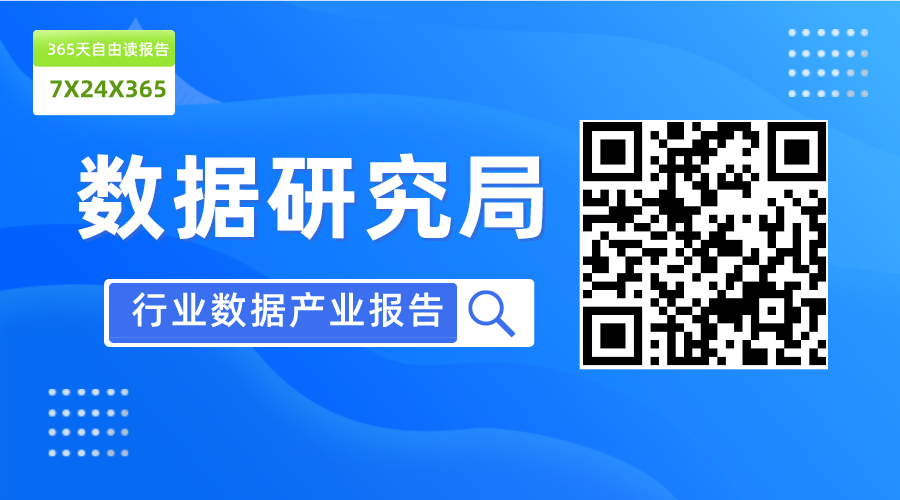
J.P.Morgan digest on risk premia strategies Summary of Q2'21 research reports on systematic investing Global Quantitative & Derivatives Strategy 20 August 2021 Global Quantitative and Derivatives Strategy Dobromir Tzotchev, PhD AC (44-20) 7134-5331 dobromir.tzotchev@jpmorgan.com J.P. Morgan Securities plc Marko Kolanovic, PhD (1-212) 622-3677 marko.kolanovic@jpmorgan.com J.P. Morgan Securities LLC Peng Cheng, CFA (1-212) 622-5036 peng.cheng@jpmorgan.com J.P. Morgan Securities LLC Meera Chandan (44-20) 7134-2924 meera.chandan@jpmorgan.com J.P. Morgan Securities plc Shivam Ghosh (44-20) 7742-4342 shivam.ghosh@jpmorgan.com J.P. Morgan Securities plc Global Cross-Asset Research Dobromir Tzotchev, PhD AC (44-20) 7134-5331 dobromir.tzotchev@jpmorgan.com J.P. Morgan Securities plc Global Cross-Asset Research Peng Cheng, CFA (1-212) 622-5036 peng.cheng@jpmorgan.com J.P. Morgan Securities LLC Global Markets Strategy Marko Kolanovic, PhD (1-212) 272-1438 marko.kolanovic@jpmorgan.com J.P. Morgan Securities LLC Global FX Strategy Meera Chandan (44-20) 7134-2924 meera.chandan@jpmorgan.com J.P. Morgan Securities plc Global Markets Strategy Thomas Salopek (1-212) 834-5476 thomas.salopek@jpmorgan.com J.P. Morgan Securities LLC European Credit Strategy Shivam Ghosh (44-20) 7742 4342 shivam.ghosh@jpmorgan.com J.P. Morgan Securities plc See page 36 for analyst certification and important disclosures, including non-US analyst disclosures. J.P. Morgan does and seeks to do business with companies covered in its research reports. As a result, investors should be aware that the firm may have a conflict of interest that could affect the objectivity of this report. Investors should consider this report as only a single factor in making their investment decision. www.jpmorganmarkets.com Dobromir Tzotchev, PhD (44-20) 7134-5331 dobromir.tzotchev@jpmorgan.com Global Quantitative & Derivatives Strategy 20 August 2021 Table of Contents J.P. Morgan Systematic Strategies Research Contacts........3 Executive Summary .................................................................4 Pure risk premia styles within the FICC universe..................5 Derivatives systematic strategies ...........................................9 Portfolio construction ............................................................16 Systematic strategies in credit and FX.................................19 Regime detection....................................................................23 Thematic investing: global outlook and key themes...........27 Systematic strategies based on retail trading activities .....28 Structural breaks in equity risk premia ................................31 List of H1'21 reports with focus on systematic investing...34 Background reports ...............................................................35 2 Dobromir Tzotchev, PhD (44-20) 7134-5331 dobromir.tzotchev@jpmorgan.com Global Quantitative & Derivatives Strategy 20 August 2021 J.P. Morgan Systematic Strategies Research Contacts Global Quantitative and Derivatives Strategy Marko Kolanovic, PhD marko.kolanovic@jpmorgan.com J.P. Morgan Securities LLC Dubravko Lakos-Bujas dubravko.lakos-bujas@jpmorgan.com J.P. Morgan Securities LLC Dobromir Tzotchev, PhD dobromir.tzotchev@jpmorgan.com J.P. Morgan Securities plc Ada Lau ada.lau@jpmorgan.com J.P. Morgan Securities (Asia Pacific) Limited/ J.P. Morgan Broking (Hong Kong) Limited Lorenzo Ravagli, PhD Lorenzo.ravagli@jpmorgan.com J.P. Morgan Securities plc Peng Cheng, CFA peng.cheng@jpmorgan.com J.P. Morgan Securities LLC Robert Smith, PhD robert.z.smith@jpmorgan.com J.P. Morgan Securities Australia Limited Berowne Hlavaty berowne.d.hlavaty@jpmorgan.com J.P. Morgan Securities Australia Limited Khuram Chaudhry khuram.chaudhry@jpmorgan.com J.P. Morgan Securities plc Ayub Hanif, PhD ayub.hanif@jpmorgan.com J.P. Morgan Securities plc William Summer william.summer@jpmorgan.com J.P. Morgan Securities plc Arun Jain arun.p.jain@jpmorgan.com J.P. Morgan Securities LLC Rahul Dalmia rahul.dalmia@jpmorgan.com J.P. Morgan Securities plc Haoshun Liu haoshun.liu@jpmorgan.com J.P. Morgan Securities (Asia Pacific) Limited/ J.P. Morgan Broking (Hong European Credit Strategy and Derivative Research Shivam Ghosh shivam.ghosh@jpmorgan.com J.P. Morgan Securities plc Matthew G Haworth matthew.g.haworth@jpmorgan.com J.P. Morgan Securities plc Saul Doctor saul.doctor@jpmorgan.com J.P. Morgan Securities plc Global FX Strategy Meera Chandan meera.chandan@jpmorgan.com J.P. Morgan Securities plc Ladislav Jankovic ladislav.jankovic@jpmorgan.com J.P. Morgan Securities LLC Arindam Sandilya arindam.x.sandilya@jpmorgan.com JPMorgan Chase Bank, N.A., Singapore Branch Juan Duran-Vara juan.duran-vara@jpmorgan.com J.P. Morgan Securities plc Global Fixed Income and US Equity Index Technical Strategy Jason Hunter Jason.x.hunter@jpmorgan.com J.P. Morgan Securities LLC Alix Tepper Floman alix.tepper.floman@jpmorgan.com J.P. Morgan Securities LLC US Fixed Income Strategy Joshua Younger Joshua.d.younger@jpmorgan.com J.P. Morgan Securities LLC Veronica Mejia Bustamante veronica.mejiabustamante@ jpmorgan.com J.P. Morgan Securities LLC EM Local Markets and Sovereign Debt Strategy George Christou george.christou@jpmorgan.com J.P. Morgan Securities plc Jonny Goulden jonathan.m.goulden@jpmorgan.com J.P. Morgan Securities plc Global Macro Strategy Thomas Salopek thomas.salopek@jpmorgan.com J.P. Morgan Securities LLC Nikolaos Panigirtzoglou nikolaos.panigirtzoglou@jpmorgan.com J.P. Morgan Securities PLC Mika Inkinen Mika.j.inkinen@jpmorgan.com J.P. Morgan Securities PLC 3 Dobromir Tzotchev, PhD (44-20) 7134-5331 dobromir.tzotchev@jpmorgan.com Global Quantitative & Derivatives Strategy 20 August 2021 Executive Summary The current report is a part of periodic series that summarizes the publications of the J.P.Morgan research department that focus on risk premia investing and systematic strategies. Below we review the relevant research notes in Q2’21 across various research groups that can be broadly classified in the following streams: Pure risk premia styles within the FICC universe Derivatives systematic strategies Portfolio construction Systematic strategies in credit and FX Regime detection Thematic investing Systematic strategies based on retail trading activities Structural breaks in equity risk premia 4 Dobromir Tzotchev, PhD (44-20) 7134-5331 dobromir.tzotchev@jpmorgan.com Global Quantitative & Derivatives Strategy 20 August 2021 Pure risk premia styles within the FICC universe Disentangling cross-sectional momentum and carry effects – application to commodities risk premia In The quest for pure equity factor exposure and Custom Performance Attribution based on Portfolio Holdings the concept of pure factors in the equities space has been introduced. The same concept is difficult to implement within the FIC universe, due to the limited number of underlying assets In Disentangling momentum and carry in commodities, structural breaks in risk premia and latest model views the framework introduced in Market-neutral carry strategies is employed to disentangle the effects of the main risk premia styles. In particular, the focus has been on the isolation of the cross-sectional momentum and carry effects with an illustration for the commodities asset class. In the commodities space there is a strong fundamentally-based relationship between the carry and the momentum that leads to an overlap and positive correlation between the typical cross-sectional carry and cross-sectional momentum strategies First, the standard implementations of the cross-sectional carry and momentum strategies have been done. In the case of the cross-sectional carry strategy first the assets are ranked by the carry/vol ratio and then we go long top quantile and short bottom quantile with the asset weight being proportional to the inverse of the asset’s volatility. At the end the portfolio is geared to a 10% annualized vol target. The standard cross-sectional momentum strategy relies on the trend-following signal introduced in Designing robust trend-following system. In a similar way the assets are ranked by the trend-following signals and we go long the top quantile and we are short the bottom quantile with the asset weight being proportional to the inverse of the asset’s volatility. The annualized portfolio volatility is similarly targeted to be 10%. Next, the pure versions of the cross-sectional carry and momentum strategies have been put forward. The market-neutral carry strategy was introduced in market-neutral carry strategies. The same concept has been leveraged to construct the market-neutral cross-sectional momentum strategy. The market-neutral cross-sectional momentum strategy has also been designed to eliminate the main risk factors within a given asset class and to provide a return stream driven by what we refer to as the asset-specific trends – those are the idiosyncratic average returns that are not attributable to the assets exposures to the factors. During an optimization the portfolio exposure to the asset specific trends is optimized with neutrality to the main factors and in addition a neutral (zero) portfolio carry income. 5 Dobromir Tzotchev, PhD (44-20) 7134-5331 dobromir.tzotchev@jpmorgan.com Global Quantitative & Derivatives Strategy 20 August 2021 Figure 1: Cumulative performance of the market-neutral carry and standard carry strategy Table 1: Performance statistics Market-neutral carry Ann Return 10.1% Ann Volatility 8.9% Sharpe 1.13 Max DD 18.8% Skewness 0.05 Kurtosis 1.72 Source: J.P. Morgan Quantitative and Derivatives Strategy Standard carry 10.2% 10.2% 1.01 24.1% -0.10 0.73 Source: J.P. Morgan Quantitative and Derivatives Strategy Figure 2: Cumulative performance of the market-neutral crosssectional momentum and standard cross-sectional momentum Table 2: Performance statistics Market-neutral cross- Standard cross- sectional momentum sectional momentum Ann Return 2.5% 4.7% Ann Volatility 7.5% 10.0% Sharpe 0.33 0.47 Max DD 31% 39% Skewness -0.04 -0.15 Kurtosis 1.20 1.00 Source: J.P. Morgan Quantitative and Derivatives Strategy Source: J.P. Morgan Quantitative and Derivatives Strategy There is no substantial difference in the performances of the market-neutral carry strategy and standard carry strategy with the market-neutral carry strategy demonstrating a more consistent performance in the recent years. Turning to the cross-sectional momentum strategy, the standard implementation has overall better performance. The standard carry and cross-sectional momentum strategies are highly correlated. In contrast the market-neutral implementations of the carry and the momentum strategies have a negligible correlation. Next, an equally-weighted (in terms of vol budget) portfolio of the carry and momentum styles has been constructed. Despite the inferior performance of the market-neutral cross-sectional system in comparison to the standard one, the combination of the market-neutral styles is performing slightly better than the combination of the standard styles because of the lower correlation. The lower and more stable correlation among the market-neutral styles allows for prudent risk allocation and well-defined performance attribution. 6 Dobromir Tzotchev, PhD (44-20) 7134-5331 dobromir.tzotchev@jpmorgan.com Global Quantitative & Derivatives Strategy 20 August 2021 Figure 3: Cumulative performance of the combination of carry and momentum under the standard and market-neutral implementations Table 3: Performance statistics Combination of Combination of market- standard carry and neutral carry and cross- cross-sectional sectional momentum momentum Ann Return 5.9% 7.0% Ann Volatility 6.4% 8.4% Sharpe 0.91 0.83 Max DD 15.3% 15.0% Skewness -0.01 -0.12 Kurtosis 1.41 0.94 Source: J.P. Morgan Quantitative and Derivatives Strategy Source: J.P. Morgan Quantitative and Derivatives Strategy Trend-Following in Factors In Risk premia performance review, trend-following in factors and latest model views the effort to disentangle various risk premia styles within the FICC universe and to provide a pure factor implementation has been continued. A trend-following in factors approach has been proposed as an alternative to the traditional trendfollowing implementation as introduced in Designing robust trend-following system in which the trend-following signal is based on the individual asset. The trend-following in factors approach is as follows: The returns of Principal Components over the lookback period are reconstructed. The number of components using the Empirical Kaiser Criterion (Braeken, 2017). 1 The returns of the Principal Components are calculated as: where asset. _ =∑ _ ∗ ,, , is the jth element in ith eigenvector scaled by the volatility of the jth Trend-following signal ( _ ) are calculated for each _ using methodology described in Designing robust trend-following system. A prudent decision reflecting the importance of the individual factors is to allocate a risk budget based on the fraction of the variance explained. We know that the fraction of the variance explained by the ith factor is proportional to the eigenvalue ( ) associated with the ith factor. Furthermore, for uncorrelated assets the position in an asset i is proportional to the ratio of / , where is the respective risk budget and is the volatility of asset i .2 Therefore, abstracting from the magnitude of the trend-following signal (or assuming an equal signal) we allocate equally among the principal components. 