 3 (at the 0.05 level).Business practices. Figure 4 shows that the predicted effects of the combined treatment (DAW) on the proportion of recommended business practices followed are significantly positive in Model 2 and in the second and third six-month post-exposure intervals in Model 3 (no data are available for the first six-month interval because data on business practices were not collected in the first follow-up survey).30 The joint test of the hypothesis that there is no combined effect is also rejected at the 0.05 level in Model 2. However, the results in26 The detailed estimation results using either inverse probability weights or a Heckman sample selection model (using the random assignment to treatment variables to identify the selection equation) are shown in section D of the online appendix. The results indicate that inverse probability weighting had little effect on the estimates. 27 The similarity in the patterns of the predicted effects of the duration of post-treatment exposure on capital and labor inputs in Tables 6–8 is noteworthy, i.e., moderate increases during the first three six-month intervals, followed by a marked decrease in the last six-month interval. However, they differ diametrically from the results for capital inputs obtained with the Heckman sample selection model in Figure 2. 28 WBOs labor inputs were already very high at baseline (i.e., an average of 28.7 days worked per typical month and 8.42 hours worked per typical day in their primary business alone. In addition, 15.5% of WBOs reported owning more than one business, while 13.4% of WBOs reported that they also had wage or salary employment. 29 And similarly, that the linear trend in the effects of agent incentives is constant across the post-treatment exposure intervals in Model 2 (at the 0.10 level). 30 The predicted effects both of high agent incentives alone and of the WBO training and mentoring alone are not shown because they are uniformly insignificant in all three models (Tables 6–8).14Tables 6–8 are also compatible with use of a traditional impact model in which the effects do not vary with the time elapsed since exposure. For example, the joint hypotheses that the treatment effects do not vary across the four six-month intervals in Model 1 or that the effects of the linear trend in the time elapsed since exposure do not vary across the sixmonth intervals in Model 2 are not rejected at conventional levels of significance.Savings. The predicted effects of the WBO training and mentoring on WBO savings are shown in Figures 5 and 6, with Figure 5 showing the predicted effects on the probability of non-zero savings reported and Figure 6 showing the predicted effects on the amount saved among those reporting non-zero savings.31 The results in both Figures 5 and 6 are strikingly similar to those in Figure 4, indicating positive and significant (or marginally significant) predicted effects in the second and third six-month intervals in Models 2 and 3, but not in the fourth time interval in either Model 1 or Model 3 where the unconstrained predicted effects are not even marginally significant. This similarity may reflect behavior that was stressed during the training and mentoring which focused on business practices (especially record keeping) and the importance of saving. The fact that the constrained linear trend in the predicted effects (Model 2) of the business training is positive and significant (or marginally significant) for both business practices and savings indicates that the predicted treatment effects on both business practices and savings increase as the time since first exposure to the business training and mentoring treatment lengthens, as with profits (Figure 1), although to a much lesser degree than for profits.Agency. Figure 7 shows that the predicted effects of all three treatments (DA, DW and DAW) on WBO agency (i.e., participation in household decision-making) differ markedly between Models 1 and 2 (results for Model 3 are not shown because the predicted effects are uniformly insignificant for all three treatments). In Model 1 (where the predicted effects are free to vary across the time since first exposure intervals), the predicted effects of the WBO training and mentoring treatment alone (DW) are largest and highly significant in the first six-month interval (i.e., 1–6 months post-treatment) before decreasing sharply in the second and successive intervals. In Model 2, however, where they are constrained to follow a linear trend, the predicted effects of the WBO training and mentoring treatment alone (DW) are significant and imply continuing increases as the time since first exposure lengthens. How can the Model 1 and Model 2 results be so different and yet both be statistically significant? The answer is that the estimates in Model 2 of the linear trend are driven mainly by the heavy concentration of observations in the second and third post-exposure intervals (i.e., N=1,086 and N=626 respectively, compared to N=508 and N=202 in the first and fourth intervals). In this case, the pattern in the predicted effects obtained with Model 1 is probably more informative about the true time pattern of the effects, i.e., the WBO training and mentoring alone (and to a lesser extent the effect of both treatments combined) had strong significant positive effects on WBO agency in the first six months following first exposure to the treatment(s) that weakened and became insignificant as the time since first exposure lengthened. This is an example of where use of the traditional constant impact model may yield misleading insights.Household welfare. Figure 8 indicates that the predicted effects on household welfare (as measured by a household-level asset index) of exposure to the WBO training and mentoring treatment, whether alone (DW) or combined with high agent incentives (DAW), are31 The predicted effects on savings of high agent incentives alone and to both treatments combined are not shown in Figures 5 and 6 