PROJECT DOCUMENTSSpeculation and price volatility in the coffee marketJavier Aliaga Lordemann Claudio Mora-García Nanno MulderThank you for your interest in this ECLAC publicationECLAC PublicationsPlease register if you would like to receive information on our editorial products and activities. When you register, you may specify your particular areas of interest and you will gain access to our products in other formats.Registerwww.cepal.org/en/publicationsfacebook.com/publicacionesdelacepalPublicaciones www.cepal.org/appsProject DocumentsSpeculation and price volatility in the coffee marketJavier Aliaga Lordemann Claudio Mora‐García Nanno MulderThis document was prepared by Javier Aliaga Lordemann, Chief of the Regional Climate Change Program of the Latin American and Caribbean Network of Fair Trade Small Producers and Workers (CLAC); Claudio Mora‐García, Professor at the University of Costa Rica and Senior Researcher at INCAE Business School, in Costa Rica; and Nanno Mulder, Chief of the International Trade Unit of the Division of Internation Trade and Integration of the Economic Commission for Latin America and the Caribbean (ECLAC). It was prepared under the 2020 cooperation agreement between CLAC and ECLAC. The authors are grateful for the comments received from the participants in a workshop held on 3 November 2020 and convey thanks to the International Coffee Organization for facilitating access to its statistics. The views expressed in this document, which has been reproduced without formal editing, are those of the authors and do not necessarily reflect the views of the Organization.United Nations publication LC/TS.2021/59 Distribution: L Copyright © United Nations, 2021 All rights reserved Printed at United Nations, Santiago S.21‐00282 This publication should be cited as: J. Aliaga Lordemann, C. Mora‐García and N. Mulder, “Speculation and price volatility in the coffee market”, Project Documents, (LC/TS.2021/59), Santiago, Economic Commission for Latin America and the Caribbean (ECLAC), 2021. Applications for authorization to reproduce this work in whole or in part should be sent to the Economic Commission for Latin America and the Caribbean (ECLAC), Documents and Publications Division, publicaciones.cepal@un.org. Member States and their governmental institutions may reproduce this work without prior authorization, but are requested to mention the source and to inform ECLAC of such reproduction.ECLACSpeculation and price volatility in the coffee market 3ContentsAbstract ................................................................................................................................................ 5 Introduction ......................................................................................................................................... 7 I. Determinants of price volatility (second moment) .................................................................. 9A. State variables ................................................................................................................... 9 B. Economic variables ............................................................................................................ 9 C. Market structure variables.................................................................................................10 II. Data description and sources .................................................................................................. 13 III. Description of the time series and specifications.................................................................... 17 IV. Methodology ........................................................................................................................... 21 V. Empirical results ...................................................................................................................... 23 VI. Discussion and conclusions ..................................................................................................... 25 Bibliography....................................................................................................................................... 27 Annex ................................................................................................................................................. 31ECLACSpeculation and price volatility in the coffee market 4Tables Table 1 Table 2Table 3Table 4 Table A1Table A2Figures Figure 1 Figure 2 Figure 3 Figure 4Box Box 1Descriptive statistics on the weekly returns on price..................................................19 Results of the baseline generalized autoregressive conditional heteroskedasticity GARCH(1,1) model on US C contract spot and futures prices ..................................... 