1 Note that in market-neutral carry strategies we prefer to err on the side of caution, i.e. overhedge if necessary, and that is why the standard Kaiser Criterion was applied. 2 See for example Managing Risk Exposures using the Risk Budgeting Approach 7 Dobromir Tzotchev, PhD (44-20) 7134-5331 dobromir.tzotchev@jpmorgan.com Global Quantitative & Derivatives Strategy 20 August 2021 Subsequently, the position in asset j is: = _∗ , The annualized portfolio volatility is targeted to be 10% on an expanded window lookback. The asset-based trend-following system presented in Designing robust trendfollowing system has slightly outperformed the trend-following in factors system over the backtest period. The trend-following in factors strategy has negligible correlations with the market-neutral implementations of carry and cross-sectional momentum, which facilitates risk allocation and performance analysis. Figure 4: Cumulative performance of trend-following in factors and asset-based trend-following Table 4: Performance statistics Ann Return Ann Volatility Sharpe Max DD Skewness Kurtosis Trend-following in Factors 4.4% 8.8% 0.50 23.5% -0.19 6.37 Source: J.P. Morgan Quantitative and Derivatives Strategy Asset-based trendfollowing 6.4% 9.7% 0.65 27.0% -0.14 5.65 Source: J.P. Morgan Quantitative and Derivatives Strategy Nevertheless the merits of the trend-following in factors approach shall not be solely judged on the performance metrics. The correlations of the trend-following in factors with the market-neutral carry strategy and the market-neutral cross-sectional momentum strategy are quite contained. As a consequence, the allocation decisions and the performance attribution are much more straightforward in the case of the pure risk premia strategies. Table 5: Correlation matrix Trend-following in Factors Market neutral Carry Market-neutral cross-sectional momentum Asset-based trend-following Standard cross-sectional momentum Standard carry strategy Trend-following in Factors 1.00 0.00 0.16 0.54 0.40 0.08 Source: J.P. Morgan Quantitative and Derivatives Strategy Market neutral Carry 0.00 1.00 0.06 0.09 0.27 0.57 Market-neutral crosssectional momentum 0.16 0.06 1.00 0.18 0.44 0.08 Asset-based trend-following 0.54 0.09 0.18 1.00 0.47 0.26 Standard cross- sectional momentum 0.40 0.27 0.44 0.47 1.00 0.39 Standard carry strategy 0.08 0.57 0.08 0.26 0.39 1.00 Furthermore, two versions of optimized implementations of the trend-following in factors approach have been proposed. The first implementation minimizes the cost of the strategy. The second optimization maximizes the net expected return of the strategy. The cost minimization approach achieves sizable cost savings but the 8 Dobromir Tzotchev, PhD (44-20) 7134-5331 dobromir.tzotchev@jpmorgan.com Global Quantitative & Derivatives Strategy 20 August 2021 overall Sharpe ratio is barely changed. The net-trend return optimization provides an improvement in the Sharpe ratio with a slightly better control on costs. Figure 5: Cumulative performance of the trend-following in actors and optimized trend-following in factors approaches Table 6: Performance statistics Ann Return Ann Volatility Sharpe Max DD Skewness Kurtosis Optimized TF in factors (cost minimization) 3.8% 7.8% 0.48 27.8% -0.17 8.91 TF in Factors 4.4% 8.8% 0.50 23.5% -0.19 6.38 Source: J.P. Morgan Quantitative and Derivatives Strategy Optimized TF in factors (net trend- return maximization) 5.5% 9.4% 0.58 28.0% -0.28 5.69 Source: J.P. Morgan Quantitative and Derivatives Strategy The cost minimization approach achieves sizable cost savings but the overall Sharpe ratio is barely changed. The net-trend return optimization provides an improvement in the Sharpe ratio with a slightly better control on costs. Derivatives systematic strategies Optimal Variance Replicating Portfolio Traditionally, to replicate a variance swap, the trader holds a static portfolio of out of the money options, weighted by the inverse of their squared strike prices. = 2Δ =⋅ ( )+ w () In the equations above, denotes the weights of the option with strike K, and ( ) and ( ) are the prices of puts and calls at forward F and strike K, respectively. Δ is the distance from the previous strike and finally, is the time to maturity. While such a weighting scheme represents the market risk neutral expectations of future volatility, the resulting implied variance is also well known to be a biased estimator of realized volatility. In other words, there may be better weightings that allow investors to more effectively predict future realized volatility and monetize the volatility risk premium. In the report Optimal Variance Replicating Portfolio we examine a simple method, 9 Dobromir Tzotchev, PhD (44-20) 7134-5331 dobromir.tzotchev@jpmorgan.com Global Quantitative & Derivatives Strategy 20 August 2021 adapted from Carr, Wu, and Zhang (2020) 3, to construct optimal variance swap replicating portfolios that achieve these goals. We run the following regression: = ( )+ β () Since the estimates are a linear combination of call and put prices, the from this regression can be used as adjustments to to form a static portfolio of options. The empirical results show that across indices and maturities, the options between 90 - 100% strikes have the lowest weights (are rich), and OTM strikes, especially on the call wing, receive the most weights (are cheap). Remarkably, we do not find systematic richness in the left tails. This seems to suggest much of the volatility premium comes from slightly out of the money put strikes. We demonstrate that when applied to S&P 500 and Euro STOXX 50, the proposed portfolio constructions: 1) better predict future realized volatility than the implied variance levels, and 2) result in more profitable volatility strategies. Table 7: Performance statistics of short variance swap strategies P&L (Vega) Mean St Dev Sharpe Skew Vanilla 2006 - Present 2010 - 2019 1.6 3.1 16.2 7.7 0.2 0.8 -5.1 -6.2 Source: J.P. Morgan 90 - 100% Corridor 2006 - Present 2010 - 2019 2.0 2.5 0.7 5.7 0.6 0.9 -1.7 -1.8 105% Up 2006 - Present 2010 - 2019 0.3 0.0 4.0 3.7 0.1 0.0 -2.2 -3.4 Figure 6: Shorting SPX 3M vanilla vs. 90-100% corridor var swap Vega P&L 0 -50 -100 Source: J.P. Morgan 2010 Corridor Vanilla 2015 2020 3 Carr, Peter, Liuren Wu, Zhibai Zhang, 2020 Using Machine Learning to Predict Realized Variance, Journal Of Investment Management, Vol. 18, No. 2 (2020) pp 1 – 16 10 Dobromir Tzotchev, PhD (44-20) 7134-5331 dobromir.tzotchev@jpmorgan.com Global Quantitative & Derivatives Strategy 20 August 2021 Figure 7: Shorting SPX 3M vanilla vs. 105% up var Vega P&L 0 -50 -100 Source: J.P. Morgan 2010 Corridor Vanilla 2015 2020 More on FX Vol Convexity Smile Strategies An extensive recent report on cross-asset vol-of-vol strategies found as main conclusion the observation that OTM options might be statistically overvalued. In there, plain vanillas and exotics constructs across markets were investigated, with the purpose of drawing general conclusions rather than market-specific features, like sensitivities to pricing parameters. A shorter follow-up focused on a few aspects that did not find room in the “bigpicture” report above. Having assessed the presence of a premium on OTM options, the natural question which follows is: OK, but how does that premium vary with the choice of the option’s strike? Figure 8 (LHS) takes EUR/USD, 1m short delta-hedged strangles as a case study and considers the sensitivity of the resulting long-term Sharpe ratio to the actual strike (or Delta). The Sharpe ratio roughly doubles by moving from ATM to 15D strikes, and starts to decline for further OTM strikes, in particular 5D ones. Similar conclusions can be drawn for other G10 volatilities. It is confirmed how ATM vols offer typically the best entry point for long-vol trades; conversely, 15D strangles are associated with the best ex-post performance measures for harvesting vol premium purposes. Figure 8: For 1m EUR/USD short-Gamma, highest Sharpe is found for 15delta strangle. Short strangle hedged with long 5delta strangle, in 2:+1 Vegas, for EUR/USD 1m gives decent long term statistics. 0.9 Strike sensitivity of EUR/USD 1m short-strangle 0.8 0.7 Short far strike RV strangle (with cost) 1.4 1.2 1.0 Short near strike RV strangles (zero cost) Cumulative PnL (%) Sharpe ratio 0.6 0.8 0.6 0.5 0.4 0.4 0.2 Jan-04 Jan-05 Jan-06 Jan-07 Jan-08 Jan-09 Jan-10 Jan-11 Jan-12 Jan-13 Jan-14 Jan-15 Jan-16 Jan-17 Jan-18 Jan-19 Jan-20 Jan-21 0.0 0.3 ATM 45 40 35 30 25 20 15 10 5 -0.2 Delta for strangle Source: J.P. Morgan Quantitative and Derivatives Strategy 11 Dobromir Tzotchev, PhD (44-20) 7134-5331 dobromir.tzotchev@jpmorgan.com Global Quantitative & Derivatives Strategy 20 August 2021 The next (more ambitious) step in terms of reasoning considers the possibility of trading RV strangle constructs based on the conclusions above. Long straddle / short strangle was the simplest plain vanilla structure allowing the capture an exposure to the aforementioned vol-of-vol premium. Replacing the ATM with a 5D leg is conceptually interesting, offering an expected higher protection in case of “fat-tailed” vol events. Adjusting the relative scaling between the two legs proves however a crucial input towards the success of the RV strategy. The case study of the -15D/+5D strangle RV construct for 1M EUR/USD, in -2:+1 Vega notionals, leverages on the features discussed above, allowing to capture vol premium while limiting drawdowns. Similar conclusions apply for other volatilities, although we stress that 5D and generally deep OTM options are less liquid that closer to the money options: therefore, the possibility of trading the structure will depend on market liquidity and positioning. Double No-Touches Go Quantum, the First Look In Double no-touches go quantum, the first look the feasibility of developing a quantum SVM trading model has been explored. DNTs are a natural fit for an SVM type of problem with their binary outcome and while a classical SVM should suffice, prodded by an occasional interest by more adventurous clients into quantum machine learning we test drive making a model on a quantum simulator. The appeal of DNTs is in their defined downside. Holder of a DNT pays a premium to be short vol in leveraged format. Market price represents theoretical probability that the structure would indeed survive till expiry and pay the holder the full notional. The barriers are continuous, thus exposed to noise and intraday spot jitter. Quantum kernel is just an exotic kernel that maps our classical features into a higher dimension space (just as the classical basis function kernel does) where it may be easier to train SVM to separate positive and negative DNT outcomes. While kernels come from a quantum distribution the optimization is same as for the classical SVM. Figure 9: Linearly inseparable data for 3M AUD/USD 20% TV. QSVM models largely able to compensate for downturns. Categorical y variable -1 = barrier hit, i.e.-TV payout, and 1 = DNT survived till expiry, i.e. 100-TV payout. Input variables: implied / realized ratio (noise level variable) and 14day ADX indicator (centered at 22.5) – drift variable. Input features are scaled and centered via standard scaler. Quantum kernel – 2nd order Pauli-Z circuit with depth 1 and 2. 256 shots. Cum P/L (mio) training testing long always 100 Depth = 1 training Depth = 2 test 75 Depth = 1 test 50 25 0 -25 -50 Dec 10 Apr 12 Sep 13 Jan 15 Jun 16 Oct 17 Mar 19 Jul 20 Source: J.P. Morgan 12 Dobromir Tzotchev, PhD (44-20) 7134-5331 dobromir.tzotchev@jpmorgan.com Global Quantitative & Derivatives Strategy 20 August 2021 The results demonstrate value in using quantum kernels in FX trading, but the speed of computation on a quantum simulator remains an obstacle for refining and testing robustness of the models. With the encouraging start the next step would be a further refinement, bigger pull of features, and kernels beyond the second-order Pauli-Z, as well as testing QSVM on a broader pool of currency pairs to assess the robustness. Finally, while we have seen interest in the broader quantum ML topic from some clients, for now we remain of opinion that QSVM is just another SVM kernel, and still a bit of an academic exercise. Systematically monetize bond/equity correlation premium via vanilla options In the report Cross Asset Volatility What can option implied skew tell us about bond equity correlation?, it was discussed how to obtain implied correlation and beta between US equities and Treasuries using listed options on the SPY and TLT ETFs. = = Additionally, it was observed that implied correlation and beta tend to trade at a premium to the subsequent realized measures. Figure 10: TLT/SPY realized beta vs. 1-month moving average of implied beta 1-month beta of TLT daily returns with respect to SPY 0 -1 Implied Realized 2012 2014 2016 2018 2020 Source: J.P. Morgan In the report Systematically monetize bond/equity correlation premium via vanilla options a simple option strategy between SPY and TLT is proposed to take advantage of the mispricing. In effect, we are going long the cheaper protection (TLT calls) and short the richer protection (SPY puts), assuming the correlation stays negative. In Figure 11 below we show the P&L of a systematic implementation where we sell 1x 1M 25 delta SPY puts to buy multiple 1M 25 delta TLT calls, premium neutral. The Sharpe ratio of the strategy is around 0.78 annualized. Additionally, the payoffs do not exhibit a negative skewness, unlike other short correlation strategies. 