because they are uniformly insignificant in all three models (Tables 6–8).15uniformly positive and highly significant in the third and fourth six-month post-exposure intervals in all three models.32 In Model 1, the unconstrained predicted effects of both treatments are positive and significant (or marginally significant) in all six-month postexposure intervals, except the second (7–12 months), and increase in magnitude with time, from about 0.1 standard deviation after 1–6 months to about 0.2 standard deviations after 19–24 months. The predicted effects of both treatments combined in Model 2 are positive and significant and are constrained to remain so in all four post-exposure intervals. The predicted effects of both treatments combined in Model 3 (quadratic trend), although insignificant in the first two six-month post-exposure intervals increase to almost 0.3 standard deviations in the fourth six-month interval (after 19–24 months). The joint tests reported in Tables 6–8 are inconsistent with the use of a traditional constant impact model. For example, joint hypotheses of equal treatment effects across six-month post-exposure intervals are rejected at the 0.01 level in Model 1 and Model 2. In addition, the results in Model 3 reject the joint hypothesis that the quadratic terms are equal to zero, implying that Model 3 is preferable to Model 2 and suggesting that the predicted positive effects on household welfare increased non-linearly over time.Both the magnitude and pattern of the estimated effects in Figure 8 are similar to those in Figure 1 (profits), which is consistent with the idea that the increase in household assets was financed by the increase in WBO profits. It is also consistent with the idea that most of the increased WBO profits were channeled into the purchase of household assets and less so into business capital (when controlling for sample attrition), rather than being channeled into the WBO’s savings. Whether the decision to use the WBOs’ increased profits in this way was made individually or jointly by the WBO and her spouse is unclear, but the decrease in the WBO’s agency during the last three six-month intervals that is observed in Figure 7 is at least consistent with the idea that her spouse may have played a greater role in the decision of how to use profits. Better business practices and increased agency, even if the latter decreased over time, help to explain WBO’s increased profits.7. ConclusionsThe results of this trial support the notion that comparatively ‘light’ interventions designed to improve women’s economic lives – in this case a short group business training and mentoring plus more motivated bank agents – need to be tracked long enough for businesswomen to manifest new and beneficial behaviors. The unfolding of economic empowerment outcomes takes time, especially for women operating very small firms in rural business environments that are traditionally male dominated.In this trial in predominantly rural villages in East Java, Indonesia, the predicted effects of these ‘light’ treatments on WBO’s firms – which are significantly smaller and less capital intensive and profitable than male-owned firms – are strongly positive on both the shortlived manifestation of agency and the delayed increase in profits. The results are consistent with the notion that improved business practices and increased agency, even if the latter was short-lived, contributed to the rise in profits. Increased profits (and perhaps some savings),32 The predicted values of high agent incentives alone (DA) are not displayed in Figure 8 because, although also uniformly positive in the third and fourth six-month intervals, they are either insignificant or only marginally significant.16in turn, were channeled into the purchase of household assets and may have contributed to some increase in business capital.It is also possible that delays in economic outcomes (profits) are partly the result of women having to practice new behaviors and/or negotiate with others in traditional male dominated household or business environments. The short-lived effect of increased agency remains puzzling, especially because it would be expected that increased agency and increased profits (the subjective and objective dimensions of economic empowerment) are mutually reinforcing. Instead, in this case the idea that her spouse may have played a greater role in the businesswoman’s decision to use business profits to finance household assets suggests that traditional household roles are hard to change, and that they may revert easily even in the presence of women’s new economic behaviors.We now turn to the three questions posed in the introduction regarding the durability of impacts over time, the best time to measure them, and how the time sequence of impacts may be informative about possible causal linkages between them. Starting with the last, the sequence of impacts does suggest some possible causal linkages: from increased agency to increased profits (but not the reverse); from increased profits to increased household welfare (not only are both impacts positive and statistically significant, but they also increase in magnitude pari pasu over time); from better business practices across the post-exposure intervals to increased profits.Second, on when to measure impacts, the results reported here underline the importance of measuring impacts over a sufficiently long period to capture a variety of possible time patterns in impacts. Six months or even a year is probably too short a period to obtain reliable estimates of economic empowerment impacts which, we argue, emerge with a delay especially for women running very small businesses embedded in traditional household and business environments.Third, the predicted effects of the WBO training and mentoring (and to a lesser extent, of both treatments combined) on both profits and household welfare exhibit increasingly positive impacts over time in all three estimation models, particularly during the last two sixmonth intervals, suggesting that these impacts are likely to continue or perhaps even increase in the future. The models estimated in this paper can provide this type of information over the time interval for which data are collected (24 months, in this case), whereas the traditional constant impact estimation models provide misleading results for these outcomes and cannot be used to answer the question as to how likely the estimated impacts will continue in the future, information that is particularly important in the context of the economic analysis of projects.17ReferencesAnderson-MacDonald, S., R. Chandy and B. Zia. 2018. “Pathways to Profits: The Impact of Marketing vs. Finance Skills on Business Performance.” Management Science 64 (12): 5559–5583.Banerjee, A., D. Karlan and J. Zinman. 