23 Results of the baseline GARCH(1,1) model of volatility on spot prices by type of position held by speculators......................................................................24 Results of the baseline GARCH(1,1) model of volatility on ICO composite prices .......24 Results of the GARCH(1,1) model of volatility on spot prices, by type of position held by speculators (includes all coefficients) ........................................... 32 Results of the baseline GARCH(1,1) model of volatility on ICO composite prices ....... 33Prices and ope interest in the US coffee C..................................................................14 Shares of open interest by type of commodity trader .................................................. 15 Weekly returns on coffee prices................................................................................. 17 Autocorrelation and partial autocorrelation functions for weekly coffee price returns.................................................................................................... 18Terminology: hedgers, speculators, open interest and volume .................................. 11ECLACSpeculation and price volatility in the coffee market 5AbstractThis study looks into the determinants of green coffee prices’ conditional volatility in three different markets (spot, futures and physical). For this purpose, a generalized autoregressive conditional heteroskedasticity (GARCH) model is used. It includes weekly data on prices and control variables for state, economic, and market structure. Conditional volatility follows a GARCH(1,1) process. Moreover, speculation within futures markets decreases conditional volatility in all three prices.ECLACSpeculation and price volatility in the coffee market 7IntroductionThere is extensive literature on the determinants of spot and futures’ price volatility for several agricultural commodities, excluding coffee. In several developing countries, many families depend financially on green coffee farming. However, significant swings and drops in its prices have increased poverty, considering that most farmers are smallholders (ICO, 2019). Consequently, it is crucial to study what factors affect price volatility. Agricultural commodities have two characteristics that explain the importance of studying volatility in spot and futures prices. On the one hand, its prices display persistent volatility (Vivian and Wohar, 2012; Ghoshray, 2013; Karali and Power, 2013; Dawson, 2015). On the other hand, its supply and demand have a low price elasticity. This means that relatively small production or consumption changes induce rather significant price shifts (Tomek and Kaiser, 2014).In this paper, we study the determinants of price volatility in the coffee market. In particular, we analyze the conditional volatility of three types of prices that reflect different market characteristics. These are a) weekly spot (cash), b) futures prices of the US C Coffee contracts traded at the Intercontinental Exchange (ICE), and c) weekly indicator prices for Arabica coffee on the physical market collected by the International Coffee Organization (ICO). The latter are green coffee quotations in physical markets for prompt shipment. We use a generalized autoregressive conditional heteroskedasticity (GARCH) model to analyze price volatility, assuming a generalized error distribution (GED). We examine the role of different types of variables related to states, the economy, and market structure, including speculation.The effect of speculation on volatility has been studied for many years. Its impact ranges from positive to negative. We measure speculation in several ways and trace its impact on different markets and different supply chain levels. We found that a simple GARCH(1,1) process explains the conditional volatility of the analyzed prices, i.e. a one‐period autoregressive and moving average process. We also discovered that speculation in futures markets reduces conditional price volatility in both the spot and futures markets and physical markets.This document is structured as follows. Section I reports on the main determinants of price volatility pointed out by the literature. Section II describes the data and its sources. Section III analyzesECLACSpeculation and price volatility in the coffee market 8the stationarity of the time series and the necessary transformations to comply with this condition. Section IV describes the methodology used to analyze the data and study the relationship between volatility and its determinants. Section V presents the outcomes. Finally, section VI puts these results in the context of recent developments in the coffee industry and provides conclusions.ECLACSpeculation and price volatility in the coffee market 9I. Determinants of price volatility (second moment)Futures or spot price volatility for agricultural commodities has been attributed to several factors. We mostly focus on the former but also