13 Dobromir Tzotchev, PhD (44-20) 7134-5331 dobromir.tzotchev@jpmorgan.com Global Quantitative & Derivatives Strategy 20 August 2021 Figure 11: Terminal P&L of the TLT/SPY 1M option switch strategy P&L as % of SPY notional 20 10 0 -10 -20 2012 2014 2016 2018 Source: J.P. Morgan 2020 The strategy involves only vanilla options of the two ETFs and is effective in exploiting the implied to realized premium. The strategy is profitable but occasionally shows sharp drawdowns, which coincide with periods of positive bond/equity correlation. An enhancement to the strategy by overlaying a high frequency correlation forecast as a timing signal has been proposed. The model forecasts the one week ahead realized correlation, using intraday prices measured at 15 minute intervals and the details of the model can be found in the Appendix of the paper. Synthetic defensive long vol strategy In the publication Quarterly review, Credit value, Synthetic defensive long vol and latest model views, the suite of defensive strategies introduced in ‘Defensive Risk Premia: Systematic Strategies for the Risk-Off Times’ has been augmented by а long vol synthetic defensive basket that invests in various maturities along the VIX futures curve. The methodology behind the synthetic defensive baskets is based on our market-neutral carry strategy (Market-Neutral Carry Strategies). The strategy relies on opportunistic capture of both carry and momentum income opportunities which facilitate the maintenance of a hedging position in the desired direction. In the case of the synthetic defensive vol strategy we aim to obtain a long exposure to volatility coupled with advantageous carry and momentum properties via a long/short basket invested in different maturities VIX futures. Long volatility positions are typically associated with negative carry and the synthetic defensive basket investing in various maturities VIX futures can alleviate this problem by being both long carry and having a positive exposure to momentum. The long vol synthetic position is constructed as a basket of long/short positions in the first 8 contracts on the VIX futures curve and the lookback periods are 1Y and 2Y. The synthetic defensive strategy strongly outperforms the long vol benchmark that takes long positions in the same futures with position sizes being determined via a risk parity approach4.The synthetic defensive vol strategy has also strongly 4 The volatility of the benchmark is set to the average volatility of the constituents. 14 Dobromir Tzotchev, PhD (44-20) 7134-5331 dobromir.tzotchev@jpmorgan.com Global Quantitative & Derivatives Strategy 20 August 2021 outperformed the benchmark during the GFC and the subsequent rebound market rebound in 2009. The performance in March 2020 has been similar but subsequently synthetic defensive vol strategy has given up the gains more quickly. Nevertheless, the overall performance in 2020/2021 has been quite similar. Figure 12: Cumulative performance of the synthetic defensive long vol strategy and long vol risk parity benchmark Table 8: Performance statistics Synthetic Defensive Long Vol Strategy Ann Return 21.2% Ann Volatility 41.1% Sharpe 0.52 Max DD5 75.6% Skewness 1.43 Kurtosis 23.32 Source: J.P. Morgan Quantitative and Derivatives Strategy Long Vol Risk Parity Benchmark -12.9% 41.1% -0.31 98.4% 2.06 22.49 Source: J.P. Morgan Quantitative and Derivatives Strategy The synthetic defensive long vol strategy brings strong diversification to a short vol benchmark and also to a long position in a typical risk-on asset as S&P500. In both the cases the combined Sharpe ratio and the drawdowns are substantially improved. Therefore, synthetic defensive long vol strategy can be used as another tool in our toolbox of defensive strategies. Figure 13: Backtest of the synthetic defensive long vol strategy– diversification results with a short vol risk parity benchmark Figure 14: Backtest of the synthetic defensive long vol strategy– diversification results with SPX Source: J.P. Morgan Quantitative and Derivatives Strategy Source: J.P. Morgan Quantitative and Derivatives Strategy 5 Please note that the drawdowns are calculated based on the compounded returns. 15 Dobromir Tzotchev, PhD (44-20) 7134-5331 dobromir.tzotchev@jpmorgan.com Global Quantitative & Derivatives Strategy 20 August 2021 Table 9: Performance statistics Ann Return sAnn Volatility Sharpe Max DD Skewness Kurtosis Synthetic Defensive Long Vol Strategy 21.2% 41.1% 0.52 75.6% 1.43 23.32 Short Vol Risk Parity Benchmark 11.5% 41.1% 0.28 76.9% -2.07 22.59 Source: J.P. Morgan Quantitative and Derivatives Strategy Combination of Synthetic Defensive Long Vol Strategy and Short Vol Risk Parity Benchmark 32.7% 38.9% 0.84 52.5% -0.94 11.36 Synthetic Defensive Long Vol Strategy 10.8% 21.0% 0.52 50.0% 1.43 23.32 SPX Index 10.2% 21.0% 0.49 52.6% -0.26 12.78 Combination of Synthetic Defensive Long Vol Strategy and SPX Index 21.0% 20.9% 1.01 36.7% -0.65 14.38 Portfolio construction What can option implied skew tell us about bond equity correlation? For much of the last two decades, the correlation between bond and equity returns has been negative. The assumption that the correlation will stay low or negative forms an important foundation for popular asset allocation strategies such as the 60:40 and risk parity. However, we observe significant variations in short term correlation over time and sharp spikes are common. Figure 15: Rolling 3M correlation between SPY and TLT using daily returns 0.0 -0.5 Source: J.P. Morgan 2010 2015 2020 Therefore, there is value in having a bond/equity correlation forecast that is high frequency and forward looking. In the report What can option implied skew tell us about bond equity correlation? such a measure is proposed, based on information extracted from exchange listed options of the SPY (S&P 500 ETF) and TLT (20Y+ US Treasuries ETF). The SPY return distribution is almost always left (negative) skewed, indicating that the put wing is overbid, and investors associate SPY downside with the systemic downside risk. On the other hand, the skewness of the TLT oscillates between positive and negative. When the TLT implied skewness is negative, it means that investors overbid the put wing and associate TLT downside with the systemic downside risk. In turn, this implies a positive correlation between TLT and SPY. When the TLT implied skewness turns positive, it indicates the investors see TLT upside representing the systemic downside risk, implying a negative correlation between TLT and SPY. 16 Dobromir Tzotchev, PhD (44-20) 7134-5331 dobromir.tzotchev@jpmorgan.com Global Quantitative & Derivatives Strategy 20 August 2021 The relationship between skew and correlation holds if the sole driver of skew is the systemic factor. Following the work of Chang et al. (2012)6 it can be shown that the implied correlation is: = Many asset allocation strategies rely on implicit or explicit forecasts of the bond/equity correlation and the whole covariance matrix. Together with the option implied variance of the assets the entire covariance matrix with option implied information can be populated. While in a two asset portfolio, the risk parity weights in risk parity and the ERC portfolios are identical7 and function of volatilities, the correlation information is still required for volatility targeting purposes. Below portfolios formed using the 1M implied covariance matrix (i.e. the risk neutral measure) and the 1M realized covariance matrix (the physical measure) are compared. The portfolios are rebalanced monthly, and the results are presented after transaction cost. When adjusted for volatility, the portfolio formed by Risk Neutral parameters outperforms, without exhibiting excess tail risk. Table 10: Summary statistics of risk parity portfolios Return % Volatility % Information Ratio Worst Drawdown % Skewness Source: J.P. Morgan Physical 13.0 12.5 1.0 -18.8 -0.7 Risk Neutral 8.5 7.2 1.2 -14.2 -0.7 Although we target our portfolio volatility to be 10%, the ex-post volatility of the Risk Neutral portfolio is much lower. This is to be expected, since the volatility and correlation premium leads to a more conservative portfolio construction. On the other hand, the realized covariance matrix produces a higher volatility portfolio, indicating that backward looking measures tend to understate the portfolio risk. Risk premia portfolio construction updates Previously the cluster portfolios and tail risk parity were introduced as alternatives to the risk parity benchmark. In Enhancing active ETF performance, cluster portfolio and tail risk parity updates, and latest model views the performances YTD of the two approaches have been updated. Figure 16shows the performances of risk premia portfolios based on various construction methodologies, all targeted to 5% vol. Both the cluster portfolio and the tail risk parity had smaller drawdowns than the risk parity portfolio in March’21. In 2021, the cluster portfolio has delivered higher risk-adjusted returns than the risk parity benchmark. Returns of the tail risk parity portfolio is lower than risk parity in 2021, but so does its expected tail risk and downside deviation. 6 Chang, Bo Young, Peter Christoffersen, Kris Jacobs and Gregory Vainberg, April 2012, Option-Implied Measures of Equity Risk, Review of Finance, Volume 16, Issue 2 7 On the properties of Equally-weighted Risk Contribution portfolios, Maillard, Roncalli, and Teiletche, working paper, 2008 17 Dobromir Tzotchev, PhD (44-20) 7134-5331 dobromir.tzotchev@jpmorgan.com Global Quantitative & Derivatives Strategy 20 August 2021 Figure 16: Performance of cluster based portfolio and tail risk parity portfolio compared with risk parity benchmark in 2021 2021 Cum. Vol t-stat Skew Returns Cluster portfolio 6.8% 5.5% 2.03 -0.78 Risk Parity 3.7% 5.0% 1.23 -1.20 HRP 0.6% 5.2% 0.20 -0.97 Source: J.P. Morgan Quantitative and Derivatives Strategy Max Kurtosis Drawdown 0.95 1.9% 4.30 2.5% 4.01 2.7% 2021 Cum. Returns Risk Parity 4.1% Tail Risk Parity 2.5% Vol 5.1% 4.1% Skew -1.20 -0.55 Kurtosis 4.13 2.95 Max DD 2.6% 1.6% Modified Downside CVaR deviation 1.0% 3.7% 0.7% 2.8% Balancing factor portfolios to enhance thematic alpha Risk premia strategies can be used to create a balancing portfolio that complements an active ETF. The overall portfolio is supposed to capture the desired alpha on top of a set of balanced risk parity factor exposures, and is expected to have better riskadjusted returns over the long run. In Enhancing active ETF performance, cluster portfolio and tail risk parity updates, and latest model views a sizable Tech theme ETF has been analyzed and it has been investigate if one could mitigate the swings in performance due to its large Momentum exposures, and harvest the stock-selection alpha in the portfolio. The Tech theme ETF portfolio is decomposed as follows: = + ×( ) where is the Tech theme ETF exposure to a particular pure equity factor j. Instead of the original factor exposures, a balanced allocation with risk parity weights on each factor is preferred. To do so, we can create a balancing portfolio as a linear combination of 5 investable ERP indices, and complement it to the original Tech theme ETF portfolio. By design, we retain the same alpha which corresponds to specific risk, and ensure equal risk contributions from each of the 5 factors. = − ×( ) so that = + + ( ) =+ 18 Dobromir Tzotchev, PhD (44-20) 7134-5331 dobromir.tzotchev@jpmorgan.com Global Quantitative & Derivatives Strategy 20 August 2021 The original factor portfolio (implicitly held in the ETF) suffered from big swings correlated to Momentum's performance, as shown in the latest drop around March. On the other hand, the balancing portfolio is designed to offset such factor volatilities, and its performance is in general negatively correlated to the original factor portfolio. Figure 17: Performance of the Tech-theme ETF portfolio with/without the factor balancing portfolio since 2018 (left) and since 2021 (right) Systematic strategies in credit and FX Value investing in corporate bonds In the April’s Quantitative Perspectives on Cross-Asset Risk Premia, we focus on value factors in credit and the performance of credit value has been compared with its equity counterpart. The credit Value signal is based on the relative cheapness of an issuer's spread compared to its peer universe. The peer universe is defined to be a cohort of issuers with similar duration and rating profile as the issuer. Every month a cross-sectional regression of the form is carried out: ,= + ,+ Ι, + Ι, where , is the senior spread of issuer i at time t and , is its effective duration. The last two terms are dummy variables to control for the cross-sectional dependence of spreads on ratings and sectors. The summation variable “rat” takes values A, BBB, BB, B and “sec” goes across 18 granular sectors like Energy, Financials, Autos, etc. The