2015. “Six Randomized Evaluations of Microcredit: Introduction and Further Steps.” American Economic Journal: Applied Economics 7(1): 1–21Bell, M., M. Kenward, D. Fairclough, and N. Horton. 2013. “Differential dropout and bias in randomized controlled trials: when it matters and when it may not.” British Medical Journal 346: e8668.Bellemarre, M. and C. Wichman. 2019. “Elasticities and the Inverse Hyperbolic Sine Transformation,” Oxford Bulletin of Economics and Statistics, July:1–12.Buvinic, M., H. C. Johnson, E. Perova, and F. Witolear. 2020 “Can boosting savings and skills support female business owners in Indonesia? Evidence from a Randomized Controlled Trail,” East Asia and Pacific Gender Innovation Lab (EAPGIL), World Bank Group (March).Buvinic, M., J. Knowles and F. Witolear. 2018. “Unequal Ventures: Results from a Baseline Study of Gender and Entrepreneurship in East Java, Indonesia.” Evidence-based Report Series on Women’s Economic Empowerment, Center for Global Development, Washington, DC (April).Buvinic, M. and M. O’Donnell. 2019. “Gender Matters in Economic Empowerment Interventions: A Research Review,” World Bank Research ObserverCalderon, G., J. M. Cunha and G. De Giorgi. 2013. “Business Literacy and development: Evidence from a randomized controlled trial in rural Mexico.” Working Paper No. 19740, National Bureau of Research.De Mel, S., D. McKenzie and C. Woodruff. 2014. “Business training and female enterprise startup, growth, and dynamics: Experimental evidence from Sri Lanka.” Journal of Development Economics 106(C): 199–2010.Deserrano, E., G. Leon-Ciliotta and F. Witoelar. 2021. “When Transparency Fails: Financial Incentives for Local Banking Agents in Indonesia,” Unpublished manuscript (January)Dumville, J. C., D. J. Torgerson and C. E. Hewitt. 2006. “Reporting attrition in randomized controlled trials,” British Medical Journal 332(7547):969–71.Goldberger, Arthur S. 1968. “The Interpretation and Estimation of Cobb-Douglas Functions,” Econometrica 36(3):464–72.Huis, M., R. Lensink, N. Vu and N. Hansen. 2019. “Impacts of the Gender and Entrepreneurship Together Ahead (GET Ahead) training on empowerment of female microfinance borrowers in Northern Vietnam,” World Development, 120: 46–61.Kennedy, P. E. 1981. “Estimation with correctly interpreted dummy variables in semilogarithmic equations,” American Economic Review 71 (4): 801.King, E. M. and J. R. Behrman. 2009. “Timing and Duration of Exposure in Evaluations of Social Programs.” World Bank Research Observer 24 (1): 55–82.Knowles, J. C. 2019a. “Midline Effects of a Randomized Controlled Trial to Increase the Utilization of Financial Services by Women Business Owners in Rural Indonesia.” Working Paper 506, Center for Global Development, Washington DC (March).Knowles, J. C. 2019b. “Some caveats when using the IHS transformation,” Processed (August).Lin, W., D. P. Green, and A. Coppock. 2016. “Standard operating procedures for Don Green’s lab at Columbia.” Version 1.05 (June 7, 2016). https://alexandercoppock.com/Green-Lab-SOP/Green_Lab_SOP.html18McKenzie, D. and S. Puerto. 2017. “Growing Markets through Business Training for Female Entrepreneurs: A Market-Level Randomized Experiment in Kenya,” Policy Research Working Paper 7993, The World Bank, Washington, D.C.Millan, T. and K. Macours. 2017. “Attrition in Randomized Control Trials: Using Tracking Information to Correct Bias.” IZA Discussion Paper No. 10711, Institute of Labor Economics, Bonn (April).Valdivia, M. 2015. “Business training plus for female entrepreneurship? Short and mediumterm experimental evidence from Peru.” Journal of Development Economics 113(C): 33–51.Wooldridge, Jeffrey M. 2010. Econometric Analysis for Cross-section and Panel Data. Second edition. Cambridge, MA: MIT Press.World Bank. 2016. Women Entrepreneurs in Indonesia: A Pathway to Increasing Shared Prosperity. Finance and Markets Global Practice, East Asia Pacific Region, Jakarta, Indonesia.19Figures and tablesFigure 1. Predicted effects of the treatments on business profits over four six-month intervals of post-treatment exposure (with 95% confidence intervals adjusted for clustered sampling)Standard deviations0.60.50.40.30.20.10-0.11-6 months7-12 months 13-18 months 19-24 months-0.2 Time since first exposure to treatmentsModel 1 (DW) Model 1 (DAW) Model 2 (DW) Model 2 (DAW) Model 3 (DW) Model 3 (DAW)Source: Tables 7–9 (see text for prediction methods)Figure 2. Predicted effects of the treatments on capital inputs over four six-month intervals of post-treatment exposure (with 95% confidence intervals adjusted for clustered sampling), showing alternative Heckman model estimates to adjust forsample attritionStandard deviations0.50.40.30.20.10-0.1 1-6 months7-12 months 13-18 months 19-24 months-0.2-0.3-0.4-0.5-0.6 Time since first exposure to treatments (DAW)Model 1 (Table 7) Model 2 (Table 8) Model 3 (Table 9)Model 1 (Heckman model) Model 2 (Heckman model) Model 3 (Heckman model)Source: Table 6–8 and Online Appendix Table D-2 (see text for prediction methods)20Figure 3. Predicted effects of the treatments on labor inputs over four six-month intervals of post-treatment exposure (with 95% confidence intervals