look into the latter towards the end.1 These factors or variables can be classified into different groups: state variables, economic variables and market structure variables. Each is reviewed below.A. State variablesEarly studies on the volatility of futures prices focused on the time‐to‐maturity impact. This is also referred to as the Samuelson (1965) effect, showing that price variance increases approaching a contract’s maturity. Other studies, in contrast, argued that the resolution of uncertainty about underlying supply and demand “state variables” coincides with time passing (Anderson and Danthine, 1983). Moreover, Anderson (1985) and Brorsen (1989) find that seasonality drives the volatility of daily price changes. For example, price volatility in grain markets rises in the Spring, peaks in July or August, and falls towards the end of the year. Milonas (1986) argues that other information that influences volatility is correlated with time‐to‐maturity.B. Economic variablesThe second wave of studies on the volatility of futures prices examined underlying supply and demand factors. These include inventory levels (Thurman, 1988; Williams and Wright, 1991), stocks‐to‐use and prices‐to‐loans ratios (Kenyon et al., 1987), and price levels (Glauber and Heifner, 1986; Kenyon et al., 1987; Streeter and Tomek, 1992). This analysis led several papers to include economic variables as controls. For example, Kenyon et al. (1987) monitored seasonal dummies, price level, production level, a lagged volatility effect, a ratio of the futures price to the loan rate, and a year effect. Glauber and Heifner (1986); Kenyon et al. (1987); Streeter and Tomek (1992), and Goodwin and Schnepf (2000) find1 For references on this topic, see Streeter and Tomek (1992), Goodwin and Schnepf (2000) and da Silveira et al. (2017).ECLACSpeculation and price volatility in the coffee market 10that higher prices tend to be associated with higher levels of price variability. Also, agricultural commodities’ price variability depends on weather conditions (e.g. temperature and rainfall) and other stochastic elements (Hennessy and Wahl, 1996).C. Market structure variablesThe third wave of studies includes market structure variables, such as scalping activity, market concentration, speculation, and trading volumes. Scalping activities should reduce bid‐ask spreads, and thus lower price variability.2 However, Brorsen (1989) argued that scalping might allow prices to adjust to information more quickly and increase price variability. Peck (1981) found that scalping had a positive effect on volatility. Market concentration may also affect price variability (Streeter and Tomek, 1992), reflecting the presence of prominent positions relative to total open interest, regardless of whether hedgers or speculators hold them. Goodwin and Schnepf (2000) pointed out that large traders’ actions may reduce liquidity or cause significant price adjustments and therefore increase price volatility.The evidence on the impact of speculation on price volatility is conflicting. On the one hand, Ward (1974), Peck (1981), Brorsen and Irwin (1987), and Irwin and Yoshimaru (1999) found that speculation reduces volatility. On the other hand, Cornell (1981), Chang, Pinegar and Schachter (1997) and Daigler and Wiley (1999) confirm the opposite.3 Cornell (1981) found a positive relationship between the level of volatility and changes in daily volume. Daigler and Wiley (1999) found that the general public drives the positive volatility‐ volume relationship. The public has imprecise information, is uninformed, and cannot differentiate liquidity demand from fundamental value change. Interestingly, Chang, Pinegar, and Schachter (1997) highlighted a positive effect of large traders on futures daily volatility.4In contrast to previous models that included only one group of variables, Streeter and Tomek (1992) developed a more comprehensive model considering all three groups’ variables. They included state variables (time‐to‐maturity, seasonality, and price levels), economic variables (total annual supply, monthly disappearance, and mill stocks), and market structure variables (scalping, speculation, and market concentration). They found that speculation reduces price variance more than hedging, whereas scalping has the opposite effect.The 2008 financial crisis triggered new literature on the impact of the “financialization” process of the commodity markets on price volatility, showing mixed results (Aulerich, Irwin and Garcia, 2014).5 On the one hand, Bryant, Bessler and Haigh (2006), Haigh, Hranaiova and Overdahl (2007), and Brunetti, Büyükşahin and Harris (2016) confirm that speculators do not influence the volatility of futures prices. Aulerich, Irwin and Garcia (2014) found that commodity index traders (CITs) positions did not cause the massive bubbles in agricultural