regression creates a framework for assigning a fair value spread , given an issuer’s duration, rating and sector. The Value signal is simply the residual from the above regression: , = log ,/ , Issuers with the highest (lowest) value scores enter the top (bottom) quintile Value basket. Over the past 20 years, value has delivered positive alpha in both credit and equity markets. While both equity and credit value performed very well before 2008, credit value delivers more consistent returns than equity value over the long run with a Sharpe ratio close to 1.0. Both credit and equity value are sensitive to the economic 19 Dobromir Tzotchev, PhD (44-20) 7134-5331 dobromir.tzotchev@jpmorgan.com Global Quantitative & Derivatives Strategy 20 August 2021 cycles, and suffer from large drawdowns during the GFC in 2008 as well as the COVID sell-off in 2020. However, credit value rebounds from the troughs about 3-6 months earlier than equity value Figure 18: Equity value and credit value performance (long over market) since 2000 Credit value rebounds earlier than equity value after the GFC in 2008 as well as after COVID sell-off in 2020. Source: J.P. Morgan Quantitative and Derivatives Strategy Since 2000 Ann.Returns Ann.Vol Sharpe ratio Long over Market Credit Value Equity Value 1.7% 1.8% 0.98 2.6% 6.5% 0.40 Furthermore, the difference between sector compositions in credit value and equity value has been analyzed with a focus on the past 12 months only. A Brinson attribution analysis has been run to highlight the allocation and selection effects across sectors. The allocation effect shows whether the value portfolio has benefited from an OW or UW of a sector. The selection effect shows the stock or bond picking ability within a certain sector. The sum of the allocation and the selection effects equals the excess return of the Value portfolios relative to the market. Figure 19 highlights the key differences we observe across credit value and equity value in the recent 12 months following COVID sell-off. Figure 19: Differences in sector contribution between credit value and equity value Credit Value Equity Value Overall comment Positive selection effects across all sectors, especially within Energy Selection effect is weaker, and especially poor within the Technology sector Energy UW the market, but very strong bond selection power within the sector, leading to positive performance Slightly negative performance due to both allocation (small UW) and selection effect Large OW leads to outperformance. Financials Selection effect is also good Technology Unlike equity value, credit value OW technology. Also there is positive selection effect within the sector Source: J.P. Morgan Quantitative and Derivatives Strategy Positive selection effect, but recent UW leads to negative performance Poor stock selection within sector. Large UW also leads to negative performance Credit risk premia performance in 2Q and sectoral tilts in the Value basket In Factor Tracker: 2Q performance and H2 Outlook the strong performance of the cyclically positioned multi-factor Integrated and Momentum credit risk premia styles has been highlighted. Both factors were over-weight higher beta and inflation 20 Dobromir Tzotchev, PhD (44-20) 7134-5331 dobromir.tzotchev@jpmorgan.com Global Quantitative & Derivatives Strategy 20 August 2021 sensitive sectors like Energy, Autos, and Industrials and benefited from rising inflation and the cyclicals led rally in credit markets. Along with Value, all three factors have outperformed the long-only market in 2021 and since January 2020, over a complete market crash and recovery cycle, Figure 20 and Figure 21. Credit Value, specifically, has had an impressive streak of outperformance over the last year and a half. The sectors where Value continues to find opportunities in the current environment of tight spreads and low dispersion are the sectors where spreads are trading cheap relative to net leverage (a measure of fundamental risk). Table 11 shows that within Euro Investment Grade (IG), Value is OW Autos, Industrials – sectors with low leverage and UW Real Estate and Telcos, sectors with comparatively higher leverage levels. Similarly, in US IG, Value is OW Autos, Transport and UW Utility and Real Estate. We are constructive on credit factors going into H2 and expect continued outperformance from the Momentum and Integrated styles. Figure 20: Credit Factor long-only cumulative returns vs the market Credit factor returns are for a composite of 40% USIG, 40% Euro IG and 20% USHY. Gross of costs with monthly rebalancing. 8% Market Value Momentum 3% Low Risk Integrated -2% -7% -12% Jan-20 Apr-20 Source: J.P. Morgan. Jul-20 Oct-20 Jan-21 Apr-21 Figure 21: Long-only factor Sharpes January 2020 – June 2021 Calculated from monthly credit excess returns gross of costs 0.45 0.41 0.41 0.40 0.35 0.35 0.30 0.25 0.20 0.16 0.14 0.15 0.10 0.05 0.00 Integrated Value Momentum Market Low Risk Source: J.P. Morgan. 21 Dobromir Tzotchev, PhD (44-20) 7134-5331 dobromir.tzotchev@jpmorgan.com Global Quantitative & Derivatives Strategy 20 August 2021 Table 11: Value factor is OW sectors with high spread to leverage ratio Spreads are as of June 2021. Leverage metric is Net Debt/EBITDA as of 1Q 2021 Sector Automotive Basic Industry Capital Goods Technology & Electronics Transportation Retail Media Utility Healthcare Services Consumer Goods Energy Telecommunications Real Estate EuroIG weight 4% 1% 1% 0% 0% 0% 0% 0% 0% -1% -1% -1% -2% -9% EuroIG Spread 48 57 48 42 65 52 63 58 41 50 50 62 49 89 EuroIG Lev 0.9 2.1 2.0 2.1 3.6 3.0 1.4 3.6 2.3 4.8 2.8 2.3 2.6 11.2 EuroIG Sprd/Lev 53 28 24 20 18 17 45 16 18 10 18 27 19 8 Source: J.P. Morgan. USIG weight 3% -3% 2% 2% 1% 0% -1% -2% 2% -4% 2% 2% 0% -5% USIG Spread 64 125 82 77 137 102 105 140 105 98 86 136 113 107 USIG Lev 1.0 2.6 2.0 1.7 2.1 2.2 2.3 5.3 2.3 2.0 2.2 2.9 2.7 7.0 USIG Sprd/Lev 62 48 41 46 64 47 45 26 46 48 40 47 42 15 FX Macro Quant Update: A neutralization across most signals, low yields and little value The growth signals based on economists’ forecasts have been unchanged for more than a quarter and hence the model has completely neutralized its pro-cyclical/ USDbearish position. This is reflected in the cross-sectional TEAM model which is now neutral USD (from bearish) for the first time in a year as well as in our time-series suite of models for G10, which have reduced exposure as very few signals are currently being triggered; a fresh set of catalysts is required for new direction for FX. Most activity data are still beating expectations and EASIs are still positive in most countries, so the stabilizaiton on growth forecast does yet not reflect data rolling over. Looking at the first half of the year, FX carry has eked out positive returns largely from EM (in contrast to last year); returns from growth were modestly negative; value has delivered negative returns for a third consecutive year. Instead, reflation signals that were not USD-centric—like relative equity momentum, cross-border FDI/ equities flows and commodity RV—were the best signals for global portfolios. Mispricing vs. rates worked well for G10. Growth signals will still be relevant but are taking an early-stage breather. Our growth model is nearly neutral on high beta FX. Risks are still biased to firming growth judging from data surprises. A lesson from 2021 is that structural changes in the macro backdrop, i.e. the US fiscal boost, should be accounted for when interpreting model results. USD should not always be considered the default funding currency. Yields remain low on carry baskets so returns remain contingent on growth. Carry baskets were resilient to higher US yields in Q1 since improving global growth was an important offset. We continue to downplay value as dispersions are still narrow. 22 Dobromir Tzotchev, PhD (44-20) 7134-5331 dobromir.tzotchev@jpmorgan.com Global Quantitative & Derivatives Strategy 20 August 2021 Figure 22: T.E.A.M model has recently neutralized its short position in the broad USD T.E.A.M cross-sectional sector weights (in %) for the USD, EM and G10 ex USD Net USD weight (%) 80 G10 ex USD 60 EM 40 20 0 -20 -40 -60 -80 2017 2019 2021 Source: J.P. Morgan Regime detection The Adaptive Regime Compass: Measuring Equity Market Similarities with ML Algorithms We highlight a ML-based approach that can be used to locate periods in the past that are similar to the current constellation of macro and financial variables. The ML technique helps to shortlist relevant market drivers from a broader list of variables and investors can make more informed market timing decisions. From this perspective, the ARC framework can have a wide array of applications, ranging from equity risk scaling, dynamic recommendations for systematic hedging, risk on/off signals for carry strategies to asset allocation, risk premia allocation etc. It is well recognized that financial investment returns vary greatly in a variety of macroeconomic and market regimes. This empirical experience prompts the development of a quantitative measure of regime similarity. A framework to systematically and scientifically identify regime similarity, i.e. the Adaptive Regime Compass (ARC) has been proposed in Introducing the Adaptive Regime Compass: Measuring Equity Market Similarities with ML Algorithms. Investors can apply this approach and gauge market environment based on the return distribution and risk profile in similar periods, and can thus navigate across market conditions. Within the ARC framework, a machine learning technique (Random Forest) is leveraged to shortlist relevant market drivers from a broader list of variables concerning market risks, investors’ positioning and economic backdrop. By focusing on key dimensions of a dataset that defines a market regime, a distance measure is applied that enables to quantitatively measure similarity to historical periods. The development of a vector representation of the regime as well as the similarity measure can be used to derive a conditional return distribution for financial assets. 23 Dobromir Tzotchev, PhD (44-20) 7134-5331 dobromir.tzotchev@jpmorgan.com Global Quantitative & Derivatives Strategy 20 August 2021 Figure 23: Illustration of the regime identification process by the Adaptive Regime Compass framework Source: J.P. Morgan Equity Derivatives Strategy By systematically drawing parallels to historical periods that exhibit similar features, investors can make more informed decisions from a market timing perspective (increasing or decreasing exposure to an underlying asset considering the regime signal) and an asset allocation perspective (rotating into assets that will likely perform better for a given market regime). From this perspective, the ARC framework can have a wide array of applications, ranging from equity risk scaling, dynamic recommendations for systematic hedging, risk on/off signals for carry strategies to asset allocation, risk premia allocation etc. As proof of concept, a trading strategy on S&P 500 and Nikkei 225 has been backtested where equity exposure is determined by the distribution of investment returns under similar periods in the past. The analysis shows that adjusting equity exposure in response to outputs of the regime model can materially outperform a benchmark strategy of buy and hold on a risk adjusted basis. 24 Dobromir Tzotchev, PhD (44-20) 7134-5331 dobromir.tzotchev@jpmorgan.com Global Quantitative & Derivatives Strategy 20 August 2021 Figure 24: Prototype risk-control strategy performance versus Buy & Hold strategy for the SPX 240 220 Ret Strategy B&H 18% 16% Strategy B&H 200 Vol Info 14% 18% 1.26 0.88 180 Max DD -19% -34% Calmar 0.91 0.47 160 Figure 25: Prototype risk-control strategy performance versus Buy & Hold strategy for the NKY 240 Strategy B&H Strategy B&H 220 Ret 15% 14% Vol 200 Info 14% 19% 1.08 0.76 Max DD 180 Calmar -15% -31% 1.03 0.46 160 140 140 120 120 100 100 80 Jun-16 Dec-16 Jun-17 Dec-17 Jun-18 Dec-18 Jun-19 Dec-19 Jun-20 Dec-20 80 Jun-16 Dec-16 Jun-17 Dec-17 Jun-18 Dec-18 Jun-19 Dec-19 Jun-20 Dec-20 Source: J.P. Morgan Equity Derivatives Strategy, Bloomberg Finance L.P. * Back-test result is not Source: J.P. Morgan Equity Derivatives Strategy, Bloomberg Finance L.P. * Back-test result is not indicative of future performance. The analysis incorporates 5bps t-cost on monthly rebalances. indicative of future performance. The analysis incorporates 5bps t-cost on monthly rebalances. Distance measure for quantifying market regimes with applications to FX vol trading In Distance measure for quantifying market regimes for FX vol trading a method for quantifying the perpetual dilemma: which historical periods are similar has been proposed. The market conditions for two time periods are considered similar if the Mahalanobis distance between them is small. The Mahalanobis distance, a commonly used metric, is essentially a multidimensional z-score with the multidimensional vector being a difference between a reference (e.g. today) and another date, and where the components of the vector are various market and economic data points at time T. It can be thought of as a way to compute the distance between two points given some underlying distribution or as a way to compute the distance between a point and a distribution (this is analogous to a standard z-score). The Mahalanobis distance versus is preferable to the Euclidean distance as in the case of the Mahalanobis distance the impact of highly redundant timeseries is suppressed. The Mahalanobis distance is actually equivalent to the Euclidean distance on the PCA-transformed dataset. 