adjusted forclustered sampling)Standard deviations0.30.20.10-0.1-0.2-0.3-0.41-6 months7-12 months 13-18 months 19-24 monthsTime since first exposure to treatmentsModel 1 (DAW) Model 2 (DAW) Model 3 (DAW)Source: Tables 6–8 (see text for prediction methods)Figure 4. Predicted effects of the treatments on adherence to recommended business practices over three* six-month intervals of post-treatment exposure (with 95% confidence intervals adjusted for clustered sampling)Standard deviatons0.50.40.30.20.101-6 months -0.17-12 months 13-18 months 19-24 months-0.2 Time since first exposure to treatmentsModel 1 (DAW) Model 2 (DAW) Model 3 (DAW)Source: Tables 6–8 (see text for prediction methods)*No estimates are available for the first six-month interval because no data on business practices were collected in the first follow-up survey.21Figure 5. Predicted effects of the treatments on any non-zero reported savings over four six-month intervals of post-treatment exposure (with 95% confidence intervalsadjusted for clustered sampling)0.30.2Standard deviations0.10 1-6 months-0.17-12 months 13-18 months 19-24 months-0.2 Time since first exposure to treatmentsModel 1 (DW) Model 2 (DW) Model 3 (DW)Source: Tables 6–8 (see text for prediction methods)Figure 6. Predicted effects of the treatments on the amount of non-zero savings over four six-month intervals of post-treatment exposure (with 95% confidence intervalsadjusted for clustered sampling)0.40.3Standard deviations0.20.10 1-6 months-0.17-12 months 13-18 months 19-24 months-0.2 Time since first exposure to treatmentsModel 1 (DW) Model 2 (DW) Model 3 (DW)Source: Tables 6–8 (see text for prediction methods)22Figure 7. Predicted effects of the treatments on WBO agency over four six-month intervals of post-treatment exposure (with 95% confidence intervals adjusted forclustered sampling)Standard deviations0.40.30.20.10-0.11-6 months7-12 months 13-18 months 19-24 months-0.2-0.3-0.4 Time since first exposure to treatmentsModel 1 (DA) Model 2 (DA)Model 1 (DW) Model 1 (DAW) Model 2 (DW) Model 2 (DAW)Source: Tables 6–8 (see text for prediction methods)Figure 8. Predicted effects of the treatments on household welfare over four sixmonth intervals of post-treatment exposure (with 95% confidence intervals adjustedfor clustered sampling)Standard deviations0.50.40.30.20.10-0.1-0.2 1-6 months7-12 months 13-18 months 19-24 monthsTime since first exposure to treatmentsModel 1 (DW) Model 1 (DAW) Model 2 (DW) Model 2 (DAW) Model 3 (DW) Model 3 (DAW)Source: Tables 6–8 (see text for prediction methods)23Table 1. Observations by time elapsed since assigned treatment and follow-up survey rounds (frequencies)Treatment→ Follow-up survey round→Assigned to treatment 1–6 months 7–12 months 13–18 months 19–24 months Not assigned to treatment Total sampleAgent incentivesWBO training & mentoring Both treatmentsFirstSecond Total FirstSecond Total FirstSecond(1)(2)(3) (4)(5)(6) (7)(8)6511,363 2,014 8001,600 2,400 37276438551436 5080508 24814140466 606 292772 1,064 124371126580 706 0626 626 02860266 266 0202 202 093748 1,3991,478 2,226 599 2,841 4,240 1,3991,241 1,840 1,027 2,841 4,240 1,3992,077 2,841Note: 11 WBOs were not included in the second follow-up survey sample, including 4 who were randomly assigned to the WBO training and mentoring treatment.Total(9)1,136 262 495 286 933,104 4,240Table 2. Conditional mean of time elapsed (in months) by treatment and by month in which the baseline survey interview was conducted (see note)Baseline survey monthNov-16 Dec-16 Jan-17 Feb-17 Jul-17 Aug-17 Sep-17 Oct-17 Nov-17 TotalAgent incentivesMeanN(1) 18.44 14.71 15.13 13.00 10.89 10.25 11.40 10.19 10.00 12.09(2) 210 304 32 49 367 615 190 170 114 2,051WBO training & mentoringMeanN(3) 15.88 13.48 12.73 11.33 9.07 9.26 9.56 9.29 9.62 10.80(4) 238 448 22 60 395 713 188 204 136 2,404Both treatmentsMeanN(5) 15.93 12.94 12.56 9.86 9.33 8.86 9.64 8.52 9.03 10.43(6) 120 176 16 28 209 351 108 88 64 1,160Note: Mean time elapsed since exposure in this table are conditional on sample WBOs receiving each treatment. Mean time elapsed depend on both the month in which the relevant training began (i.e., agent training in column (1), WBO training in column (3), both in column (5) and on the month in which the WBO was interviewed in the two follow-up surveys.Table 3. Mean time elapsed (months) since exposure to treatments in the second follow-up survey according to whether the village was included in the first follow-upsurvey sampleIncluded in first follow-up survey sample: Yes No Overall mean (second follow-up survey)Agent incentives WBO training & Both treatments N mentoring8.038.784.081,3985.696.092.881,4436.847.423.472,84124Table 4. Characteristics of the eight outcomes analyzed by survey roundBaseline survey (both phases) Profits Capital inputs Labor inputs Business practices Any savings Non-zero level of savings HH decisionmaking HH welfare First follow-up survey Profits Capital inputs Labor inputs Business practices Any savings Non-zero level of savings HH decisionmaking HH welfare Second follow-up survey Profits Capital inputs Labor inputs Business practices Any savingsMean(1)1.651 21.016 244.855 0.228 0.8377.0960.794 0.0401.802 24.793 225.850 NA 0.8455.0110.841 -0.0641.755 27.920 198.633 0.283 0.914Standard deviation(2)3.065 71.502 104.188 0.186 0.36913.7590.293 1.8182.717 84.092 105.244 NA 0.36219.7120.261 1.6809.347 90.800 98.004 0.227 0.280Minimum value(3)0.0000 0.0268 1.0000 0.0000 0.00000.00190.0000 -5.3220.0000 0.0000 0.0000 NA 0.00000.00010.0000 -3.3820.0000 0.0000 0.0000 0.0000 0.0000Maximum value(4)101.1 1622.4 720.0 1.0 1.0246.11.0 7.04236.6 2035.8 630.0 NA 1.0370.21.0 10.571465.7 2355.1 570.0 1.0 1.0Median value(5)0.963 4.574 240.000 0.154 1.0002.9291.000 0.0320.962 5.957 230.000 NA 1.0001.2271.000 -0.3840.931 7.591 182.000 0.231 1.000No. of zero values (6)1 0 0 124 46410147 01 10 1 NA 208146 01 3 1 101 234No. of reported values (7)2,835 2,685 2,849 2,846 2,8502,3762,846 2,8471,312 1,324 1,325 0 1,3451,1361,345 1,3452,655 