futures prices. Brunetti, Büyükşahin and Harris (2016) found little evidence that speculators destabilize financial markets and discovered that swap dealer activity is unrelated to contemporaneous volatility. Moreover, Bohl, Javed and Stephan (2013) did not find evidence that conditional price volatility has been affected by expected and unexpected open interest of CITs. Sanders and Irwin (2010) also found little evidence that index funds impact commodity futures returns. On the other hand, Irwin and Holt (2004), Du, Yu and Hayes (2011) and McPhail, Du and2 “Scalping” refers to traders entering and exiting the market often without having open positions at the end of the closing day. Astandard measure of scalping is the ratio of volume to open interest. 3 For a survey on the relation between price changes and trading volume in financial markets in general see Karpoff (1987). 4 Bessembinder and Seguin (1993) distinguish between expected and unexpected aggregate trading volumes, while Yang, Balyeatand Leatham (2005) consider the unexpected overall volume. 5 The financialization process refers to the determination of commodity prices not only by its supply and demand, but also by financialfactors and investors’ behavior in derivative markets (Creti, Joëts and Mignon, 2013).ECLACSpeculation and price volatility in the coffee market 11Muhammad (2012) found a positive relationship. Beckmann and Czudaj (2014) also found evidence in favor of an existing short‐run volatility transmission process in agricultural futures markets.6Box 1 Terminology: hedgers, speculators, open interest and volumeAccording to the CFTC glossary, a hedger is “a trader who enters into positions in a futures market opposite to positions held in the cash market to minimize the risk of financial loss from an adverse price change; or someone who purchases or sells futures as a temporary substitute for a cash transaction that will occur later.” A speculator is “a trader who does not hedge, but who trades with the objective of achieving profits through the successful anticipation of price movements.” A hedger uses futures markets to manage and offset risk, while a speculator assumes market risk for profit.Open interest, also called open contracts or open commitments, is the total number of futures contracts (long or short) held by market participants at the end of the day. This is a flow concept.Volume counts the number of traded contracts. This is a stock concept.Source: the CFTC glossary, see [online] https://www.cftc.gov/LearnAndProtect/AdvisoriesAndArticles/CFTCGlossary/index.htm.More generally, Algieri (2016) argues that speculation is beneficial, increasing liquidity and commercial entities’ ability to transfer the risk of price changes and finance storage. However, excessive speculation may cause price deviations from supply and demand fundamentals. As excessive speculation distorts real market price dynamics, it overcomes the need to satisfy net hedging transactions and market liquidity.Other studies on the relationship between futures markets and the volatility of spot prices found mixed results. Bohl and Stephan (2013) did not find evidence that speculation in futures markets of raw materials increased the volatility of spot prices. However, Algieri (2016) discovered speculation in the futures markets does lead to higher volatility of spot prices.6 According to the Commodity Futures Trading Commission (CFTC), a commodity index fund is “an investment fund that enters into futures or commodity swap positions for the purpose of replicating the return of an index of commodity prices or commodity futures prices.” In addition, a commodity index trader (CIT) can be described as “an entity that conducts futures trades on behalf of a commodity index fund or to hedge commodity index swap positions.” Two well‐diversified and transparent benchmark indicators are the Standard and Poor’s‐Goldman Sachs (S&P‐GSCI) and the Dow Jones‐UBS Commodity Index (DJ‐UBSCI). The activities of CITs are also referred as the equitization or securitization of commodity futures (Bohl and Stephan, 2013).ECLACSpeculation and price volatility in the coffee market 13II. Data description and sourcesThis section explains the sources and features of our time series. We used weekly spot and futures prices on the US ‘C’ Contract for Arabica Coffee traded at the InterContinental Exchange (ICE) market from January 1990 to September 2020. This is a continuous contract for Arabica coffee.7 We complemented this data with weekly averages of ICO indicator prices for Arabica coffee. Three groups make up this indicator price: Brazilian Naturals, Colombian Milds, and Other Milds. ICO indicator prices are a weighted average of daily prices of green coffee on the physical markets in the United States, France, and Germany. Prices are for prompt shipment (within 30 calendar