25 Dobromir Tzotchev, PhD (44-20) 7134-5331 dobromir.tzotchev@jpmorgan.com Global Quantitative & Derivatives Strategy 20 August 2021 Figure 26: An example of an S-1 matrix on an 11 variable basket (aka Global risk markets basket). The strongly negative term in S-1 matrix offsets the combined effect of the highly correlated VXYEM and VXY-GL. A similar though somewhat less detrimental case is seen for EUR and GBP swaptions. Source: J.P. Morgan As a proof-of-concept the framework is applied to seven baskets: global risk basket, positioning basket, economics/broad market backdrop basket, three FX vol baskets (USD, EUR and JPY vs G10) and the overall basket that incorporates variables from other six baskets, overweighting FX vols. The Mahalanobis distance is constrained to the 10%-tile and then a visualization of the similarity between today’s market vs. history going back to 2006 is generated. Intuitively correct, we find the current broad market conditions have the smallest distance to the pre-GFC, 2014, 2017-2019 (with interruptions) and finally for the late 2020 period. Of course there are also some shortcomings to this distance function. The key one is that the covariance is fixed (at least in this implementation) rather than time varying, thus risking going stale if the relationships change over time. Also, there is still the ‘outlier problem’ since this is a quadratic distance formula. An extreme value in a single component can cause the distance to be extreme which may or may not be a desired part of the model. 26 Dobromir Tzotchev, PhD (44-20) 7134-5331 dobromir.tzotchev@jpmorgan.com Global Quantitative & Derivatives Strategy 20 August 2021 Figure 27: The overall basket highlights similarity of the current backdrop with pre-GFC, 2104, 2017-2019 (w/ interruptions) and finally late 2020. Note that FX vols are overweight in the Overall basket even though Mahalanobis framework tends to suppress redundancies. Doubles removed from the Overall basket Source: J.P. Morgan Thematic investing: global outlook and key themes Thematic investing is a strategy of investing in new and fast-growing market segments, based on a certain theme, often tied to technological, macroeconomic, regulatory or other developments. These themes often overlap with price momentum, revenue growth, speculative investors’ interest, and accelerated institutional and retail flows. Thematic investing hence can be seen as a combination of investing in custom defined, emerging industry sectors and momentum investing. The most common application of thematic investing is in identifying a portfolio of stocks exposed to a perceived ‘hot’ area. However, the approach can be more systematic in identifying relevant stocks, weighting, risk managing, consideration of valuations, and anticipating inflection points (e.g. to avoid a theme jumping the shark). This is important, as thematic investing often picks up momentum, and can result in unsustainable valuations and subsequent losses for latecomer investors. One example of the risks involved in thematic investing is the popular theme of Solar energy. After a strong run with over 300% gains during the COVID-19 pandemic, these stocks have declined by nearly 50% this year (see here). With J.P. Morgan's QUEST Thematic Investing framework machine learning and natural language processing (NLP) tools on big data (news-flow) are leveraged to capture a more dynamic and accurate (weighted) representation of themes in the market. Apart from performance, our analytical tools enable us to track developments on the themes (e.g. news volume trend, ETF fund flows), as well as cross-linkages between the themes and signs of crowding. Macro and fundamental considerations are an important part of thematic investing, and we rely on J.P. Morgan's global research to analyze the themes, industry dynamics, and potential changes in the macro environment. In the report Thematic Investing Handbook Global Outlook and Key Themes 17 investment themes are presented in various areas such as: Paradigm Shifts - 27 Dobromir Tzotchev, PhD (44-20) 7134-5331 dobromir.tzotchev@jpmorgan.com Global Quantitative & Derivatives Strategy 20 August 2021 opportunities that are emerging from new business models, technological breakthroughs, etc.; Secular Growth – areas where fundamental drivers are well known and relatively steady, but are likely to remain strong for a longer period of time; and Macro/Market themes – these aim to capture inflection points in cycles such as the recent surge in commodity prices, geopolitical trends, market-structure issues, etc. By broader category, themes covered in the report include Digitalization (e.g. Cloud computing, Edge connectivity, Telehealth, Online Gaming, Cybersecurity, Fintech), Innovation (e.g. Electric and Autonomous Vehicles, Blockchain and Cryptocurrencies, Genomic Revolution, etc.), Industry 4.0 (e.g. Robotics and Automation, 3D printing, etc.); Sustainable Resources (Renewable Energy, Water Security, Sustainable Food, etc.); and Macro themes (e.g. Return of Inflation, Commodity Supercycle and Re-opening). Systematic strategies based on retail trading activities Retail Order Flow in US Equity Options: Case Studies and Signal Value In a “A (Sub)Penny Saved” we analyzed retail orders in the US equities market, and confirmed that retail buying is a short term continuation signal. In a subsequent report, we discussed retail trading activity in the US options market in which we briefly explored the use of option order flow as a volatility trading signal. In the report Retail Order Flow in US Equity Options: Case Studies and Signal Value a further look at retail activity in the US options market has been taken, by providing some interesting case studies, and exploring its potential signal value as a complement to retail order flow on cash equities. Due to the heterogeneity of option contracts, the number of contracts traded and premium paid may not be appropriate representations of option risk. Greeks, such as delta and gamma, are better measures, and allow for a reasonable comparison across securities. A couple of interesting case studies, involving NASDAQ 100 ETF and options, and the VIX index and ETP options, have been examined to better understand retail option trading behaviour. We find that retail traders utilize options as an alternative and high leverage way of accessing high price stocks and that the option delta imbalances are only weakly correlated with cash equity imbalance on most stocks. Furthermore we find that SPY and QQQ options are used as hedging instruments by retail traders and that retail investors see TQQQ as a substitute for QQQ call options. In addition high retail participation in VIX compared to other index options is due to the small notional for VIX options and the retail investors who trade VIX options are more likely to have intentionally sought out the capability and are likely more informed/sophisticated than those who trade VIX ETP options Some previous research has shown that informed traders prefer options to take advantage of the high leverage. This begs the question of whether the retail option order imbalance data has any signal value. Finally some results on using retail option activity data as a directional trading signal are shown. The following definition of delta imbalance as the trading signal has been used.. 28 Dobromir Tzotchev, PhD (44-20) 7134-5331 dobromir.tzotchev@jpmorgan.com Global Quantitative & Derivatives Strategy 20 August 2021 Δ =$ Δ −$ Δ Δ = |$ Δ | + |$ Δ| Δ =$ Δ −$ Δ Δ = |$ Δ = Δ | + |$ Δ+ Δ+ Δ| Δ Δ We sort these stocks into deciles by their delta imbalance. Going long the top decile and short the bottom decile produces the performances seen in Figure 28 and Figure 29. It appears that the signaling value decays relatively quickly, as the weekly holding period Sharpe ratio is materially lower than the daily holding period. Figure 28: Top vs. bottom decile daily holding period(Sharpe = 1.69) 1.4 1.2 1.0 Jul 2020 Oct 2020 Jan 2021 Source: J.P. Morgan Figure 29: Top vs. bottom decile weekly holding period(Sharpe = 0.55) Apr 2021 1.5 1.0 Jul 2020 Source: J.P. Morgan Oct 2020 Jan 2021 Apr 2021 29 Dobromir Tzotchev, PhD (44-20) 7134-5331 dobromir.tzotchev@jpmorgan.com Global Quantitative & Derivatives Strategy 20 August 2021 Do Retail Traders Move Commodity Markets? Impacts of Retail Order Flow on Commodity Momentum Strategies In the report Do Retail Traders Move Commodity Markets? the impacts of retail order flow to on gold (GLD), silver (SLV), and oil (USO) have been studied. The data set contains retail market orders only, and therefore represents liquidity taking activity. At the same time, academic studies have shown that CTA strategies are also takers of market liquidity8. We hypothesize that CTA strategies outperform when they provide liquidity to retail investors, and underperforms when they compete for liquidity with retail investors. To test the hypothesis, we define the daily retail demand/supply imbalance for each ETF as follows and then filter the trades in a prototypical momentum strategy: − = + Strategy 1: If the momentum trade direction is positive (negative), then the strategy will go long (short) if the retail flow imbalance is less than .05 (greater than -.05) and stay flat otherwise. Strategy 2: If the momentum trade direction is positive (negative), then the strategy will go long (short) if the retail flow imbalance is less than 0 (greater than 0) and stay flat otherwise. Strategy 3: If the momentum trade direction is positive (negative), then the strategy will go long (short) if the retail flow imbalance is less than -.05 (greater than .05) and stay flat otherwise. Each strategy is more restrictive that the previous one. In the below Figure 30, the results for oil (USO) are shown with the momentum signal being based on 1 week lookback. The additional three curves show a progressive filtering based on the retail flow data. 8 Kang, Wenjin, Geert Rouwenhourst, Ke Tang, 2020, A Tale of Two Premiums: The Role of Hedgers and Speculators in Commodity Futures Markets, Journal of Finance, Vol 75.1 30 Dobromir Tzotchev, PhD (44-20) 7134-5331 dobromir.tzotchev@jpmorgan.com Global Quantitative & Derivatives Strategy 20 August 2021 Figure 30: Oil Long/Short Momentum Strategy filtered with Retail Order Flow oil Momentum_Sharpe = 0.5 Momentum_Flow Sharpe1 = 0.63 Momentum_Flow Sharpe2 = 0.57 Momentum_Flow Sharpe3 = 0.94 colour Momentum Momentum_Flow1 1.0 Momentum_Flow2 Momentum_Flow3 0.5 Cumulative Return 0.0 2016 Source: J.P. Morgan 2018 Date 2020 Table 12: Backtest results Oil Long/Short Momentum Momentum Flow 1 Momentum Flow 2 Momentum Flow 3 Source: J.P. Morgan Mean 0.23 0.29 0.28 0.51 Sdev 0.46 0.47 0.48 0.54 Sharpe 0.5 0.63 0.57 0.94 Drawdown -0.38 -0.37 -0.34 -0.22 It can be seen that the more restrictive strategies tend to provide better Sharpe ratios. Results have been somewhat similar for silver and inclusive for gold. Furthermore, the retail buying tends to be more coordinated and persistent than retail selling. Therefore the liquidity premium may be more elevated on retail buying flow than selling flow. A separate analysis of the performances of the long and short positons supports this finding. In the end, the retail flow data has been compared with BBG CFTC data. The correlation between these two is inconclusive and this gives us more confidence in the unique value of our retail flow data. Structural breaks in equity risk premia In the report Disentangling momentum and carry in commodities, structural breaks in risk premia and latest model views, the questions of how equity risk premia has evolved over time and whether there have been any structural breaks in the levels of risk premia were analyzed. Break point detection algorithm has been used to find out if equity styles are now commanding lower risk premia compared to historical levels. First, factor risk premia are estimated by running cross-sectional regressions on monthly excess returns of MSCI World stocks: 31 Dobromir Tzotchev, PhD (44-20) 7134-5331 dobromir.tzotchev@jpmorgan.com Global Quantitative & Derivatives Strategy 20 August 2021 ,− , =+ , , + , ,+ , ,+ , ,+ , , +, where , is the Value factor loading of stock i at t-1, and similarly for factor loadings on Momentum, Size, Quality and Volatility. The lambdas are the estimated monthly risk premia commanded by the corresponding factor. The idea is to detect if the mean of the risk premia has changed significantly after certain dates, which will be identified as structural break points. We consider the recursive residuals from the mean model: =+ It is known that the cumulative sum (CUSUM) of the recursive residuals follows a Brownian motion if the mean is constant. On the other hand, if there is a sudden change in the mean level, the CUSUM process will cross some predefined threshold, indicating the existence of a structural break point. Each structural break is required to last for at least 3 years9. It has been found that the Value risk premium has dropped from a high of 6.9% in early 2000s to 1% p.a. in recent years. Momentum and Quality risk premia are relatively more stable, although both have decreased to about 2% as of latest. There are also increasingly positive premia on large caps and volatile stocks, which is quite similar to what we saw during the dot-com bubble in 2000. Figure 31: Break points in Value and Momentum risk premia Period 1995(1) - 2000(10) 2000(11) - 2006(9) 2006(10) - 2016(4) 2016(5) - 2021(4) Value risk premia 3.5% 6.9% 1.8% 1.0% Period 1995(1) - 2003(5) 2003(6) - 2009(4) 2009(5) - 2014(4) 2014(5) - 2021(4) Momentum risk premia 3.7% 1.7% 0.0% 1.5% 9 The R package strucchange is used to determine the break points in each risk premia. 