2,600 2,679 2,679 2,724No. of nonresponses (8)17 167 3 6 206 587 75 74 0 545454 54186 241 162 162 117Total sample(9)2,852 2,852 2,852 2,852 2,8522,3862,852 2,8521,399 1,399 1,399 0 1,3991,1911,399 1,3992,841 2,841 2,841 2,841 2,84125Table 4. Characteristics of the eight outcomes analyzed by survey round, continuedNon-zero level of savings HH decisionmaking HH welfare Pooled data Profits Capital inputs Labor inputs Business practices Any savings Non-zero level of savings HH decisionmaking HH welfareMean(1)7.5650.754 -0.0441.721 24.489 223.111 0.255 0.8696.8920.787 -0.013Standard deviation(2)14.1550.318 1.4926.280 82.126 104.107 0.209 0.33715.2500.299 1.670Minimum value(3)0.00920.0000 -4.1750.0000 0.0000 0.0000 0.0000 0.00000.00010.0000 -5.322Maximum value(4)254.11.0 9.731465.7 2355.1 720.0 1.0 1.0370.21.0 10.571Median value(5)3.1651.000 -0.2460.946 5.972 210.000 0.154 1.0002.6021.000 -0.190No. of zero values (6)4181 03 13 2 225 90615374 0No. of reported values (7)2,4572,724 2,7246,802 6,609 6,853 5,525 6,9195,9696,915 6,916No. of nonresponses (8)146117 117290 483 239 168 173200177 176Total sample(9)2,6072,841 2,8417,092 7,092 7,092 5,693 7,09261847,092 7,092NA=no data collected. HH=household.Notes: The data on outcomes in this table are in their original (pre-transformed) metrics, with any subsequent transformations described in section 5.1. Detailed information on the individual outcomes is provided in Annex 1. Non-responses in column 8 include 54 WBOs in the first follow-up survey and 117 WBOs in the second follow-up survey who were interviewed in the baseline survey but who could not be interviewed in the follow-up survey. Units for profits, capital inputs and non-zero levels of savings are Rupiah (Rp.) millions in November 2016 Surabaya Municipality prices, units for labor inputs are number of days, units for business practices, any savings, and household decision-making are proportions. The values reported for household welfare are the predicted scores of the first principal components of indicators referring to household ownership of 20 durable goods.26Table 5. Multivariate analysis of attrition in the two follow-up surveysDependent variables →Estimation method →TREATMENTS High agent incentivesWBO training & mentoring COVARIATES (Baseline values) Location (urban=1, rural=0)WBO’s ageWBO’s age squaredWBO is currently married WBO has childrenNumber of WBO’s children WBO’s household sizeWBO’s highest completed level of schooling WBO’s cognitive score (1–4) WBO owns a smart phone WBO knows partner bank agent’s name Number of other sample WBOs who are known by WBO Number of other sample WBOs who are close friends, relatives or family members of WBO Number of other sample WBOs with whom WBO talks weekly Number of other sample WBOs with whom WBO talks weekly about business matters Primary business is registeredOverall sample attritionOLS (1)0.000 (0.007) -0.008 (0.008)-0.007(0.012) 0.005 (0.004) -0.000 (0.000) 0.001 (0.011) 0.004 (0.010) -0.011** (0.005) -0.002 (0.002) 0.001 (0.004)-0.007 (0.004) 0.010 (0.007) 0.001 (0.008) -0.005* (0.003)-0.004 (0.004)-0.001 (0.003)-0.001 (0.003)-0.001 (0.011)Attrition of profits outcomeaOLS (2)0.008 (0.009) -0.018* (0.010)0.001(0.014) 0.004 (0.005) -0.000 (0.000) -0.015 (0.016) 0.012 (0.013) -0.016** (0.006) -0.001 (0.002) 0.012** (0.005)-0.011* (0.006) 0.005 (0.010) -0.008 (0.010) -0.007* (0.004)-0.001 (0.005)-0.001 (0.004)-0.005 (0.003)-0.008 (0.013)Attrition of capital inputs outcomea OLS (3)0.010 (0.015) -0.025** (0.012)-0.030(0.027) 0.004 (0.007) 0.000 (0.000) -0.024 (0.023) 0.007 (0.015) -0.009 (0.009) 0.000 (0.004) 0.022*** (0.008)-0.027*** (0.008) 0.013 (0.012) -0.025* (0.015) -0.005 (0.005)-0.005 (0.007)-0.002 (0.006)-0.010* (0.005)-0.028 (0.019)Overall sample attritionVL_FEb (4)NAc-0.008 (0.008)NAc0.002 (0.004) -0.000 (0.000) -0.003 (0.012) 0.008 (0.011) -0.008 (0.005) -0.002 (0.002) 0.001 (0.006)-0.008 (0.005) -0.001 (0.008) 0.004 (0.009) -0.005 (0.003)-0.005 (0.004)0.001 (0.003)0.002 (0.003)0.004 (0.012)Attrition of Attrition ofprofitscapital inputsoutcomea outcomeaVL_FEb (5)VL_FEb (6)NAcNAc-0.019* (0.010)-0.024** (0.012)NAcNAc0.004 (0.005) -0.000 (0.000) -0.019 (0.018) 0.014 (0.014) -0.011* (0.007) 0.000 (0.002) 0.014** (0.007)-0.009 (0.006) -0.008 (0.010) -0.006 (0.012) -0.009** (0.004)-0.005 (0.005)0.004 (0.006) 0.000 (0.000) -0.030 (0.023) 0.019 (0.014) -0.001 (0.009) 0.001 (0.004) 0.020** (0.009)-0.016* (0.009) 0.005 (0.013) -0.032** (0.016) -0.006 (0.005)-0.000 (0.007)0.002 (0.004)0.002 (0.004)0.001 (0.006)-0.005 (0.006)-0.011 (0.015)-0.016 (0.019)27Table 5. Multivariate analysis of attrition in the two follow-up surveys, continuedDependent variables →Overall Attrition of sample profits attrition outcomeaEstimation method → OLS(1)OTHER STATISTICSJoint test: randomized 0.548treatments=0 (p)Joint test: covariates=0 0.029**(p)Sample mean0.040R-squared0.014Sample size4240*** p<0.01 ** p<0.05 * p<0.10OLS (2)0.1070.000***0.070 0.019 4240Attrition of capital inputs outcomea OLS (3)0.083*0.000***0.127 0.026 4240Overall sample attritionVL_FEb (4)0.2890.2800.040 0.010 4240Attrition of Attrition ofprofitscapital inputsoutcomea outcomeaVL_FEb (5)VL_FEb (6)0.0560.034**0.0340.001***0.070 0.013 42400.127 0.018 4240Notes: Estimation sample includes data from both follow-up surveys. Estimated standard errors are adjusted for heteroscedasticity and for serial correlation and clustering at the village level.a Includes attrition in baseline values. b Village-level fixed-effects. c Not available (village-level variable)28Table 6. Village-level fixed-effects estimates of Model 1 (treatment effects permitted to vary freely between six-month post-treatment exposure intervals)TREATMENT EFFECTS DA1: 1–6 monthsDA2: 7–12 monthsDA:3 13–18 monthsDA4: 19–24 monthsDW1: 1–6 monthsDW2: 7–12 monthsDW3: 13–18 monthsDW4: 19–24 monthsDAW1: 1–6 monthsDAW2: 7–12 monthsDAW3: 13–18 monthsDAW4: 19–24 monthsSURVEY ROUND EFFECTS Baseline survey (phase 2)First follow-up surveySecond follow-up surveyJOINT