days —instead of immediate shipment) and sales from the origin; quotations come from at least five traders and brokers. Therefore, ICO’s prices are essentially spot prices. ICO indicator prices and the Coffee C Contract traded at ICE are correlated as traders and brokers often trade green coffee as Coffee C Contract including a margin.Weekly data on open interest for each Tuesday is taken from Commitments of Traders (COT) reports issued by the Commodity Futures Trading Commission (CFTC). The COT disaggregate open interest by different market participants and outline whether they are holding long or short positions. The COT splits the number of long and short positions of major futures markets and options into commercial and non‐commercial traders (i.e., hedgers and speculators) and non‐reportables (i.e. small traders). A hedger uses futures markets to manage and offset risk, while a speculator assumes market risk for profit (see Box 1 below). In this respect, only data on futures markets was considered. The sample period started in October, 1992 when the weekly COT report became available.We also use monthly inventory data for Arabica Coffee from the ICE (i.e., historical end‐of‐month certified Coffee C stocks by port held by ICE Futures US Licensed Warehouses measured in total bags). Inventories act as buffers that absorb shocks to demand and supply affecting spot prices (Kim, 2015, pg. 701).7 The market and exchange names of the Coffee C contract changed over time. Until August 2007, it was the Coffee, Sugar and Cocoa Exchange. From September 2007 to December 2019, it was the New York Board of Trade. From January 2020 onwards, it is ICE Futures United States. Futures prices are taken from https: //www.investing.com/commodities/us‐coffee‐c, which are for the contract with the nearest delivery month. Cash/spot prices are from https://www.barchart.com/futures/quotes/KCY00/interactive‐ chart.ECLACSpeculation and price volatility in the coffee market 14We also included macroeconomic indicators to capture the effect of supply and demand shocks. We used quarterly gross domestic product (GDP, Billions of Dollars, Quarterly, Seasonally Adjusted) growth rate and monthly Producer Price Index for All Commodities (Not Seasonally Adjusted), and the Industrial Production Index (Seasonally Adjusted) growth rates. These variables were taken from the Federal Reserve Economic Data (FRED) of the Federal Reserve Bank of St. Louis’ website.Figure 1 shows weekly open interest and prices for the US Coffee C contract. Prices increased from a minimum of US$42.6 on November 25, 2001, to a maximum of US$164.6 on February 24, 2008. Afterward, they dropped again to a minimum of US$108.4 on December 21, 2008. Subsequently, prices increased again, reaching a historical maximum of US$299.35 in April 2011. Other commodities —such as corn, soybeans, sugar, and wheat — showed similar price movements.Figure 1 Prices and ope interest in the US coffee CSource: Elaboration by the authors based on the cited data sources in section 3.For its part, aggregate open interest (OI) in the Coffee C market has persistently increased over the analyzed period. This trend is referred to as “financialization of the commodity market” (Aulerich, Irwin and Garcia, 2014). Between January 2000 and March 2017, OI increased 286% for Arabica. It reached a record high during the financial crisis but plummeted until mid‐2009. Later on, it consistently rose again.Figure 2 displays the shares of open interest by type of trader. These are expressed as ratios of long plus short positions to twice the aggregate open interest. A higher share of short or long positions held by non‐commercial traders reflects increased speculation. From October 1992 to November 2013, the speculators’ share increased from 10% to almost 40%. In the same period, the hedgers’ share fluctuated between 50% and 70%. Small traders lost more than half of their participation. Other agricultural and energy commodities show similar trends.ECLACSpeculation and price volatility in the coffee market 15Figure 2 Shares of open interest by type of commodity trader(Decimals)Source: Elaboration by the authors based on the cited data sources in section 3.ECLACSpeculation and price volatility in the coffee market 17III. Description of the time series and specificationsFigure 1 showed that the time series for prices and open interest are autocorrelated. The price series has no clear linear trend except for periods of large swings or instability followed by a more stable period. This feature is known as a volatility persistence. The open interest series shows a deterministic trend. As a result, we modified prices in levels and calculated price returns —defined as the weekly change on the natural logarithm of the price (
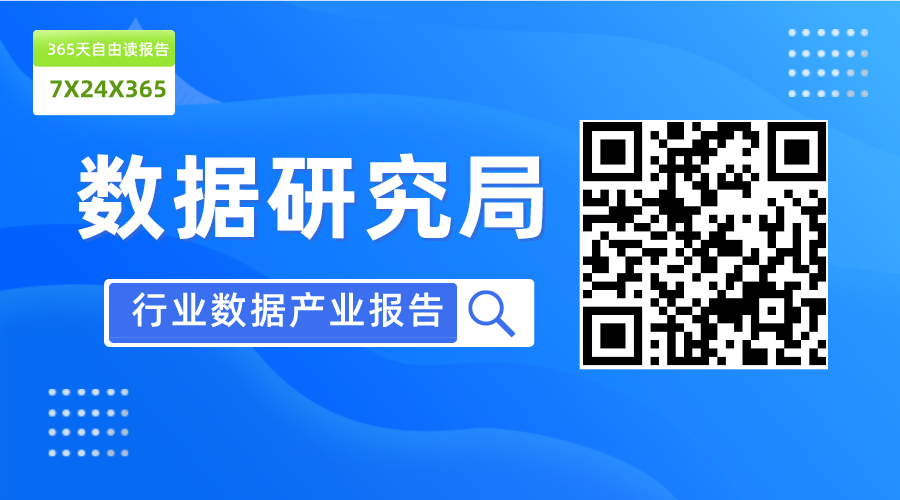
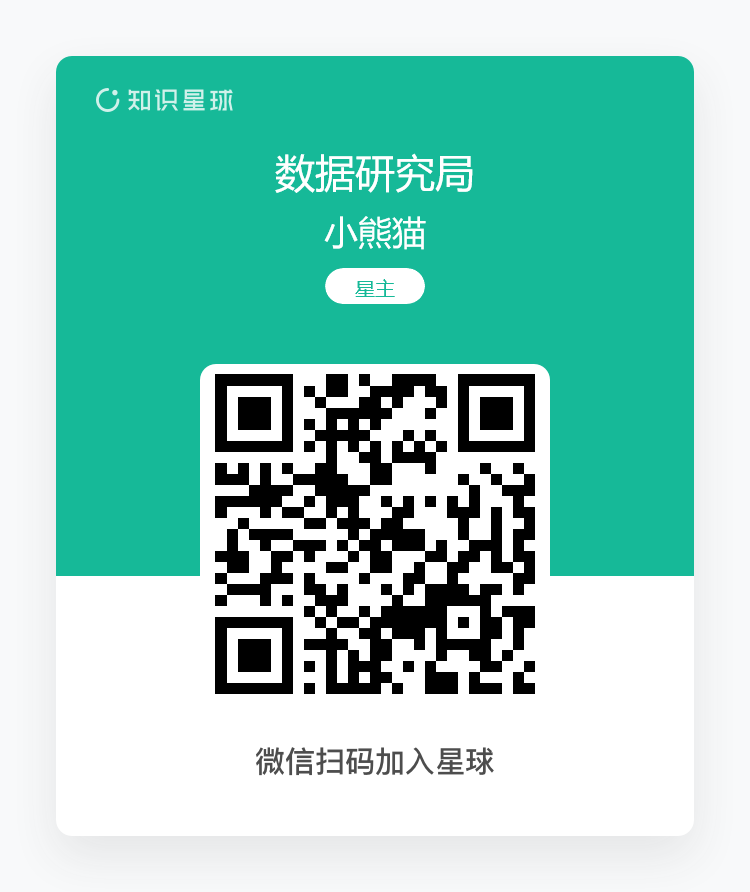