32 Dobromir Tzotchev, PhD (44-20) 7134-5331 dobromir.tzotchev@jpmorgan.com Global Quantitative & Derivatives Strategy 20 August 2021 Period 1995(1) - 2001(1) 2001(2) - 2006(1) 2006(2) - 2011(1) 2011(2) - 2021(4) Volatility risk premia (-ve = prefer low vol) 1.2% -2.8% -0.9% 0.3% Period 1995(1) - 2003(5) 2003(6) - 2008(5) 2008(6) - 2014(3) 2014(4) - 2021(4) Size risk premia (-ve: prefer small cap) 1.4% -3.3% -1.3% -0.2% 33 Dobromir Tzotchev, PhD (44-20) 7134-5331 dobromir.tzotchev@jpmorgan.com Global Quantitative & Derivatives Strategy 20 August 2021 List of H1'21 reports with focus on systematic investing Team Global Quantitative and Derivatives Strategy Global Quantitative and Derivatives Strategy Global Quantitative and Derivatives Strategy Global Quantitative and Derivatives Strategy Global Quantitative and Derivatives Strategy Global Quantitative and Derivatives Strategy Global FX Strategy Global Quantitative and Derivatives Strategy Global FX Strategy Global FX Strategy Global Quantitative and Derivatives Strategy Global Quantitative and Derivatives Strategy Global Quantitative and Derivatives Strategy Global Quantitative and Derivatives Strategy Global Quantitative and Derivatives Strategy European Credit Strategy and Derivative Research Global FX Strategy Global FX Strategy Chief Global Markets Strategist Chief Global Markets Strategist Global Quantitative and Derivatives Strategy Global Quantitative and Derivatives Strategy Global Quantitative and Derivatives Strategy Global Quantitative and Derivatives Strategy Global Quantitative and Derivatives Strategy Global Quantitative and Derivatives Strategy Global Quantitative and Derivatives Strategy Global Quantitative and Derivatives Strategy Global Quantitative and Derivatives Strategy Lead AC Dobromir Tzotchev Dobromir Tzotchev Dobromir Tzotchev Dobromir Tzotchev Dobromir Tzotchev Lorenzo Ravagli Meera Chandan Berowne Hlavaty Meera Chandan Meera Chandan Peng Cheng Peng Cheng Peng Cheng Thomas J Murphy Peng Cheng Shivam Ghosh Ladislav Jankovic Ladislav Jankovic Marko Kolanovic Marko Kolanovic Tony SK Lee Dobromir Tzotchev Berowne Hlavaty Peng Cheng Dobromir Tzotchev Dobromir Tzotchev Dobromir Tzotchev Khuram Chaudhry Khuram Chaudhry Title Quantitative Perspectives on CrossAsset Risk Premia Quantitative Perspectives on CrossAsset Risk Premia Quantitative Perspectives on CrossAsset Risk Premia Quantitative Perspectives on CrossAsset Risk Premia Risk Premia Portfolio Construction via Uncorrelated Clusters JPM FX - Derivatives Chartpack Notes T.E.A.M. 2.0* Big Data and AI Strategies Subtitle Risk premia performance review, trend-following in factors and latest model views Quarterly review, Credit value, Synthetic defensive long vol and latest model views Disentangling momentum and carry in commodities, structural breaks in risk premia and latest model views Enhancing active ETF performance, cluster portfolio and tail risk parity updates, and latest model views Introducing Autoencoders as an extension of Principal Components RV of OTM strangles as sentiment indicators remain in risk-loving mode A time-series multi-factor approach to FX Assessing a range of NLP Transformer Models such as BERT, Electra, Funnel, GPT2, MPNet and variants FX Macro Quant A neutralization across most signals FX Macro Quant Mid-year Outlook Cross Asset Volatility Low yields and little value meet neutralizing growth momentum What can option implied skew tell us about bond equity correlation? Cross Asset Volatility Retail Order Flow in US Equity Options Do Retail Traders Move Commodity Markets? Global Equity Derivatives Systematically monetize bond/equity correlation premium via vanilla options Case Studies and Signal Value Impacts of Retail Order Flow on Commodity Momentum Strategies Optimal Variance Replicating Portfolio Factor Tracker 2Q performance and H2 Outlook FX Derivatives Research Note Distance measure for quantifying market regimes for FX vol trading FX Derivatives Research Note Double no-touches go quantum, the first look Thematic Investing Handbook Market and Volatility Commentary Introducing the Adaptive Regime Compass Risk Premia Investing in the Age of Machines Big Data and AI Strategies Inflation Expectation Tracking Equity Baskets Quantitative Perspectives on CrossAsset Risk Premia Quantitative Perspectives on CrossAsset Risk Premia Quantitative Perspectives on CrossAsset Risk Premia ESGQ ESGQ Global Outlook and Key Themes Outlook remains positive - buy the dip; where are the bubbles and COVIDGreen risk Measuring Equity Market Similarities with ML Algorithms Investable Strategies with Machine Learning Asset Pricing Models for Global Equities Assessing a range of NLP Transformer Models such as BERT, Electra, Funnel, GPT2, MPNet and variants A Pure Equity Factor Approach Hedging rising inflation, trend-following system review, Bitcoin trendfollowing backtest and latest model views Reviewing 2020 performance, a note on January effect and latest model views Pure ESG factors, new research on risk premia and latest model views ESG Investing - short term vulnerabilities, long term opportunities Solid and consistent returns for ESGQ, concerns about the high correlation to Momentum & Technology 34 Dobromir Tzotchev, PhD (44-20) 7134-5331 dobromir.tzotchev@jpmorgan.com Global Quantitative & Derivatives Strategy 20 August 2021 European Credit Strategy and Derivative Research European Credit Strategy and Derivative Research Global Quantitative and Derivatives Strategy Global Quantitative and Derivatives Strategy Global Quantitative and Derivatives Strategy Shivam Ghosh Shivam Ghosh Khuram Chaudhry Peng Cheng Peng Cheng Do Good; Get Paid Virtue isn't the only reward ESG - Environmental, Social & Governance Investing A (Sub)Penny Saved Big Data and AI Strategies Estimating ESG Alpha in European Credit Active management under ESG constraints Introducing the 'Human Capital Factor' Tracking Retail Trading Activity in US Equities and ETFs Tracking Retail Activity in the US Equity Options Market Background reports Team Global Quantitative and Derivatives Strategy Global Quantitative and Derivatives Strategy Global Quantitative and Derivatives Strategy Global Quantitative and Derivatives Strategy Global Quantitative and Derivatives Strategy Global Quantitative and Derivatives Strategy Global Quantitative and Derivatives Strategy Global FX Strategy Global FX Strategy Global Quantitative and Derivatives Strategy Global Quantitative and Derivatives Strategy Global Quantitative and Derivatives Strategy Global Quantitative and Derivatives Strategy Global Quantitative and Derivatives Strategy Lead AC Dobromir Tzotchev Dobromir Tzotchev Dobromir Tzotchev Dobromir Tzotchev Lorenzo Ravagli Dobromir Tzotchev Dobromir Tzotchev Meera Chandan Meera Chandan Haoshun Liu Dobromir Tzotchev Khuram Chaudhry Khuram Chaudhry Peng Cheng Title The quest for pure equity factor exposure Defensive Risk Premia Subtitle How to eliminate the unwanted biases in equity factors? Systematic Strategies for the Risk-Off Times Custom Performance Attribution based on Portfolio Holdings Decomposing Risk and Return Drivers via Factor-Mimicking Portfolios Market-neutral carry strategies Trading vol-of-vol risk premium Designing robust trend-following system Quantitative Perspectives on CrossAsset Risk Premia J.P. Morgan FX single-factor style frameworks Harvesting carry without market risk Harvesting smile convexity across asset classes Behind the scenes of trend-following Macro-Economic Backdrop, Liquidity in Equity Styles and Tail Risk Parity T.E.A.M.* Introducing a multi-factor approach to FX AI and Big Data Approach to Thematic Investing A Quantitative Framework for Cross Asset Style Timing ESG - Environmental, Social & Governance Investing ESGQ Structural digitalization trends in cloud computing, telehealth, video gaming and cybersecurity Machine Learning, Macro and Time-Series models providing views for Portfolio Tilting Launching JPM's ESGQ - A Quantitative ESG Metric for stock selection models. Is ESG performance simply a measure of long Technology & short Energy? Follow the Robinhood Money Buying Behavior and Market Impacts of Individual Traders 35 Dobromir Tzotchev, PhD (44-20) 7134-5331 dobromir.tzotchev@jpmorgan.com Global Quantitative & Derivatives Strategy 20 August 2021 Disclosures Analyst Certification: The Research Analyst(s) denoted by an “AC” on the cover of this report certifies (or, where multiple Research Analysts are primarily responsible for this report, the Research Analyst denoted by an “AC” on the cover or within the document individually certifies, with respect to each security or issuer that the Research Analyst covers in this research) that: (1) all of the views expressed in this report accurately reflect the Research Analyst’s personal views about any and all of the subject securities or issuers; and (2) no part of any of the Research Analyst's compensation was, is, or will be directly or indirectly related to the specific recommendations or views expressed by the Research Analyst(s) in this report. For all Korea-based Research Analysts listed on the front cover, if applicable, they also certify, as per KOFIA requirements, that the Research Analyst’s analysis was made in good faith and that the views reflect the Research Analyst’s own opinion, without undue influence or intervention. All authors named within this report are Research Analysts unless otherwise specified. In Europe, Sector Specialists (Sales and Trading) may be shown on this report as contacts but are not authors of the report or part of the Research Department. Important Disclosures This report is a product of the research department's Global Quantitative and Derivatives Strategy group. Views expressed may differ from the views of the research analysts covering stocks or sectors mentioned in this report. Structured securities, options, futures and other derivatives are complex instruments, may involve a high degree of risk, and may be appropriate investments only for sophisticated investors who are capable of understanding and assuming the risks involved. Because of the importance of tax considerations to many option transactions, investors considering options should consult with their tax advisor as to how taxes affect the outcome of contemplated option transactions. Company-Specific Disclosures: Important disclosures, including price charts and credit opinion history tables, are available for compendium reports and all J.P. Morgan–covered companies, and certain non-covered companies, by visiting https://www.jpmm.com/research/disclosures, calling 1-800-477-0406, or e-mailing research.disclosure.inquiries@jpmorgan.com with your request. Explanation of Equity Research Ratings, Designations and Analyst(s) Coverage Universe: J.P. Morgan uses the following rating system: Overweight [Over the next six to twelve months, we expect this stock will outperform the average total return of the stocks in the analyst’s (or the analyst’s team’s) coverage universe.] Neutral [Over the next six to twelve months, we expect this stock will perform in line with the average total return of the stocks in the analyst’s (or the analyst’s team’s) coverage universe.] Underweight [Over the next six to twelve months, we expect this stock will underperform the average total return of the stocks in the analyst’s (or the analyst’s team’s) coverage universe.] Not Rated (NR): J.P. Morgan has removed the rating and, if applicable, the price target, for this stock because of either a lack of a sufficient fundamental basis or for legal, regulatory or policy reasons. The previous rating and, if applicable, the price target, no longer should be relied upon. An NR designation is not a recommendation or a rating. In our Asia (ex-Australia and ex-India) and U.K. small- and mid-cap equity research, each stock’s expected total return is compared to the expected total return of a benchmark country market index, not to those analysts’ coverage universe. If it does not appear in the Important Disclosures section of this report, the certifying analyst’s coverage universe can be found on J.P. Morgan’s research website, www.jpmorganmarkets.com. J.P. Morgan Equity Research Ratings Distribution, as of July 03, 2021 J.P. Morgan Global Equity Research Coverage* IB clients** JPMS Equity Research Coverage* IB clients** Overweight (buy) 52% 54% 49% 77% Neutral (hold) 37% 48% 39% 69% Underweight (sell) 11% 39% 13% 55% *Please note that the percentages might not add to 100% because of rounding. **Percentage of subject companies within each of the "buy," "hold" and "sell" categories for