TESTS No agent incentives effect (DA1– DA4=0) No WBO training effect (DW1– DW4=0) No interaction effect (DAW1– DAW4=0) No combined effect (ΣDA+ΣDW+ΣDAW=0)Profits Capital (IHS) inputs(IHS)Labor inputs(1)(2)(3)Business Any practices savings(4)(5)Nonzero level of savings (log) (6)Household Household decision- welfare making(7)(8)-0.116 (0.098) -0.041 (0.069) -0.042 (0.071) 0.011 (0.115) 0.040 (0.091) 0.016 (0.061) 0.165** (0.068) 0.288** (0.135) 0.137 (0.131) 0.117 (0.087) 0.008 (0.107) -0.385* (0.200)-0.040 (0.136) 0.035 (0.115) -0.086 (0.101) -0.049 (0.167) 0.096 (0.111) 0.089 (0.100) -0.061 (0.104) 0.210 (0.200) 0.112 (0.175) -0.026 (0.148) 0.233 (0.160) -0.509 (0.324)-5.761 (8.988) 6.733 (7.623) -9.502 (7.191) 9.891 (10.403) -8.621 (8.573) 1.861 (6.265) -3.541 (6.370) -12.666 (11.178) 18.381 (11.886) 5.013 (8.699) 23.935** (9.408) 2.528 (16.132)-0.019 (0.026) 0.026 (0.018) -0.012 (0.016) -0.015 (0.026) NA0.019 (0.014) 0.014 (0.015) 0.034 (0.022) -0.048 (0.038) 0.001 (0.021) 0.039* (0.022) -0.005 (0.041)-0.006 (0.036) 0.009 (0.024) 0.031 (0.026) 0.020 (0.036) 0.026 (0.028) 0.029 (0.018) 0.017 (0.021) 0.023 (0.034) -0.031 (0.042) -0.017 (0.027) -0.010 (0.033) 0.040 (0.061)-0.168 (0.157) 0.055 (0.105) -0.142 (0.103) -0.203 (0.173) 0.088 (0.136) 0.132* (0.077) 0.072 (0.103) 0.131 (0.179) 0.095 (0.187) -0.031 (0.112) -0.034 (0.141) 0.183 (0.248)0.047* (0.026) -0.004 (0.025) 0.050** (0.023) 0.040 (0.031) 0.062*** (0.023) 0.028 (0.018) 0.024 (0.020) 0.023 (0.033) -0.049 (0.031) -0.028 (0.027) -0.013 (0.031) -0.104* (0.059)0.129 (0.130) -0.033 (0.101) 0.345*** (0.106) 0.140 (0.144) 0.212** (0.099) -0.057 (0.070) 0.321*** (0.084) 0.359** (0.157) -0.134 (0.139) -0.013 (0.104) -0.349*** (0.132) -0.010 (0.232)-0.000 (0.060) 0.132**(0.058) -0.020(0.071)0.000 (0.090) 0.044(0.095) 0.412***(0.109)-2.838 (6.638) 18.792*** (5.982) 50.245*** (7.287)-0.016 (0.017) NA0.028* (0.016)0.051** (0.023) 0.042*(0.023) 0.094***-0.066 (0.106) 0.824*** (0.099) 0.0960.003 (0.018) -0.011(0.019) -0.057***(0.025) (0.119) (0.020)0.368*** (0.089) 0.249***(0.085) 0.075(0.091)0.818 0.809 0.1680.236 0.763 0.204 0.085*0.001***0.022** 0.444 0.4560.265 0.535 0.548 0.090*0.000***0.041 0.235 0.1220.188 0.829 0.877 0.1970.1080.431 0.992 0.2620.567 0.163 0.613 0.3640.012**29Table 6. Village-level fixed-effects estimates of Model 1 (treatment effects permitted to vary freely between sixmonth post-treatment exposure intervals), continuedProfits Capital (IHS) inputs(IHS)Equal effects across intervals (DA1=DA2=DA3=DA4)a Equal effects across intervals (DW1=DW2=DW3=DW4)a Equal effects across intervals (DAW1=DAW2=DAW3=DAW4)a OTHER STATISTICS Baseline sample mean Baseline standard deviation Baseline RMSE R-squared Sample size(1) 0.8350.020**0.039**7.497 1.125 0.917 0.178 6780(2) 0.7050.3300.1579.191 1.661 1.406 0.190 6387Labor inputs(3) 0.093*0.4210.362244.855 104.188 73.359 0.435 6853Business Any practices savings(4) 0.138(5) 0.691Nonzero level of savings (log) (6) 0.155Household decisionmaking(7) 0.1230.703 0.952 0.944 0.4110.284 0.787 0.752 0.3560.228 0.186 0.142 0.481 55250.834 0.372 0.274 0.289 69191.012 1.478 1.233 0.230 59690.794 0.293 0.229 0.336 6915*** p<0.01 ** p<0.05 * p<0.10a test of Model 1 vs constant impact modelNotes: NA=not available (no data on business practices were collected in the first follow-up survey). The baseline sample means are in their pre-transformation metrics, with profits, capital inputs and the non-zero level of savings in Rp. millions in November 2016 Surabaya Municipality prices.Household welfare(8) 0.001***0.000***0.1050.040 1.818 0.966 0.593 691630Table 7. Village-level fixed-effects estimates of Model 2 (Linear trends in treatment effects)Profits (IHS)Capital inputs (IHS)Labor inputsBusiness Any practices savings(1)(2)(3)(4)(5)TREATMENT EFFECTSDA-0.0016 -0.0035 -0.1818 -0.0007 0.0018(0.0044) (0.0063) (0.4065) (0.0008) (0.0015)DW0.0089** 0.0035 -0.3106 0.0011 0.0019(0.0043) (0.0067) (0.3515) (0.0008) (0.0012)DAW0.0005 0.0018 1.1544** 0.0016 -0.0007(0.0065) (0.0100) (0.4968) (0.0012) (0.0019)SURVEY ROUND EFFECTSBaseline survey (phase 2) 0.0056 -0.0456 -2.0935 -0.0262** 0.0482**(0.0530) (0.0810) (5.9785) (0.0121) (0.0205)First follow-up survey 0.1176** 0.0809 -18.445*** NA0.0398*(0.0520) (0.0853) (5.6702)(0.0209)Second follow-up survey -0.0239 0.3847*** -48.079*** 0.0248* 0.0895***(0.0683) (0.1081) (6.9121) (0.0142) (0.0244)JOINT TESTSAgent incentives have 0.716 0.5740.6550.438 0.219zero effect (DA=0)WBO training has no 0.041** 0.6050.3770.139 0.106effect (DW=0)No interaction effect 0.933 0.8550.021** 0.171 0.716(DAW=0)No combined effect0.071* 0.8020.1380.018** 0.053*(DA+DW+DAW=0)DA effect is constant 0.696 0.6300.079* 0.155 0.693across six-monthintervals (test of Model 2vs Model 1)DW effect is constant 0.104 0.8580.5950.665 0.706across six-monthintervals (test of Model 2vs Model 1)DAW effect does not 0.015** 0.1500.4440.709 0.993vary across six-monthintervals (test of Model 2vs Model 1)OTHER STATISTICSBaseline sample mean 7.497 9.191244.855 0.228 0.834Baseline standard1.125 1.661104.188 0.186 0.372deviationBaseline RMSE0.917 1.40773.409 0.142 0.274R-squared0.177 0.1890.4330.480 0.289Sample size6780 638768535525 6919*** p<0.01 ** p<0.05 * p<0.10Non-zero level of savings (log) (6)Household decisionmaking(7)-0.0083 (0.0061) 0.0074 (0.0055) 0.0019 (0.0073)0.0025* (0.0014) 0.0022* (0.0012) -0.0031* (0.0018)-0.0905 -0.0161 (0.0909) (0.0161) -0.7956*** -0.0021 (0.0919) (0.0164) 0.0923 -0.0733*** (0.1143) (0.0203)0.1730.063*0.1780.064*0.7930.078*0.8730.2520.1180.1930.9740.093*0.7620.1081.012 1.4781.233 0.228 59690.794 0.2930.229 0.334 6915Household welfare(8)0.0149** (0.0060) 0.0147*** (0.0049) -0.0129* (0.0072)0.3661*** (0.0830) 0.2295*** (0.0747) 0.0297 (0.0923)0.013** 0.003*** 0.075* 0.006*** 0.001***0.001***0.092*0.040 1.818 0.969 0.590 6916Notes: RMSE=root mean square error. NA=not available (no data on business practices were collected in the first follow-up survey). The baseline sample means are in their pre-transformation metrics, with profits, capital inputs and the non-zero level of savings in Rp. millions in November 2016 Surabaya Municipality prices.31Table 8. Village-level fixed-effects estimates of Model 3 (Quadratic trends in treatment effects)Profits (IHS)Capital inputs (IHS)(1)(2)TREATMENT EFFECTSDA-0.0155 -0.0182(0.0107) (0.0168)DA-squared (DA2)0.0008 0.0009(0.0006) (0.0009)DW-0.0034 -0.0028(0.0109) (0.0164)DW-squared (DW2)0.0008 0.0004(0.0006) (0.0010)DAW0.0410** 0.0460*(0.0169) (0.0265)DAW-squared (DAW2) -0.0028*** -0.0031*(0.0010) (0.0016)SURVEY ROUND EFFECTSBaseline survey-0.0114 -0.0463(phase 2)(0.0568) (0.0880)First follow-up survey 0.1216** 0.0794(0.0530) (0.0863)Second follow-up survey -0.0315 0.3863***(0.0681) (0.1075)JOINT TESTSAgent incentives have 0.3260.550zero effect (DA=DA2=0)WBO training has no 0.047** 0.777effect (DW=DW2=0)No interaction effect 0.025** 0.142(DAW=DAW2=0)No combined effect0.061* 0.189(DA+DA2+DW+DW2+DAW+DAW2=0)DA2=DW2=DAW2=0 0.058* 0.219(test of Model 3 vs Model2)OTHER STATISTICSBaseline sample mean 7.4979.191Baseline standard1.1251.661deviationBaseline RMSE0.9171.406R-squared0.1780.190Sample size67806387Labor inputsBusiness practices(3)(4)0.2764 (1.1868) -0.0251 (0.0620) 0.8304 (1.1257) -0.0771 (0.0679) 0.5498 (1.6873) 0.0392 (0.1011)0.0037 (0.0030) -0.0002 (0.0002) 0.0023 (0.0029) -0.0001 (0.0002) -0.0012 (0.0046) 0.0002 (0.0003)0.9981 -0.0133 (6.2725) (0.0166) -19.322*** NA (5.7513) -46.438*** 0.0307** (6.9024) (0.0153)0.8350.2580.2780.2810.041** 0.3740.1740.1380.6270.511244.855 104.18873.409 0.433 68530.228 0.1860.142 0.480 5525Any savings(5)-0.0013 (0.0039) 0.0002 (0.0002) 0.0049 (0.0032) -0.0002 (0.0002) -0.0021 (0.0057) 0.0001 (0.0004)0.0467** (0.0221) 0.0405* (0.0215) 0.0900*** (0.0244)0.2600.1960.8950.6980.4190.834 0.3720.274 0.289 6919Non-zero level of savings (log) (6)Household decisionmaking(7)-0.0017 (0.0166) -0.0004 (0.0009) 0.0152 (0.0146) -0.0005 (0.0009) -0.0074 (0.0225) 0.0006 (0.0014)0.0006 (0.0038) 0.0001 (0.0002) 0.0064** (0.0032) -0.0003 (0.0002) -0.0014 (0.0055) -0.0001 (0.0004)-0.0671 -0.0084 (0.1027) (0.0180) -0.8028*** -0.0043 (0.0927) (0.0166) 0.1038 -0.0684*** (0.1171) (0.0204)0.3730.1170.3530.084*0.8760.1580.7130.1280.9340.2101.012 1.4781.233 0.228 59690.794 0.2930.229 0.335 6915Household welfare(8)0.0019 (0.0150) 0.0007 (0.0007) -0.0166 (0.0126) 0.0021*** (0.0008) 0.0069 (0.0208) -0.0013 (0.0013)0.2845*** (0.0900) 0.2537*** (0.0761) -0.0126 (0.0942)0.020**0.001***0.1500.6720.046**0.040 1.8180.968 0.591 6916*** p<0.01 ** p<0.05 * p<0.10Notes: RMSE=root mean square error. NA=not available (no data on business practices were collected in the first follow-up survey). The baseline sample means are in their pre-transformation metrics, with profits, capital inputs and the non-zero level of savings in Rp. millions in November 2016 Surabaya Municipality prices.32Annex 1. Description of the outcomes analyzedProfits in all survey rounds is average monthly profits during the past 12 months in the primary and secondary business combined in millions of Indonesia Rupiah (Rp. millions) in November 2016 Surabaya Municipality prices.33 It is based on a single question and with 3 zero responses (1 in the baseline survey, 1 in the first follow-up survey and 1 in the second follow-up survey) and no negative responses. Several WBOs failed to respond to the question on business profits: 17 in the baseline survey, 87 in the first follow-up survey, and 186 in the second follow-up survey.34Capital inputs is the value of all business capital in all businesses owned by the WBO in millions of Indonesia Rupiah (Rp. millions) in November 2016 Surabaya Municipality prices. It is based on questions of whether the WBO has any of six different types of business capital (i.e., own shop premises, advances paid for rented shop, furniture and fixtures, equipment, inventories, and other), and if so, its current value (i.e., “how much would you sell this for?”). There are only 13 zero values in the follow-up surveys (10 in the first followup survey and 3 in the second follow-up survey), but there are significant numbers of nonresponses (mostly responses of “don’t know” with relatively few “refuse to answer” responses): 167 in the baseline survey, 75 in the first follow-up survey, and 241 in the second follow-up survey.Labor inputs is the number of hours worked in a typical month by the WBO in her primary business.35 This variable is the product of responses to the following two questions: (1) “How many days do you work in your primary business during a typical month?” and (2) “How many hours per day do you work in your primary business?” Many WBOs responded that they work 30 days per month (5,132), while 57 WBOs reported that they worked 16 or more hours per day. There are only two zero responses (1 each in the first and second follow-up surveys). Although only 3 WBOs did not report labor inputs in the baseline survey, 74 did not respond in the first follow-up survey and 162 did not respond in the second follow-up survey.Business practices is the mean proportion of WBOs indicating that they adhere to the following 13 recommended business practices: (1) ask a supplier which products are selling well in your industry, (2) used a special offer to attract customers in the last three months, (3) done any form of advertising in the last six months, (4) done anything to measure the effectiveness of advertising (coded zero if (3) is no), (5) attempted to negotiate with a supplier for a lower price in the last three months, (6) have a record-keeping system that allows you to know your current inventory, (7) keep written business records, (8) record every purchase or sale (coded zero if (7) is no), (9) able to know cash on hand at any point in hand (coded zero if (7) is no) (10) use records to know whether sales of a particular product are increasing or decreasing from one month to another (coded zero if (7) is no), (11) worked out the cost of each product sold, (12) have a written monthly budget, and (13) have records needed to apply for a bank loan. Only six WBOs did not respond to these questions in the baseline survey, while 162 did not respond in the second follow-up survey (no data on33 Profits in the secondary business were assumed to have been zero if the WBO reported not having any secondary business. 