which J.P. Morgan has provided investment banking services within the previous 12 months. For purposes only of FINRA ratings distribution rules, our Overweight rating falls into a buy rating category; our Neutral rating falls into a hold rating category; and our Underweight rating falls into a sell rating category. Please note that stocks with an NR designation are not included in the table above. This information is current as of the end of the most recent calendar quarter. Equity Valuation and Risks: For valuation methodology and risks associated with covered companies or price targets for covered companies, please see the most recent company-specific research report at http://www.jpmorganmarkets.com, contact the primary analyst or your J.P. Morgan representative, or email research.disclosure.inquiries@jpmorgan.com. For material information about the proprietary models used, please see the Summary of Financials in company-specific research reports and the Company Tearsheets, which are 36 Dobromir Tzotchev, PhD (44-20) 7134-5331 dobromir.tzotchev@jpmorgan.com Global Quantitative & Derivatives Strategy 20 August 2021 available to download on the company pages of our client website, http://www.jpmorganmarkets.com. This report also sets out within it the material underlying assumptions used. A history of J.P. Morgan investment recommendations disseminated during the preceding 12 months can be accessed on the Research & Commentary page of http://www.jpmorganmarkets.com where you can also search by analyst name, sector or financial instrument. Explanation of Credit Research Valuation Methodology, Ratings and Risk to Ratings: J.P. Morgan uses a bond-level rating system that incorporates valuations (relative value) and our fundamental view on the security. Our fundamental credit view of an issuer is based on the company's underlying credit trends, overall creditworthiness and our opinion on whether the issuer will be able to service its debt obligations when they become due and payable. We analyze, among other things, the company's cash flow capacity and trends and standard credit ratios, such as gross and net leverage, interest coverage and liquidity ratios. We also analyze profitability, capitalization and asset quality, among other variables, when assessing financials. Analysts also rate the issuer, based on the rating of the benchmark or representative security. Unless we specify a different recommendation for the company’s individual securities, an issuer recommendation applies to all of the bonds at the same level of the issuer’s capital structure. We may also rate certain loans and preferred securities, as applicable. This report also sets out within it the material underlying assumptions used. We use the following ratings for bonds (issues), issuers, loans, and preferred securities: Overweight (over the next three months, the recommended risk position is expected to outperform the relevant index, sector, or benchmark); Neutral (over the next three months, the recommended risk position is expected to perform in line with the relevant index, sector, or benchmark); and Underweight (over the next three months, the recommended risk position is expected to underperform the relevant index, sector, or benchmark). J.P. Morgan Emerging Markets Sovereign Research uses Marketweight, which is equivalent to Neutral. NR is Not Rated. In this case, J.P. Morgan has removed the rating for this particular security or issuer because of either a lack of a sufficient fundamental basis or for legal, regulatory or policy reasons. The previous rating no longer should be relied upon. An NR designation is not a recommendation or a rating. NC is Not Covered. An NC designation is not a rating or a recommendation. For CDS, we use the following rating system: Long Risk (over the next three months, the credit return on the recommended position is expected to exceed the relevant index, sector or benchmark); Neutral (over the next three months, the credit return on the recommended position is expected to match the relevant index, sector or benchmark); and Short Risk (over the next three months, the credit return on the recommended position is expected to underperform the relevant index, sector or benchmark). J.P. Morgan Credit Research Ratings Distribution, as of July 03, 2021 Global Credit Research Universe* IB clients** Overweight 30% 64% Neutral 54% 64% Underweight 15% 65% *Please note that the percentages might not add to 100% because of rounding. **Percentage of subject companies within each of the "buy," "hold" and "sell" categories for which J.P. Morgan has provided investment banking services within the previous 12 months. The Credit Research Rating Distribution is at the issuer level. Issuers with an NR or an NC designations are not included in the table above. This information is current as of the end of the most recent calendar quarter. Analysts' Compensation: The research analysts responsible for the preparation of this report receive compensation based upon various factors, including the quality and accuracy of research, client feedback, competitive factors, and overall firm revenues. Registration of non-US Analysts: Unless otherwise noted, the non-US analysts listed on the front of this report are employees of non-US affiliates of J.P. Morgan Securities LLC, may not be registered as research analysts under FINRA rules, may not be associated persons of J.P. Morgan Securities LLC, and may not be subject to FINRA Rule 2241 or 2242 restrictions on communications with covered companies, public appearances, and trading securities held by a research analyst account. Analysts' Compensation: The research analysts responsible for the preparation of this report receive compensation based upon various factors, including the quality and accuracy of research, client feedback, competitive factors, and overall firm revenues. Other Disclosures J.P. Morgan is a marketing name for investment banking businesses of JPMorgan Chase & Co. and its subsidiaries and affiliates worldwide. QIB Only All research material made available to clients are simultaneously available on our client website, J.P. Morgan Markets, unless specifically permitted by relevant laws. Not all research content is redistributed, e-mailed or made available to third-party aggregators. For all research material available on a particular stock, please contact your sales representative. 37 Dobromir Tzotchev, PhD (44-20) 7134-5331 dobromir.tzotchev@jpmorgan.com Global Quantitative & Derivatives Strategy 20 August 2021 Any long form nomenclature for references to China; Hong Kong; Taiwan; and Macau within this research material are Mainland China; Hong Kong SAR (China); Taiwan (China); and Macau SAR (China). JPMorgan research may, from time to time, write on issuers or securities targeted by economic or financial sanctions imposed or administered by the governmental authorities of the U.S., EU, UK or other relevant jurisdictions (Sanctioned Securities). Nothing in this report is intended to be read or construed as encouraging, facilitating, promoting or otherwise approving investment or dealing in such Sanctioned Securities. Clients should be aware of their own legal and compliance obligations when making investment decisions. Options and Futures related research: If the information contained herein regards options- or futures-related research, such information is available only to persons who have received the proper options or futures risk disclosure documents. Please contact your J.P. Morgan Representative or visit https://www.theocc.com/components/docs/riskstoc.pdf for a copy of the Option Clearing Corporation's Characteristics and Risks of Standardized Options or http://www.finra.org/sites/default/files/Security_Futures_Risk_Disclosure_Statement_2018.pdf for a copy of the Security Futures Risk Disclosure Statement. Changes to Interbank Offered Rates (IBORs) and other benchmark rates: Certain interest rate benchmarks are, or may in the future become, subject to ongoing international, national and other regulatory guidance, reform and proposals for reform. For more information, please consult: https://www.jpmorgan.com/global/disclosures/interbank_offered_rates Private Bank Clients: Where you are receiving research as a client of the private banking businesses offered by JPMorgan Chase & Co. and its subsidiaries (“J.P. Morgan Private Bank”), research is provided to you by J.P. Morgan Private Bank and not by any other division of J.P. Morgan, including, but not limited to, the J.P. Morgan Corporate and Investment Bank and its Global Research division. Legal entity responsible for the production and distribution of research: The legal entity identified below the name of the Reg AC Research Analyst who authored this material is the legal entity responsible for the production of this research. Where multiple Reg AC Research Analysts authored this material with different legal entities identified below their names, these legal entities are jointly responsible for the production of this research. Research Analysts from various J.P. Morgan affiliates may have contributed to the production of this material but may not be licensed to carry out regulated activities in your jurisdiction (and do not hold themselves out as being able to do so). Unless otherwise stated below, this material has been distributed by the legal entity responsible for production. If you have any queries, please contact the relevant Research Analyst in your jurisdiction or the entity in your jurisdiction that has distributed this research material. Legal Entities Disclosures and Country-/Region-Specific Disclosures: Argentina: JPMorgan Chase Bank N.A Sucursal Buenos Aires is regulated by Banco Central de la República Argentina (“BCRA”Central Bank of Argentina) and Comisión Nacional de Valores (“CNV”- Argentinian Securities Commission” - ALYC y AN Integral N°51). Australia: J.P. Morgan Securities Australia Limited (“JPMSAL”) (ABN 61 003 245 234/AFS Licence No: 238066) is regulated by the Australian Securities and Investments Commission and is a Market, Clearing and Settlement Participant of ASX Limited and CHIX. This material is issued and distributed in Australia by or on behalf of JPMSAL only to "wholesale clients" (as defined in section 761G of the Corporations Act 2001). A list of all financial products covered can be found by visiting https://www.jpmm.com/research/disclosures. J.P. Morgan seeks to cover companies of relevance to the domestic and international investor base across all Global Industry Classification Standard (GICS) sectors, as well as across a range of market capitalisation sizes. If applicable, in the course of conducting public side due diligence on the subject company(ies), the Research Analyst team may at times perform such diligence through corporate engagements such as site visits, discussions with company representatives, management presentations, etc. Research issued by JPMSAL has been prepared in accordance with J.P. Morgan Australia’s Research Independence Policy which can be found at the following link: J.P. Morgan Australia - Research Independence Policy. Brazil: Banco J.P. Morgan S.A. is regulated by the Comissao de Valores Mobiliarios (CVM) and by the Central Bank of Brazil. Ombudsman J.P. Morgan: 0800-7700847 / ouvidoria.jp.morgan@jpmorgan.com. Canada: J.P. Morgan Securities Canada Inc. is a registered investment dealer, regulated by the Investment Industry Regulatory Organization of Canada and the Ontario Securities Commission and is the participating member on Canadian exchanges. This material is distributed in Canada by or on behalf of J.P.Morgan Securities Canada Inc. Chile: Inversiones J.P. Morgan Limitada is an unregulated entity incorporated in Chile. China: J.P. Morgan Securities (China) Company Limited has been approved by CSRC to conduct the securities investment consultancy business. Dubai International Financial Centre (DIFC): JPMorgan Chase Bank, N.A., Dubai Branch is regulated by the Dubai Financial Services Authority (DFSA) and its registered address is Dubai International Financial Centre - The Gate, West Wing, Level 3 and 9 PO Box 506551, Dubai, UAE. This material has been distributed by JP Morgan Chase Bank, N.A., Dubai Branch to persons regarded as professional clients or market counterparties as defined under the DFSA rules. European Economic Area (EEA): Unless specified to the contrary, research is distributed in the EEA by J.P. Morgan AG (“JPM AG”), which is a member of the Frankfurt Stock Exchange, is authorised by the European Central Bank (“ECB”) and is regulated by the Federal Financial Supervisory Authority (BaFin). JPM AG is a company incorporated in the Federal Republic of Germany with a registered office at Taunustor 1, 60310 Frankfurt am Main, the Federal Republic of Germany. The material has been distributed in the EEA to persons regarded as professional investors (or equivalent) pursuant to Art. 4 para. 1 no. 10 and Annex II of MiFID II and its respective implementation in their home jurisdictions (“EEA professional investors”). This material must not be acted on 38 Dobromir Tzotchev, PhD (44-20) 7134-5331 dobromir.tzotchev@jpmorgan.com Global Quantitative & Derivatives Strategy 20 August 2021 or relied on by persons who are not EEA professional investors. Any investment or investment activity to which this material relates is only available to EEA relevant persons and will be engaged in only with EEA relevant persons. Hong Kong: J.P. Morgan Securities (Asia Pacific) Limited (CE number AAJ321) is regulated by the Hong Kong Monetary Authority and the Securities and Futures Commission in Hong Kong, and J.P. Morgan Broking (Hong Kong) Limited (CE number AAB027) is regulated by the Securities and Futures Commission in Hong Kong. JP Morgan Chase Bank, N.A., Hong Kong (CE Number AAL996) is regulated by the Hong Kong Monetary Authority and the Securities and Futures Commission, is organized under the laws of the United States with limited liability. India: J.P. Morgan India Private Limited (Corporate Identity Number - U67120MH1992FTC068724), having its registered office at J.P. Morgan Tower, Off. C.S.T. Road, Kalina, Santacruz - East, Mumbai – 400098, is registered with the Securities and Exchange Board of India (SEBI) as a ‘Research Analyst’ having registration number INH000001873. J.P. Morgan India Private Limited is also registered with SEBI as a member of the National Stock Exchange of India Limited and the Bombay Stock Exchange Limited (SEBI Registration Number – INZ000239730) and as a Merchant Banker (SEBI Registration Number - MB/INM000002970). Telephone: 91-22-6157 3000, Facsimile: 91-22-6157 3990 and Website: www.jpmipl.com. JPMorgan Chase Bank, N.A. - Mumbai Branch is licensed by the Reserve Bank of India (RBI) (Licence No. 53/ Licence No. BY.4/94; SEBI - IN/CUS/014/ CDSL : IN-DP-CDSL-444-2008/ IN-DP-NSDL-2852008/ INBI00000984/ INE231311239) as a Scheduled Commercial Bank in India, which is its primary license allowing it to carry on Banking business in India and other activities, which a Bank branch in India are permitted to undertake. For non-local research material, this material is not distributed in India by J.P. Morgan India Private Limited. Indonesia: PT J.P. Morgan Sekuritas Indonesia is a member of the Indonesia Stock Exchange and is regulated by the OJK a.k.a. BAPEPAM LK. Korea: J.P. Morgan Securities (Far East) Limited, Seoul Branch, is a member of the Korea Exchange (KRX). JPMorgan Chase Bank, N.A., Seoul Branch, is licensed as a branch office of foreign bank (JPMorgan Chase Bank, N.A.) in Korea. Both entities are regulated by the Financial Services Commission (FSC) and the Financial Supervisory Service (FSS). For non-macro research material, the material is distributed in Korea by or through J.P. Morgan Securities (Far East) Limited, Seoul Branch. Japan: JPMorgan Securities Japan Co., Ltd. and JPMorgan Chase Bank, N.A., Tokyo Branch are regulated by the Financial Services Agency in Japan. Malaysia: This material is issued and distributed in Malaysia by JPMorgan Securities (Malaysia) Sdn Bhd (18146-X), which is a Participating Organization of Bursa Malaysia Berhad and holds a Capital Markets Services License issued by the Securities Commission in Malaysia. Mexico: J.P. Morgan Casa de Bolsa, S.A. de C.V.and J.P. Morgan Grupo Financiero are members of the Mexican Stock Exchange and are authorized to act as a broker dealer by the National Banking and Securities Exchange Commission. New Zealand: This material is issued and distributed by JPMSAL in New Zealand only to "wholesale clients" (as defined in the Financial Advisers Act 2008). JPMSAL is registered as a Financial Service Provider under the Financial Service providers (Registration and Dispute Resolution) Act of 2008. Pakistan: J. P. Morgan Pakistan Broking (Pvt.) Ltd is a member of the Karachi Stock Exchange and regulated by the Securities and Exchange Commission of Pakistan. Philippines: J.P. Morgan Securities Philippines Inc. is a Trading Participant of the Philippine Stock Exchange and a member of the Securities Clearing Corporation of the Philippines and the Securities Investor Protection Fund. It is regulated by the Securities and Exchange Commission. Russia: CB J.P. Morgan Bank International LLC is regulated by the Central Bank of Russia. Singapore: This material is issued and distributed in Singapore by or through J.P. Morgan Securities Singapore Private Limited (JPMSS) [MCI (P) 018/04/2020 and Co. Reg. No.: 199405335R], which is a member of the Singapore Exchange Securities Trading Limited, and/or JPMorgan Chase Bank, N.A., Singapore branch (JPMCB Singapore) [MCI (P) 052/09/2020], both of which are regulated by the Monetary Authority of Singapore. This material is issued and distributed in Singapore only to accredited investors, expert investors and institutional investors, as defined in Section 4A of the Securities and Futures Act, Cap. 289 (SFA). This material is not intended to be issued or distributed to any retail investors or any other investors that do not fall into the classes of “accredited investors,” “expert investors” or “institutional investors,” as defined under Section 4A of the SFA. Recipients of this material in Singapore are to contact JPMSS or JPMCB Singapore in respect of any matters arising from, or in connection with, the material. As at the date of this material, JPMSS is a designated market maker for certain structured warrants listed on the Singapore Exchange where the underlying securities may be the securities discussed in this material. Arising from its role as a designated market maker for such structured warrants, JPMSS may conduct hedging activities in respect of such underlying securities and hold or have an interest in such underlying securities as a result. The updated list of structured warrants for which JPMSS acts as designated market maker may be found on the website of the Singapore Exchange Limited: http://www.sgx.com. South Africa: J.P. Morgan Equities South Africa Proprietary Limited and JPMorgan Chase Bank, N.A., Johannesburg Branch are members of the Johannesburg Securities Exchange and are regulated by the Financial Services Board. Taiwan: J.P. Morgan Securities (Taiwan) Limited is a participant of the Taiwan Stock Exchange (company-type) and regulated by the Taiwan Securities and Futures Bureau. Material relating to equity securities is issued and distributed in Taiwan by J.P. Morgan Securities (Taiwan) Limited, subject to the license scope and the applicable laws and the regulations in Taiwan. According to Paragraph 2, Article 7-1 of Operational Regulations Governing Securities Firms Recommending Trades in Securities to Customers (as amended or supplemented) and/or other applicable laws or regulations, please note that the recipient of this material is not permitted to engage in any activities in connection with the material that may give rise to conflicts of interests, unless otherwise disclosed in the “Important Disclosures” in this material. Thailand: This material is issued and distributed in Thailand by JPMorgan Securities (Thailand) Ltd., which is a member of the Stock Exchange of Thailand and is regulated by the Ministry of Finance and the Securities and Exchange Commission, and its registered address is 3rd Floor, 20 North Sathorn Road, Silom, Bangrak, Bangkok 10500. UK: Unless specified to the contrary, research is distributed in the UK by J.P. Morgan Securities plc (“JPMS plc”) which is a member of the London Stock Exchange and is authorised by the Prudential Regulation Authority and regulated by the Financial Conduct Authority and the Prudential Regulation Authority. JPMS plc is registered in England & Wales No. 2711006, Registered Office 25 Bank Street, London, E14 5JP. This material is directed in the UK only to: (a) persons having professional experience in matters relating to investments falling within article 19(5) of the Financial Services and Markets Act 2000 (Financial Promotion) (Order) 2005 (“the FPO”); (b) persons outlined in article 49 of the FPO (high net worth companies, unincorporated 39 Dobromir Tzotchev, PhD (44-20) 7134-5331 dobromir.tzotchev@jpmorgan.com Global Quantitative & Derivatives Strategy 20 August 2021 associations or partnerships, the trustees of high value trusts, etc.); or (c) any persons to whom this communication may otherwise lawfully be made; all such persons being referred to as "UK relevant persons". This material must not be acted on or relied on by persons who are not UK relevant persons. Any investment or investment activity to which this material relates is only available to UK relevant persons and will be engaged in only with UK relevant persons. Research issued by JPMS plc has been prepared in accordance with JPMS plc's policy for prevention and avoidance of conflicts of interest related to the production of Research which can be found at the following link: J.P. Morgan EMEA - Research Independence Policy. U.S.: J.P. Morgan Securities LLC (“JPMS”) is a member of the NYSE, FINRA, SIPC, and the NFA. JPMorgan Chase Bank, N.A. is a member of the FDIC. Material published by non-U.S. affiliates is distributed in the U.S. by JPMS who accepts responsibility for its content. General: Additional information is available upon request. The information in this material has been obtained from sources believed to be reliable. While all reasonable care has been taken to ensure that the facts stated in this material are accurate and that the forecasts, opinions and expectations contained herein are fair and reasonable, JPMorgan Chase & Co. or its affiliates and/or subsidiaries (collectively J.P. Morgan) make no representations or warranties whatsoever to the completeness or accuracy of the material provided, except with respect to any disclosures relative to J.P. Morgan and the Research Analyst's involvement with the issuer that is the subject of the material. Accordingly, no reliance should be placed on the accuracy, fairness or completeness of the information contained in this material. Any data discrepancies in this material could be the result of different calculations and/or adjustments. J.P. Morgan accepts no liability whatsoever for any loss arising from any use of this material or its contents, and neither J.P. Morgan nor any of its respective directors, officers or employees, shall be in any way responsible for the contents hereof, apart from the liabilities and responsibilities that may be imposed on them by the relevant regulatory authority in the jurisdiction in question, or the regulatory regime thereunder. Opinions, forecasts or projections contained in this material represent J.P. Morgan's current opinions or judgment as of the date of the material only and are therefore subject to change without notice. Periodic updates may be provided on companies/industries based on company-specific developments or announcements, market conditions or any other publicly available information. There can be no assurance that future results or events will be consistent with any such opinions, forecasts or projections, which represent only one possible outcome. Furthermore, such opinions, forecasts or projections are subject to certain risks, uncertainties and assumptions that have not been verified, and future actual results or events could differ materially. The value of, or income from, any investments referred to in this material may fluctuate and/or be affected by changes in exchange rates. All pricing is indicative as of the close of market for the securities discussed, unless otherwise stated. Past performance is not indicative of future results. Accordingly, investors may receive back less than originally invested. This material is not intended as an offer or solicitation for the purchase or sale of any financial instrument. The opinions and recommendations herein do not take into account individual client circumstances, objectives, or needs and are not intended as recommendations of particular securities, financial instruments or strategies to particular clients. The recipients of this material must make their own independent decisions regarding any securities or financial instruments mentioned herein and should seek advice from such independent financial, legal, tax or other adviser as they deem necessary. J.P. Morgan may trade as a principal on the basis of the Research Analysts’ views and research, and it may also engage in transactions for its own account or for its clients’ accounts in a manner inconsistent with the views taken in this material, and J.P. Morgan is under no obligation to ensure that such other communication is brought to the attention of any recipient of this material. Others within J.P. Morgan, including Strategists, Sales staff and other Research Analysts, may take views that are inconsistent with those taken in this material. Employees of J.P. Morgan not involved in the preparation of this material may have investments in the securities (or derivatives of such securities) mentioned in this material and may trade them in ways different from those discussed in this material. This material is not an advertisement for or marketing of any issuer, its products or services, or its securities in any jurisdiction. "Other Disclosures" last revised July 17, 2021. Copyright 2021 JPMorgan Chase & Co. All rights reserved. This material or any portion hereof may not be reprinted, sold or redistributed without the written consent of J.P. Morgan. #$J&098$#*P 40 Completed 20 Aug 2021 04:38 PM BST Disseminated 20 Aug 2021 04:47 PM BST
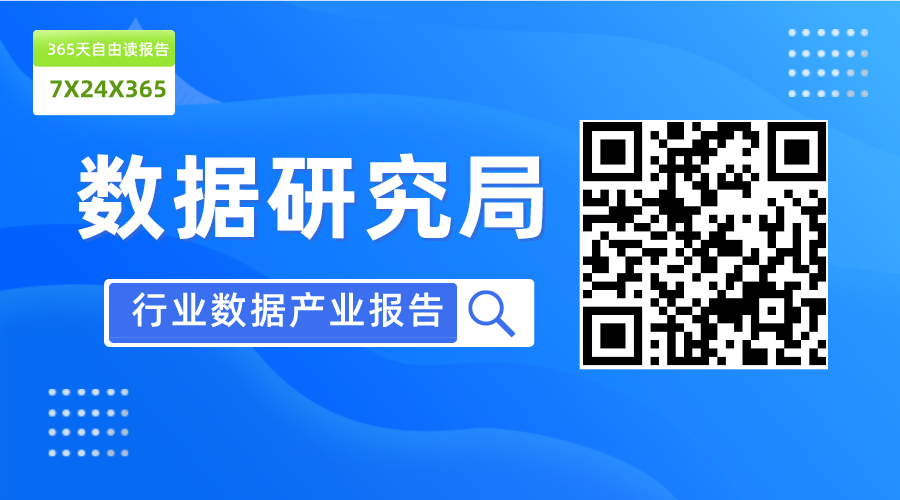
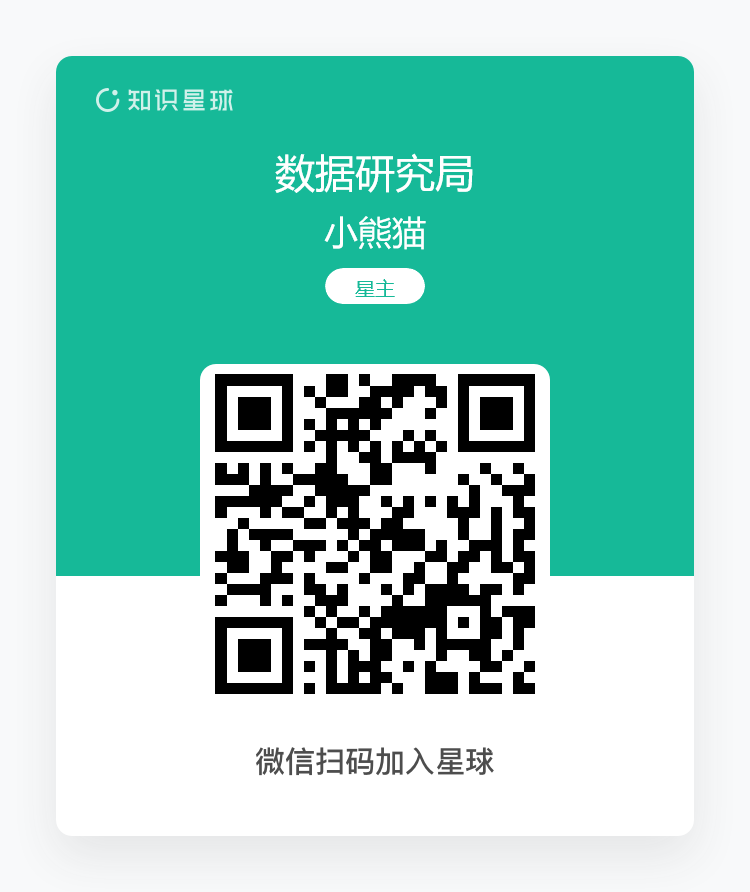