34 The number of non-responses in the two follow-up surveys include 54 WBOs who could not be interviewed in the first follow-up survey and 177 WBOs who could not be interviewed in the second follow-up survey. 35 WBOs total labor inputs in both primary and secondary businesses is not meaningful because of the tendency of WBOs to report the same labor inputs in both businesses.33adherence to recommended business practices were collected in the first follow-up survey). There are 124 zero responses in the baseline survey and 101 in the second follow-up survey.Savings refers to WBOs’ total reported savings during the past 12 months (or past 3 months in the first follow-up survey) in all savings instruments. It is based on responses to a question whether any amount was saved during the reference period (any savings) and if so, how much was saved in each of several alternative savings instruments (non-zero level of savings in millions of Indonesia Rupiah in November 2016 Surabaya Municipality prices). The list of alternative savings instruments varies to some extent between survey rounds. In the baseline survey, they include: (1) formal bank account, (2) electronic savings account, (3) hiding place at home, (4) with friends or family, (5) cooperative, (6) informal saving network, (7) community savings fund (BMT), (8) rotating saving/credit association (ROSCA), and (9) other. The following savings instruments were added in the first follow-up survey: (10) other household members’ saving, (11) physical assets (e.g., jewelry), (12) laku pandai (LP)36 savings account of the partner bank, (13) electronic savings account of another bank,37 and (14) LP savings account of another bank. In the second follow-up survey, the savings instruments included those in the baseline survey plus (10) LP savings account of the partner bank and (11) cash wallet account of the partner bank, while saving instrument (2) was changed to “electronic savings account in other banks.” The change in the reference period in the first follow-up survey and the changes in the lists of savings instruments need to be taken into account in the analysis. Although all but two WBOs responded to both savings questions in the baseline survey, 54 did not respond to either question on savings in the first follow-up survey, while 117 did not respond to the question on any savings and 146 did not respond to the question on the non-zero level of savings in the second follow-up survey.Household decision-making is the average proportion of WBOs who indicate that that they are either the sole decision-maker or participate with their spouse/partner or with another household member in the following five decisions: (1) whether to purchase an appliance for the home, (2) how household members may work outside the home, (3) whether to support other family members, (4) whether to save for the future, and (5) whether to sign up for a new banking product. There are 147 zero responses in the baseline survey, 46 in the first follow-up survey and 374 in the second follow-up survey. Although all but six WBOs responded to these questions in the baseline survey, 54 did not respond to all of these questions in the first follow-up survey, and 117 did not respond to them in the second follow-up survey (i.e., the WBOs who were not interviewed).Household welfare is measured by an asset index, based on the household’s reported ownership (yes-no) of 20 consumer durables. The asset index is calculated as the first principal component of the available indicators, as is the usual practice (Filmer and Scott 2012). Although all but five WBOs responded to the questions on durable goods ownership in the baseline survey, while 54 did not respond to the same questions in the first follow-up survey, and 117 did not respond in the second follow-up survey (i.e., the WBOs who were not interviewed).36 laku pandai is an Indonesian acronym for “branchless banking.” 37 Instrument (2) in the baseline survey was also changed to “electronic savings account of the partner bank” in the first follow-up survey.34Annex 2. Re-transformation of estimates to their original metricsThe estimated average treatment effects, when the treatments are represented by dummy variables (as in Model 1), can be converted to approximate percentage changes from the baseline sample mean value using the following transformation (Kennedy 1981):P ≈ 100*(exp(β̂ - 0.5 Vâr(β̂)) – 1)(A2–1)where P is the percentage change estimate, β̂ is the estimated treatment effect in the IHS (or natural log) metric, and Vâr(β̂) is its estimated standard error squared.When the treatment effects are represented by linear or quadratic time trends (as in Models 2 and 3) and the dependent variable is transformed to an IHS value (profits, capital inputs) or to a natural log value (non-zero level of savings), the projected percentage changes can be obtained using the following formula:Pt=100*[(exp(xtθ̂t + 0.5*(RMSE)2)-1]/Y̅t=0 - Pt=0 (t=0, 1, … , 24)(A2–2)where t refers to month, x refers to the row vector of treatment measures in Models 2 and 3 (e.g., DA in Model 2 or both DA and DA2 in Model 3), θ̂ refers to the vector of estimated treatment effects (β, γ, δ), RMSE refers to the root mean square error, and Y̅t=0 refers to the baseline sample mean value of the outcome.3838 The formula in equation (A2–2) assumes that the projections are based on the conditional mean, rather than the conditional median. If Y has a lognormal distribution with mean μ and constant variance σ2, exp(μ) is the conditional median, while the conditional mean is equal to (exp(μ + 0.5*σ2) (Goldberger 1968).35
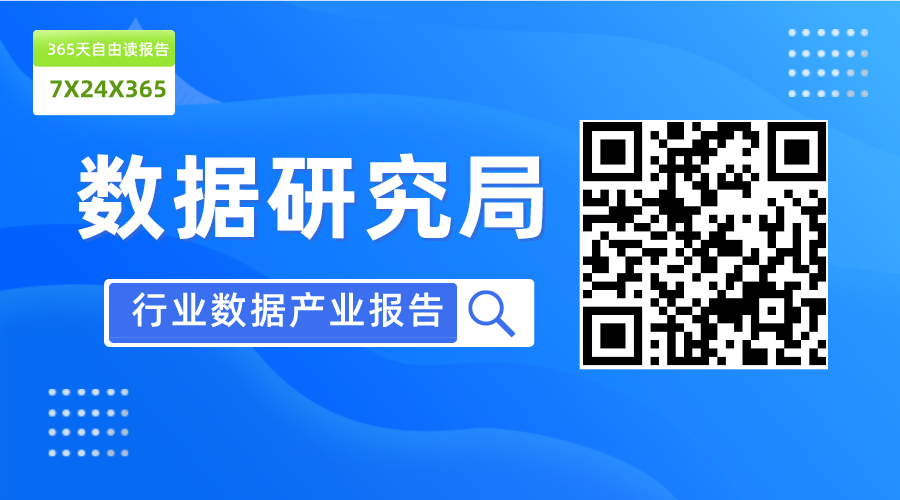
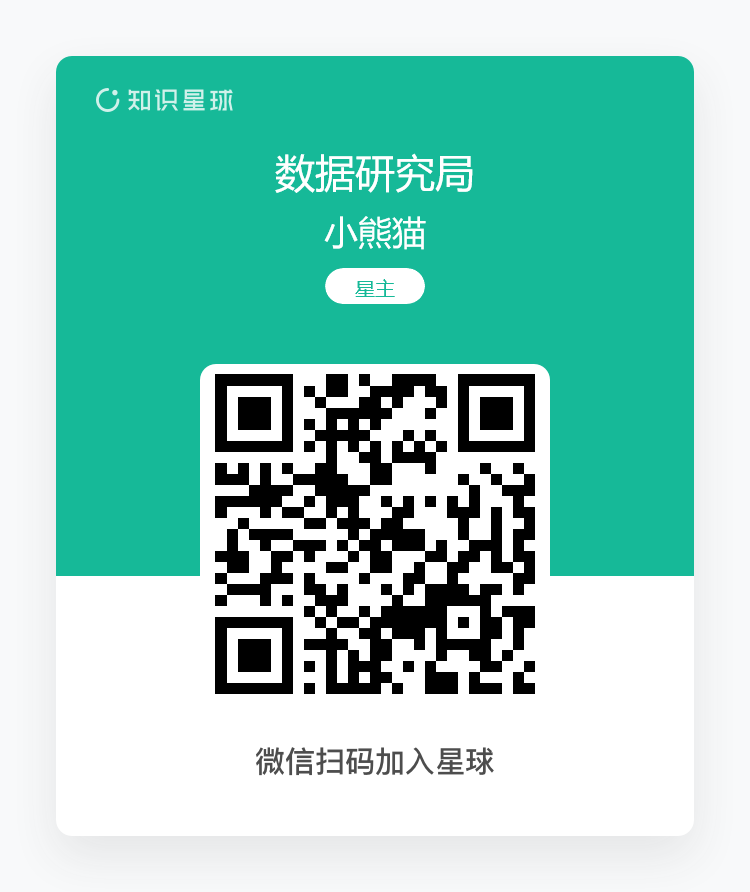